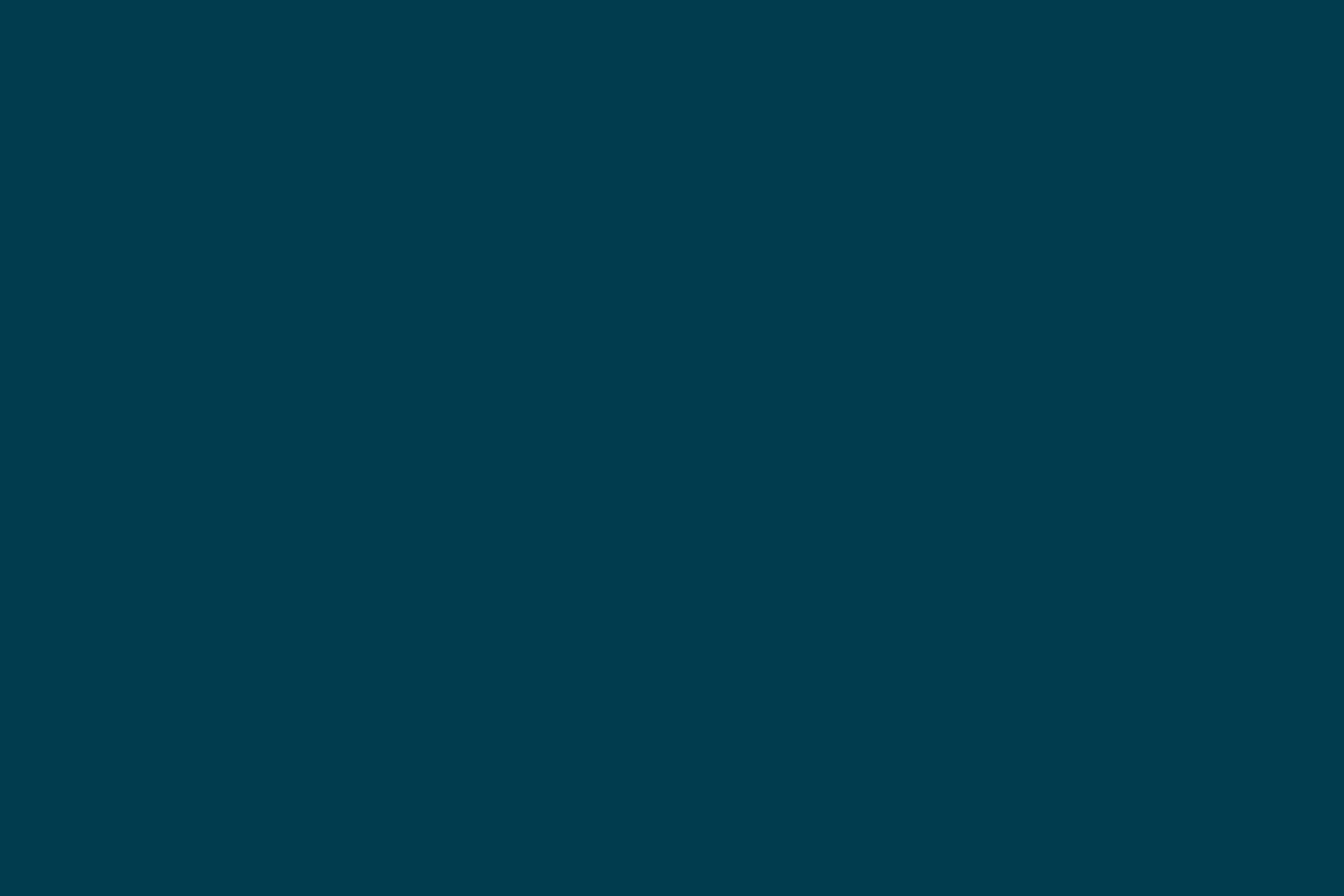
7 Reasons Big Data Analytics Initiatives Fail
By
Published: February 12, 2017
Every business worth its multi-million dollar tagline wants to understand and leverage big data analytics. As the former try to understand big data in all its beauty—and derive true and timely business value from it, sometimes the well intended initiatives do fail.
I have picked out seven reasons for such failures and described them in the form of scenarios. Also, for better context, I have placed these scenarios under three separate themes; Organizational Quagmire, Old Habits Die Hard and New Approaches Create New Problems.
