Clouds. Lakes. Warehouses. What do they have in common? Centralized data platforms. While unique, each represents a significant step forward in how organizations gather, analyze and extract value from their data. But they all share a common purpose: to bring vast quantities of data together while converting them into valuable insights without leaving any stone unturned.
These architectures provide the foundation for modern business intelligence and analytics. As a result, there are no limits to the questions you can ask of your data within a single data pool, enabling teams to learn more about their customers, markets and operations.
Well, at least that was the idea.
Time and time again, centralized data platforms have failed to meet the demands of modern businesses. With data volumes booming and demand for business intelligence and analytics surging, some centralized platforms have morphed from a "single source of truth" into a bloated insight bottleneck.
The new challenges of centralized data platforms
Centralized data platforms themselves haven't changed, and in most cases, continue to deliver meaningful business value. But, when it comes to handling today's advanced analytics use cases, they often can't handle the task.
The explosive demand for enterprise data, insights and analytics has put them under significant pressure. Today, that pressure is manifesting itself in three specific ways:
Long cycle times: As centralized platforms swell, the time it takes to import new data has gotten much longer. These long cycle times make it difficult for teams to quickly respond to changing business needs and significantly reduces the value of new data delivery.
High cost of ownership: Many centralized data platforms are built on expensive, proprietary technologies that aren't well-suited for data science and research. In addition, as data volumes grow, so have the costs and workloads associated with maintaining data warehouses and lakes.
Legacy lock-in: Many organizations have created centralized data architectures so complex that moving on from them can feel impossible. The ability to adapt and embrace new data management best practices comes at the expense of getting the greatest possible value.
Challenges like these are keeping businesses from one of the biggest promises of centralized data platforms: the rapid delivery of deep, valuable insights from a single source of truth. So it's time to reconsider the "one ring to rule them all" paradigm.
Enter the Data Mesh.
Introducing Data Mesh
The theory behind centralized data platforms makes sense. But in reality, different business domains call for additional data perspectives, curation techniques and value-creating use cases. The efforts to combine all enterprise data into a single platform for a highly diverse set of value cases becomes increasingly problematic as the diversity of use cases and scale of the platform increases.
One particular problem is the low speed of new value creation. Integrating new data sources can take months and may not even fit the individual value cases of various domain experts. On top of that, most data consumers don't even require access to all enterprise data. Instead, they need a meaningful, trustworthy subset of cross-cutting data that contains what they want — nothing more, nothing less. Or easier said, tailor-made data packaged as a product.
Data aligns itself with functional enterprise domains such as sales, finance or inventory management and becomes a product in a data mesh. Each available domain takes responsibility for these data products and the evolving needs of the end-users, while an overarching governance policy maintains interoperability between them. This decentralized approach to data management shares ownership between functional teams and all have the freedom to explore new ways of putting data to its best use.
From a business point of view, the Data Mesh puts the people most likely to get value from data sets in control of them. The result? Bottlenecks break down. Time to insight is accelerated. And everyone can help themselves to relevant data products while managing and expanding their custom data sets. It's easier and faster to add new sources to a data mesh because it doesn't require modifying existing data schemas or processing pipelines. Instead, new data products are introduced into the mesh by consuming data from other data products and combining or enhancing that data for other consumers.
So, what does Data Mesh look like in action? We introduced Data Mesh as an alternative for a healthcare company that relied on an enterprise data warehouse. When the COVID-19 pandemic struck, the organization responded in just thirteen days, deploying new data products into their Data Mesh while developing a set of care applications for their members. Having near real-time data available allowed teams to produce insights, train models and predict trends to optimize their approach. It wouldn't have been possible for the former enterprise data warehouse to do the same.