Organizational Quagmire
Never-ending queue of unabated requests
A lead technologist becomes the Chief Data Officer (CDO) of an investment bank. These are some of the first requests to reach her table:

Functional silos
A retail company's IT team is horizontally structured with sub-teams for program management, business analysis, user experience, deployment and database management. Each team has a lead and subculture. Awareness and interest in big data leads to adding three more sub-teams: data science, data engineering and data visualization.
Along comes a high-profile big data project for which a Center of Excellence (CoE) representing each of the IT sub-teams is assembled. However, the CoE’s members do not get out of their functional siloes, delivering a suboptimal product.
How could this have been avoided?

Chasm between business and IT
A retail organization’s IT arm sponsors a data analytics group that quickly gains free reign. Business views it as an ‘IT project’ and does not intervene. Six months goes into building sophisticated models for demand forecasting and inventory management.
During a demo, business users ask, “Is the new model really necessary? The old model does what we want, at least for now.” But given the investment already made, the IT lead productionizes the proposed model. A year later at launch, business users refuse the product.
How could this have been avoided?

Careless positioning statements
The aforementioned retail company was probably dealing with ill informed assumptions.
Quite often, big data analytics tools are expected to provide insights on trading recommendations, process, asset recommendations, and more. This crosses paths with what senior business analysts and functional experts work on.
Doing away with the misrepresentation:

Old Habits Die Hard
Huge upfront investment
A product company’s Chief Technology Officer (CTO) buys commercial technology with 50% of his hardware budget. In a year, the company onboards ten new customers and data volume increases by a hundredfold. However, the product company’s infrastructure supports only 10% of their use cases and the CTO is caught between a rock and a hard place.
What should the CTO have kept in mind:

New Approaches Create New Problems
Using the wrong tools for the job
A heavy equipment manufacturer wants to use predictive analytics for effective lead generation. The manufacturer wants to aggregate on-the-ground-wear-and-tear telematics data, along with machinery specifications and customer service schedules. The information will predict service visits and recommend preventive interventions for customers.
The team uses brute force to integrate the accumulated data into the legacy data warehousing and business intelligence infrastructure. The job takes several days to run, and is obviously not scalable.
More than just a cosmetic change because:

Building organizational trust in data products and algorithms
A major oil company wants big data analytics to provide insights into and optimize their oil pipeline and storage assets. One of their quantitative experts develop an ingenious algorithm that pulls in all pipeline and storage capacity, consumption details, and demand and supply scenarios. Using this information, the algorithm presents recommendations.
But the leads do not trust the algorithm. The data team needs to build trust using detailed and creative data visualizations. This requires pre-planning and proper instrumentation of code and pipeline to record the right intermediate snapshots.
Building trust is important because:

Big data analytics is a high impact area, but there are risks that can be smartly mitigated as businesses embark on their big data journeys. It all starts with answering a few pertinent questions and visualizing a few impending scenarios to get the best out of high-stake big data analytics initiatives.
This article was featured in Firstpost.
I have picked out seven reasons for such failures and described them in the form of scenarios. Also, for better context, I have placed these scenarios under three separate themes; Organizational Quagmire, Old Habits Die Hard and New Approaches Create New Problems.