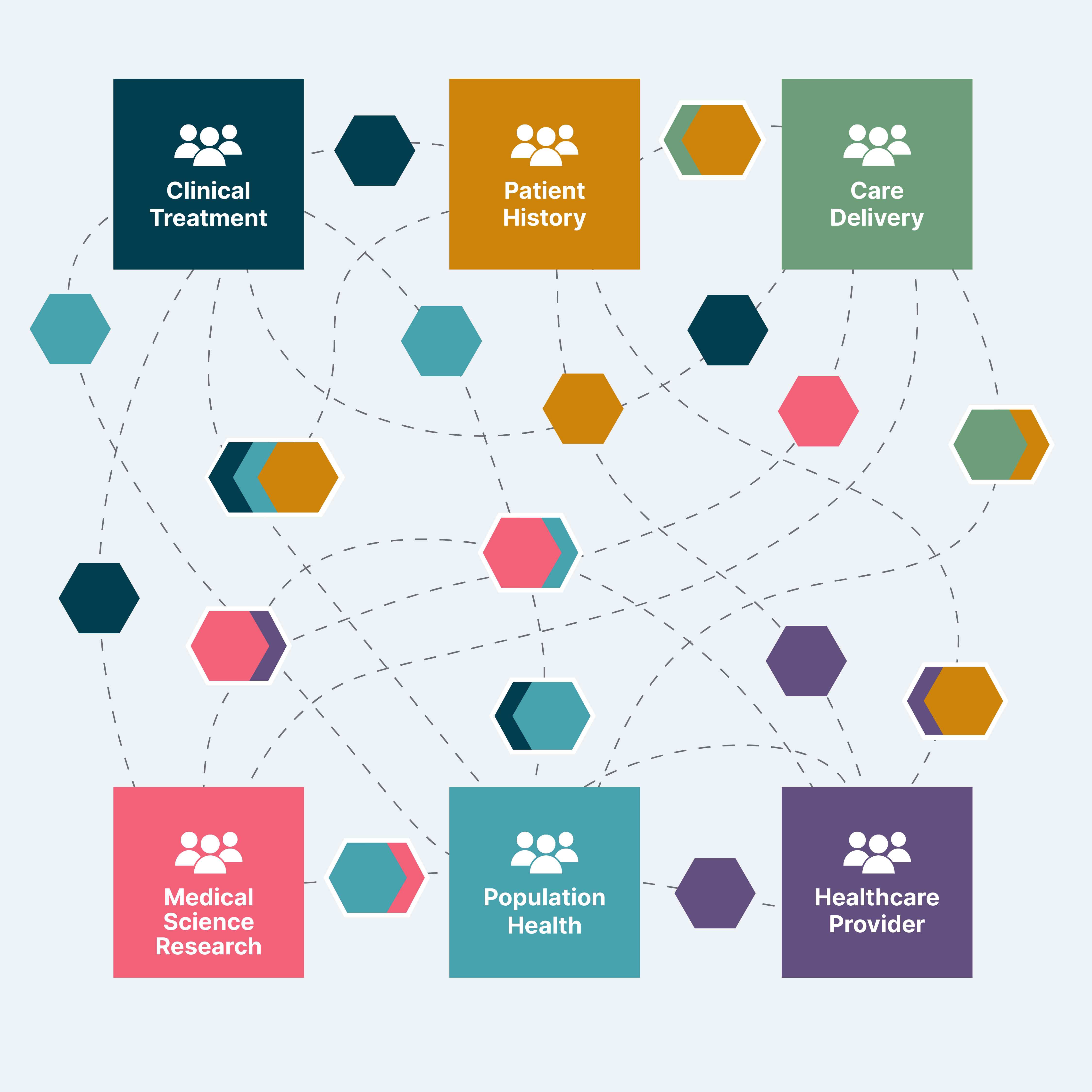
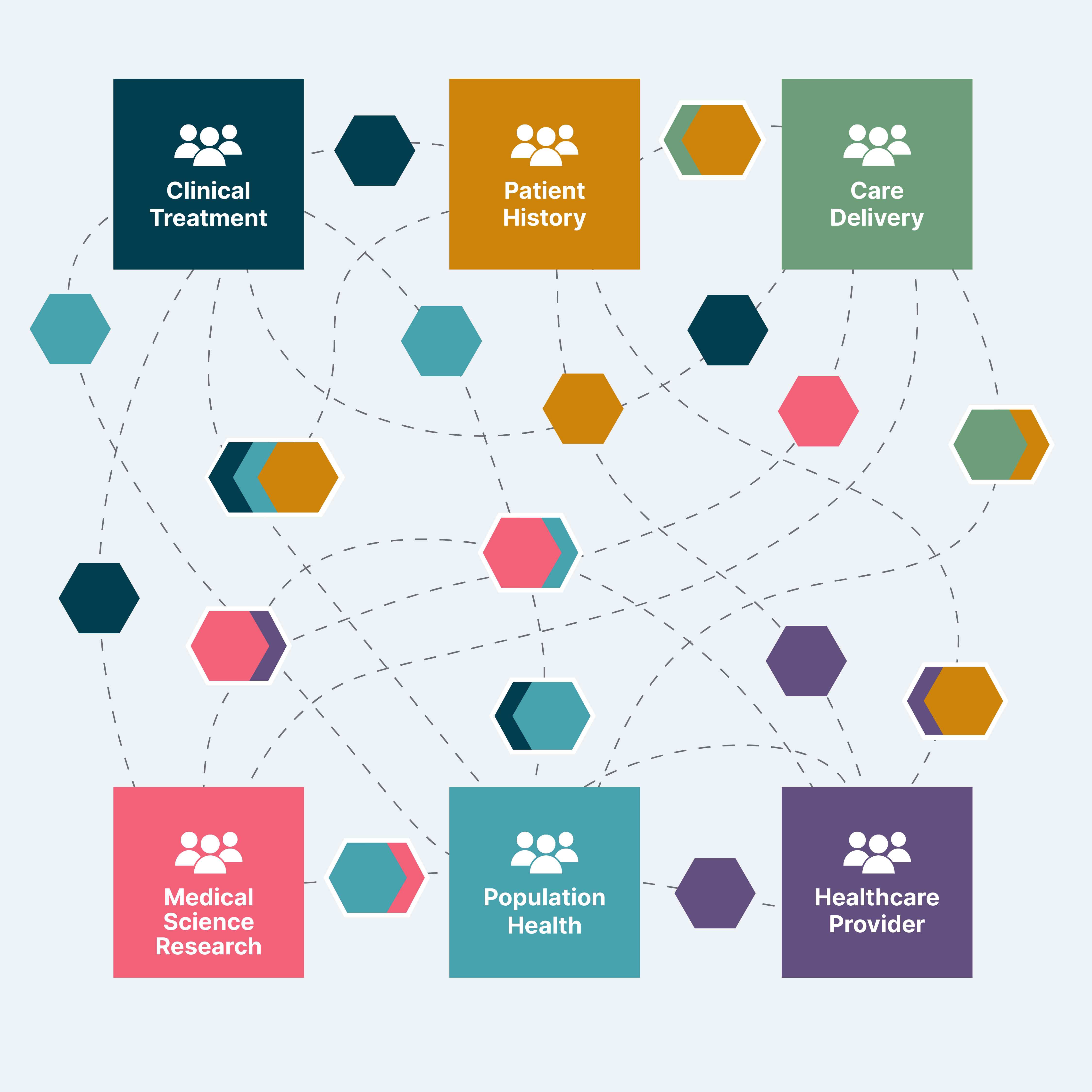
Bringing product and platform thinking to data management
A Data Mesh combines several emerging technology best practices to create a new paradigm of data management — one designed to meet the demands of modern organizations and teams.
Firstly, Data Mesh is built upon the principles of self-service platform design, allowing teams to help themselves to data products. The creation of new data products helps reduce the vast amount of management work required when relying on centralized data platforms. Instead of going to the core IT group, everyone is empowered to serve themselves.
Data Mesh also uses the principles of Product Thinking. Teams can see the data sets they curate as products while recognizing the organization's groups, analytical applications and data scientists as the customers. Then, those products are managed with the same rigor as external customer-facing products, ensuring they always meet the needs of the consumers that depend on their insights.
Together, platform design and product thinking help make decentralized, democratized data management a reality — resolving many of the quality and management concerns organizations naturally have with this new approach.
Enabling a continuous cycle of intelligence
Data Mesh is great at unlocking data, empowering functional teams and eliminating the inertia created by centralized data platforms. But it's only the start of the operational value they can deliver when properly managed and implemented.
Data Mesh represents a leap towards an innovation business and technology leaders have been trying to engineer for decades: a continuous cycle of intelligence.
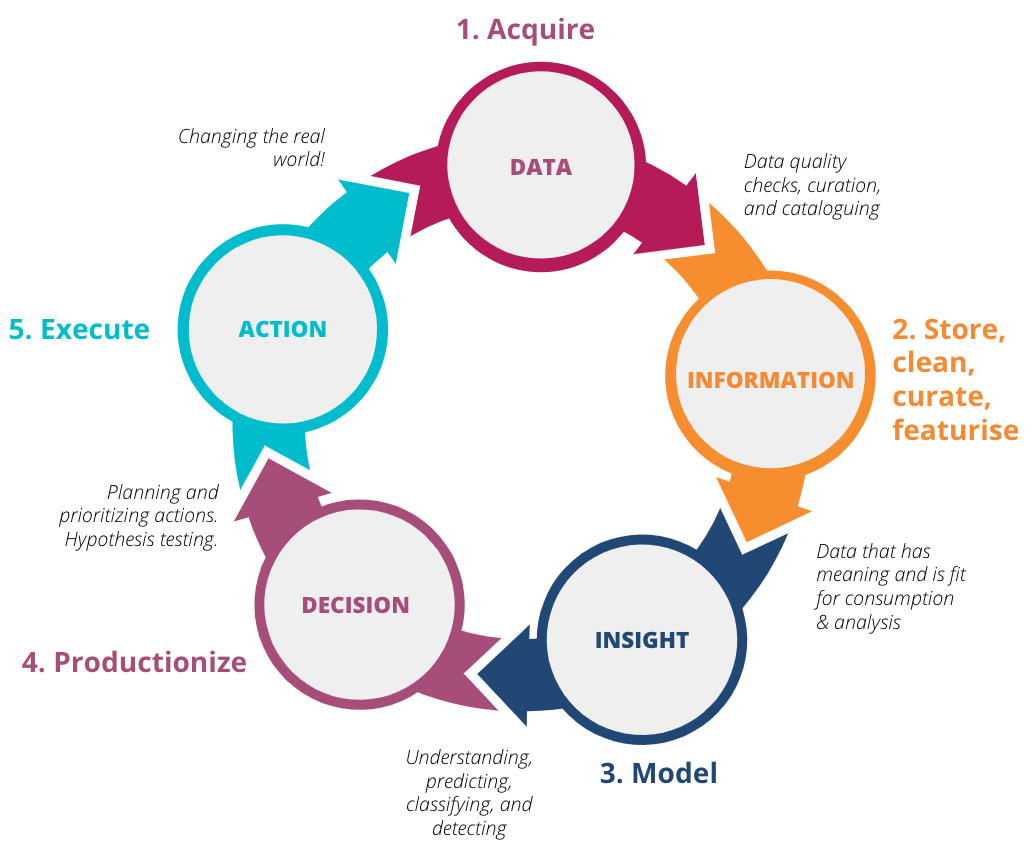
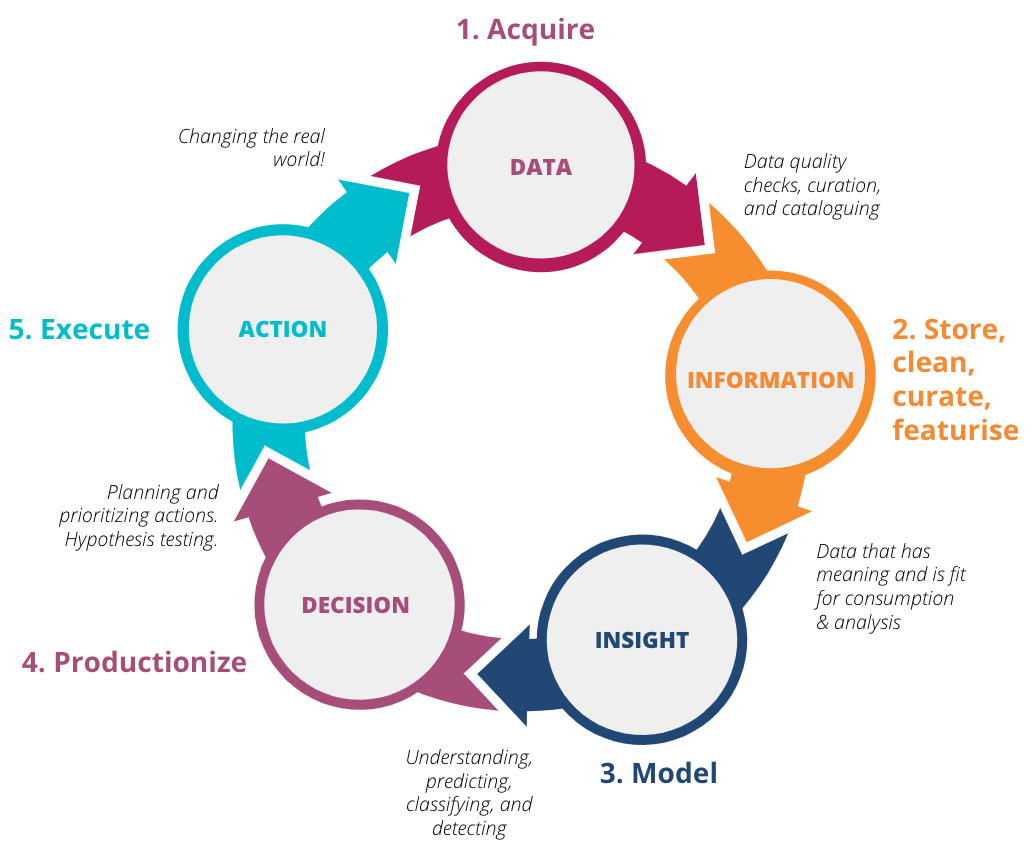
The Cycle of Intelligence above visualizes how data converts from intelligence and insight into informed business decisions. The results create new data and ultimately renew the cycle. It may seem simple, but looks can be deceiving. Achieving continuous intelligence remains elusive for most organizations, mainly because they struggle with unlocking their data, quickly acting on insights or accurately capturing feedback.
Data Mesh can help solve all three challenges at once:
Bespoke, user-created data products help unlock valuable data at speed.
Democratized access to the products accelerates insight generation and puts them into the hands of people who can take action.
Data products can receive updates by the teams directly seeing and measuring the impacts of any changes made.
That's the power of bringing data closer to the people that need it most and know it best. And at its heart, that's what a Data Mesh both supports and delivers.
Is a Data Mesh right for you?
There is no "one size fits all" solution when it comes to data management. But, if you'd like to learn more about Data Mesh and how we can enable it for your organization, get in touch with us today.