Organizational Quagmire
Never-ending queue of unabated requests
A lead technologist becomes the Chief Data Officer (CDO) of an investment bank. These are some of the first requests to reach her table:
- The Chief Risk Officer (CRO) wants big data to address regulatory and risk management gaps
- The Chief Marketing Officer (CMO) wants deeper customer understanding to inform marketing spends and drive revenue through personalization
- The Chief Operations Officer (COO) wants to benchmark operations against competition and build dashboards to optimize and improve efficiencies
- Trading Managers want solutions around portfolio optimization, automated research and similar
- The Chief Information Officer (CIO) wants to reduce costs by leveraging artificial intelligence for production monitoring and technology operations
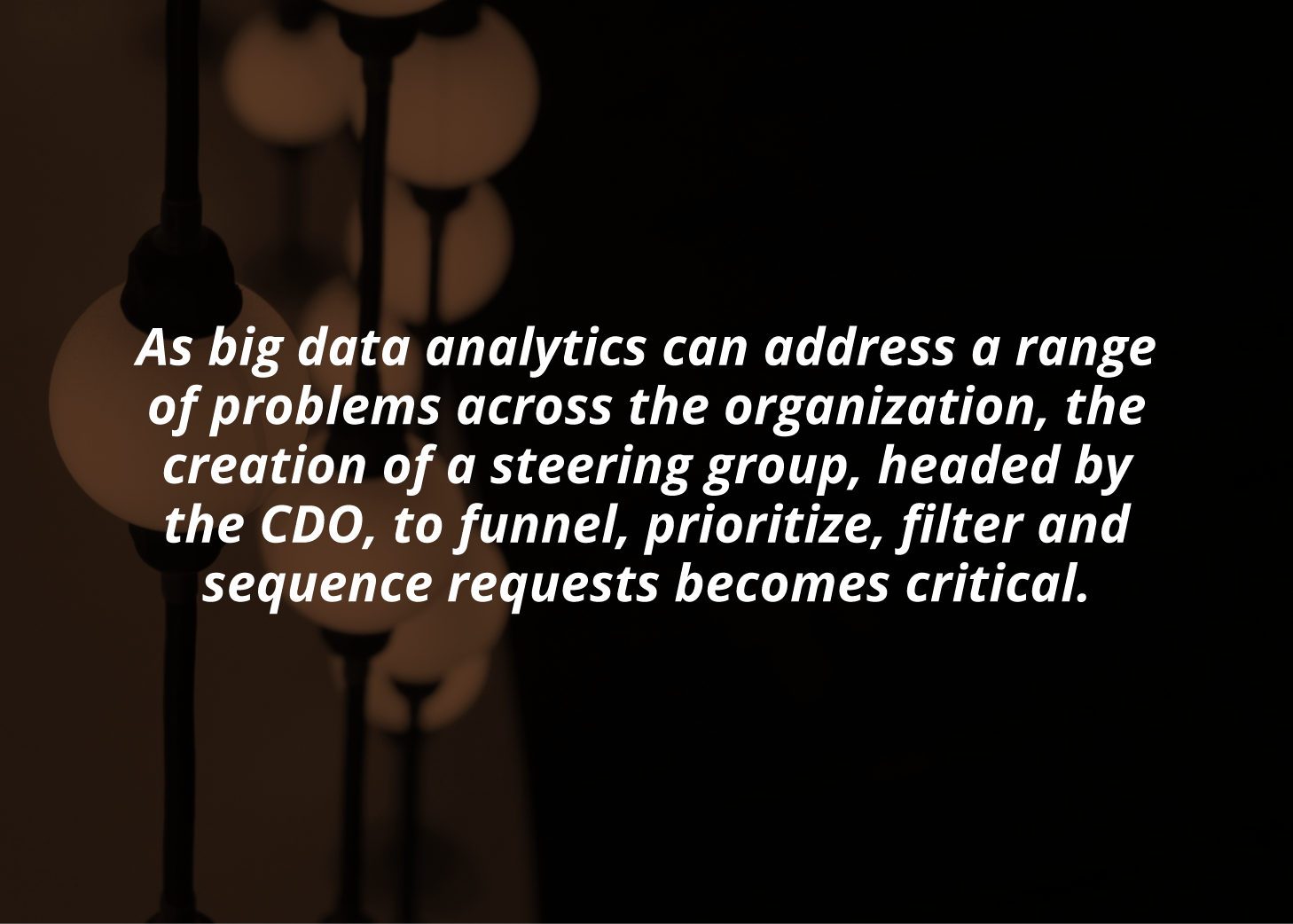
Functional silos
A retail company's IT team is horizontally structured with sub-teams for program management, business analysis, user experience, deployment and database management. Each team has a lead and subculture. Awareness and interest in big data leads to adding three more sub-teams: data science, data engineering and data visualization.
Along comes a high-profile big data project for which a Center of Excellence (CoE) representing each of the IT sub-teams is assembled. However, the CoE’s members do not get out of their functional siloes, delivering a suboptimal product.
How could this have been avoided?
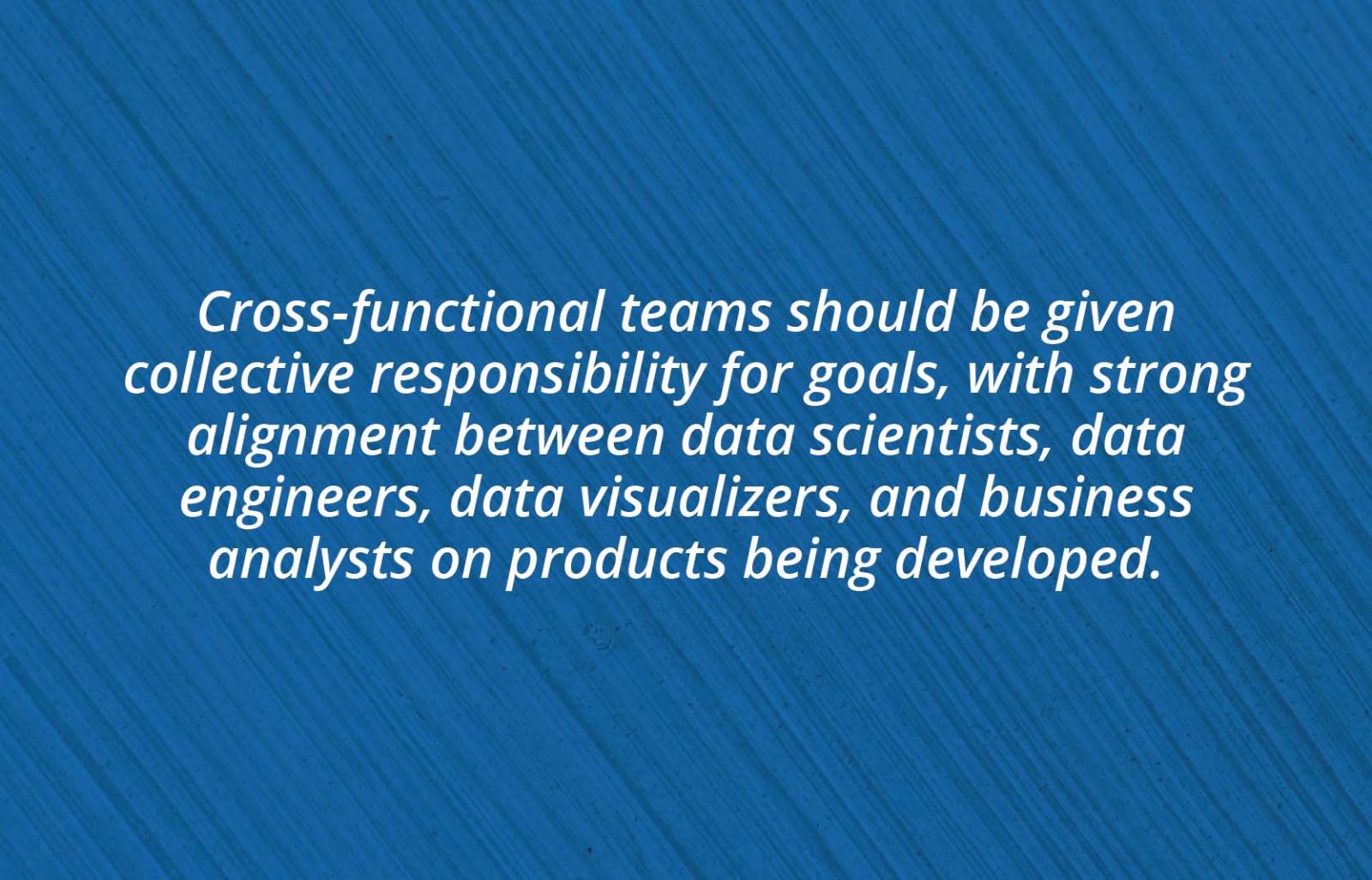
Chasm between business and IT
A retail organization’s IT arm sponsors a data analytics group that quickly gains free reign. Business views it as an ‘IT project’ and does not intervene. Six months goes into building sophisticated models for demand forecasting and inventory management.
During a demo, business users ask, “Is the new model really necessary? The old model does what we want, at least for now.” But given the investment already made, the IT lead productionizes the proposed model. A year later at launch, business users refuse the product.
How could this have been avoided?
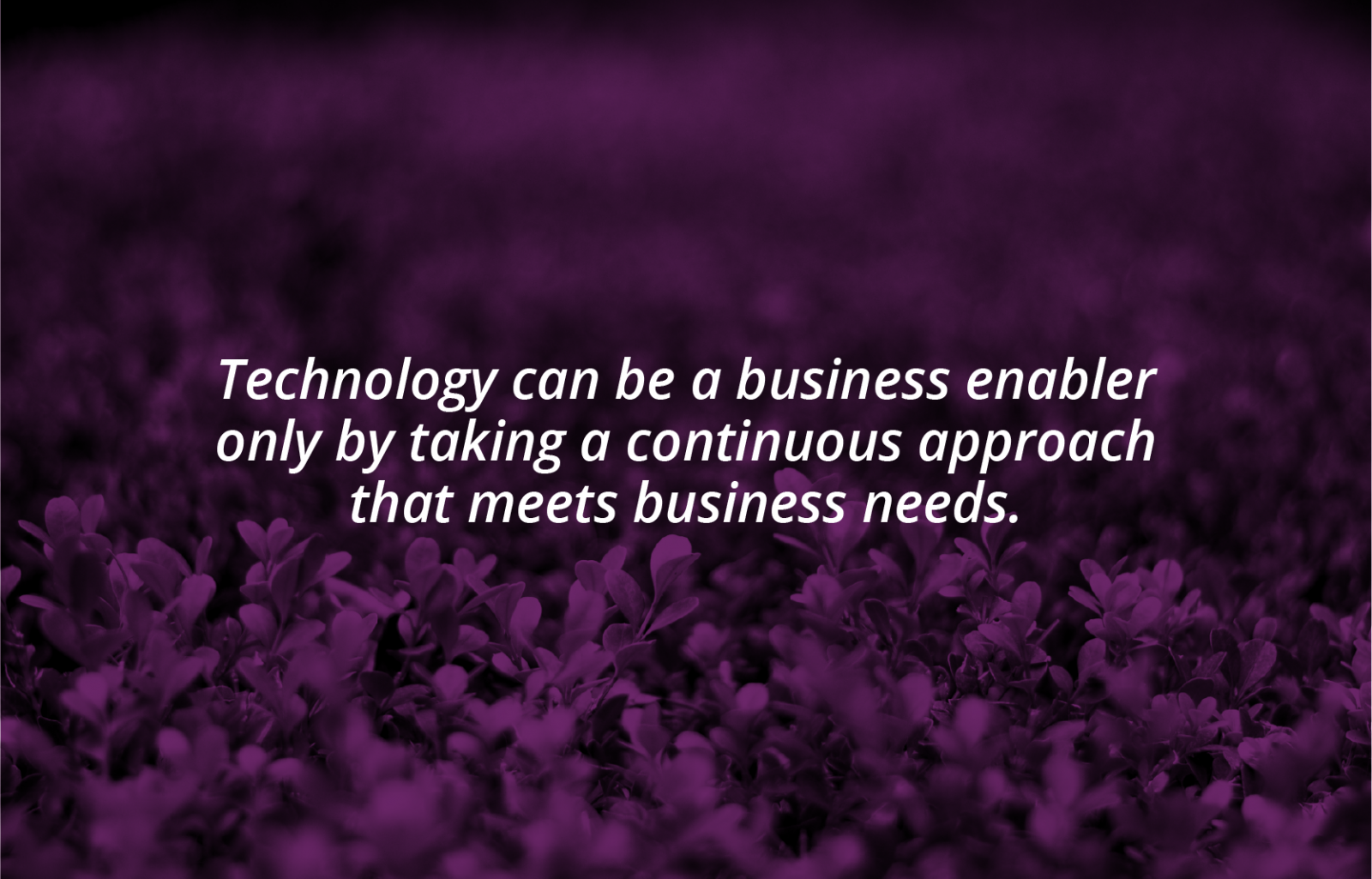
Careless positioning statements
The aforementioned retail company was probably dealing with ill informed assumptions.
Quite often, big data analytics tools are expected to provide insights on trading recommendations, process, asset recommendations, and more. This crosses paths with what senior business analysts and functional experts work on.
Doing away with the misrepresentation:
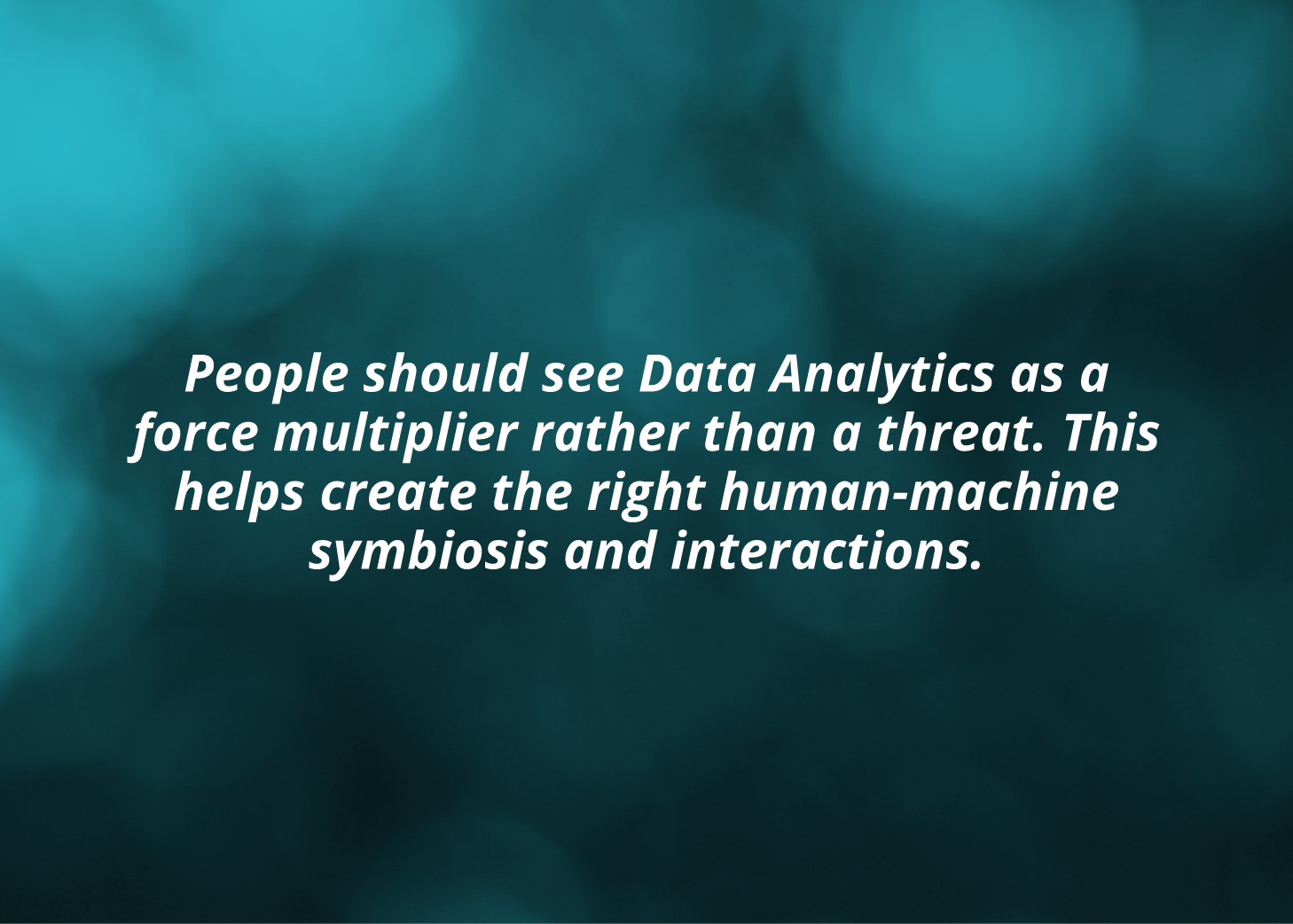
Old Habits Die Hard
Huge upfront investment
A product company’s Chief Technology Officer (CTO) buys commercial technology with 50% of his hardware budget. In a year, the company onboards ten new customers and data volume increases by a hundredfold. However, the product company’s infrastructure supports only 10% of their use cases and the CTO is caught between a rock and a hard place.
What should the CTO have kept in mind:
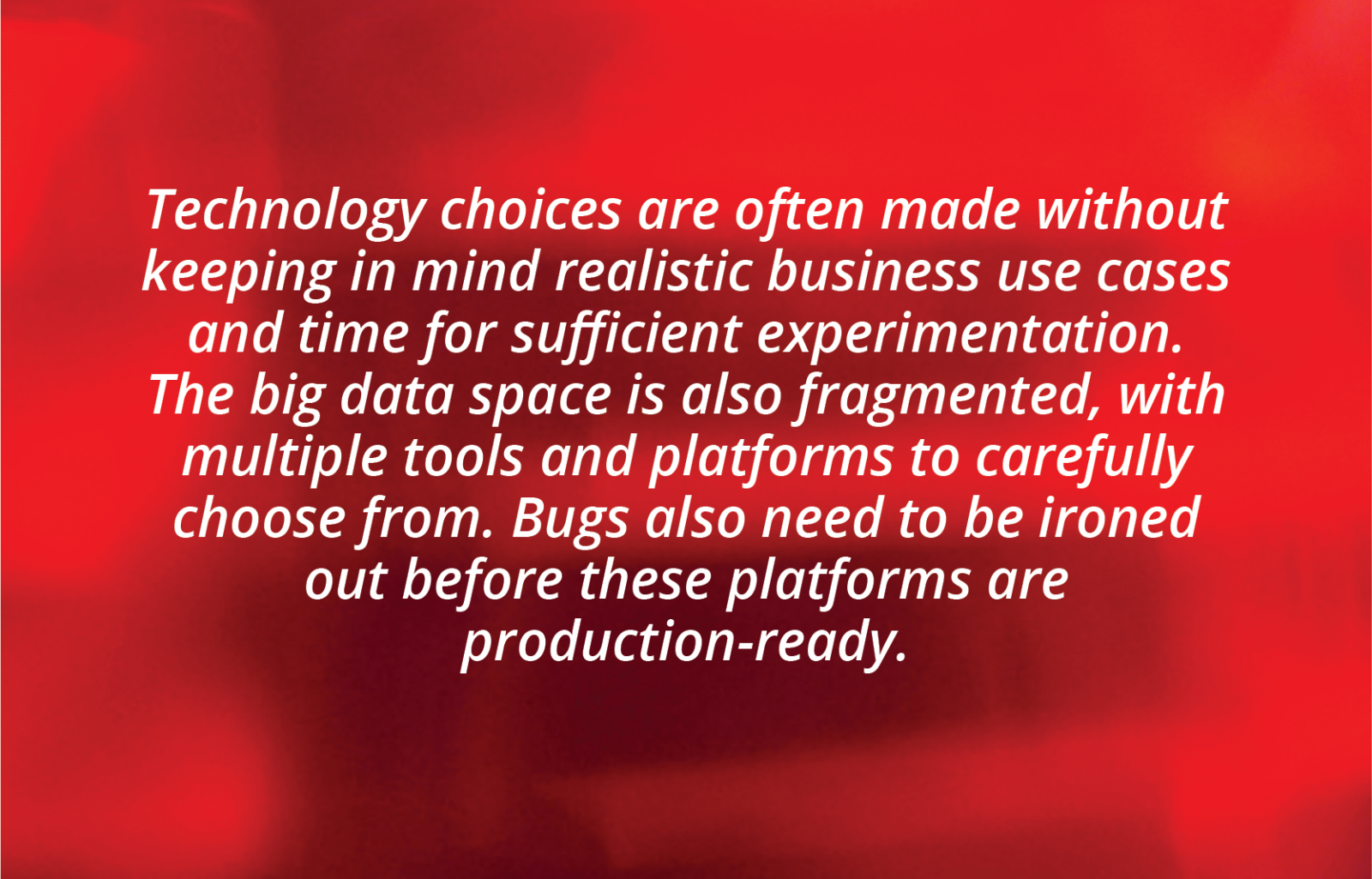
New Approaches Create New Problems
Using the wrong tools for the job
A heavy equipment manufacturer wants to use predictive analytics for effective lead generation. The manufacturer wants to aggregate on-the-ground-wear-and-tear telematics data, along with machinery specifications and customer service schedules. The information will predict service visits and recommend preventive interventions for customers.
The team uses brute force to integrate the accumulated data into the legacy data warehousing and business intelligence infrastructure. The job takes several days to run, and is obviously not scalable.
More than just a cosmetic change because:
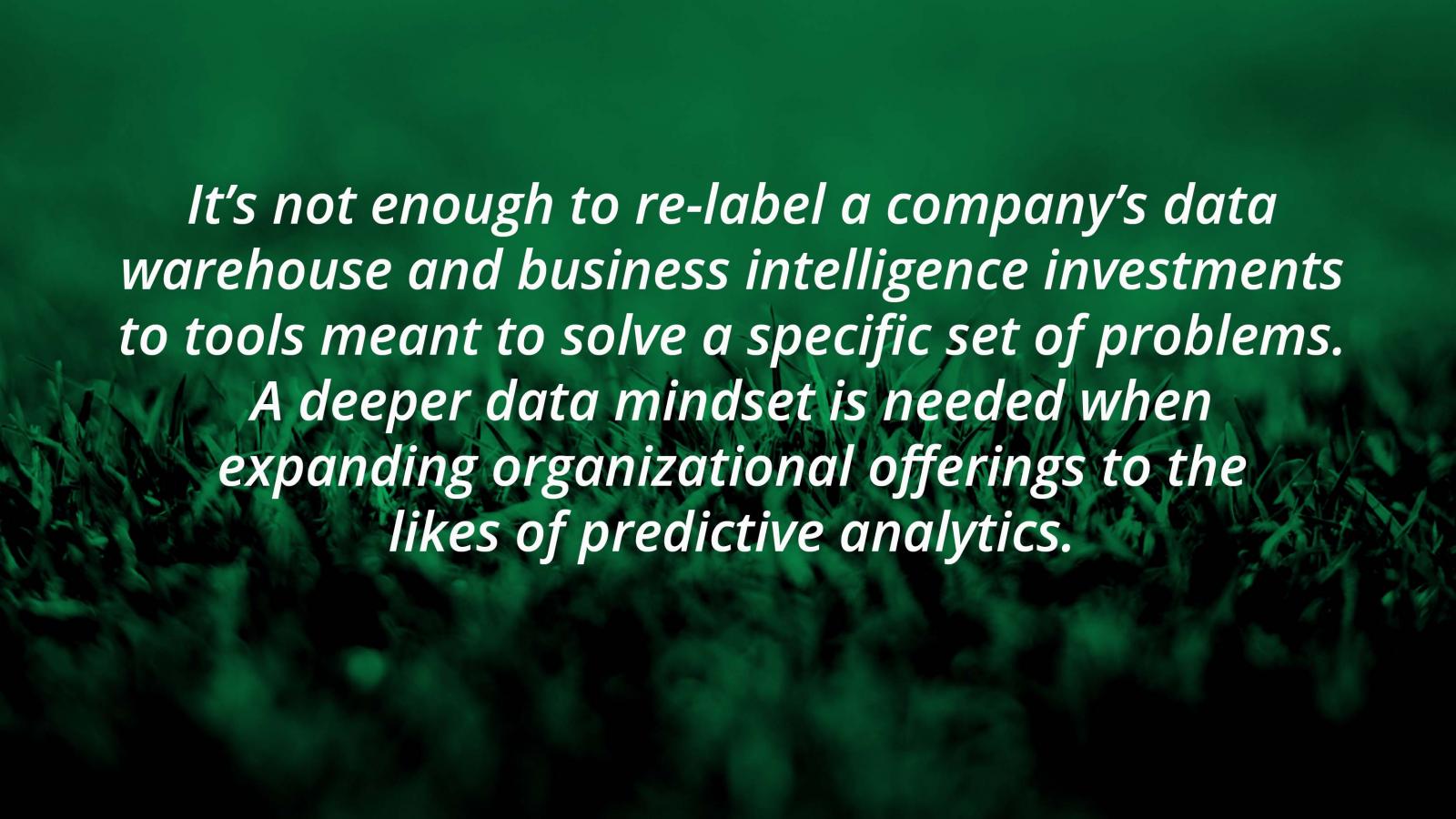
Building organizational trust in data products and algorithms
A major oil company wants big data analytics to provide insights into and optimize their oil pipeline and storage assets. One of their quantitative experts develop an ingenious algorithm that pulls in all pipeline and storage capacity, consumption details, and demand and supply scenarios. Using this information, the algorithm presents recommendations.
But the leads do not trust the algorithm. The data team needs to build trust using detailed and creative data visualizations. This requires pre-planning and proper instrumentation of code and pipeline to record the right intermediate snapshots.
Building trust is important because:
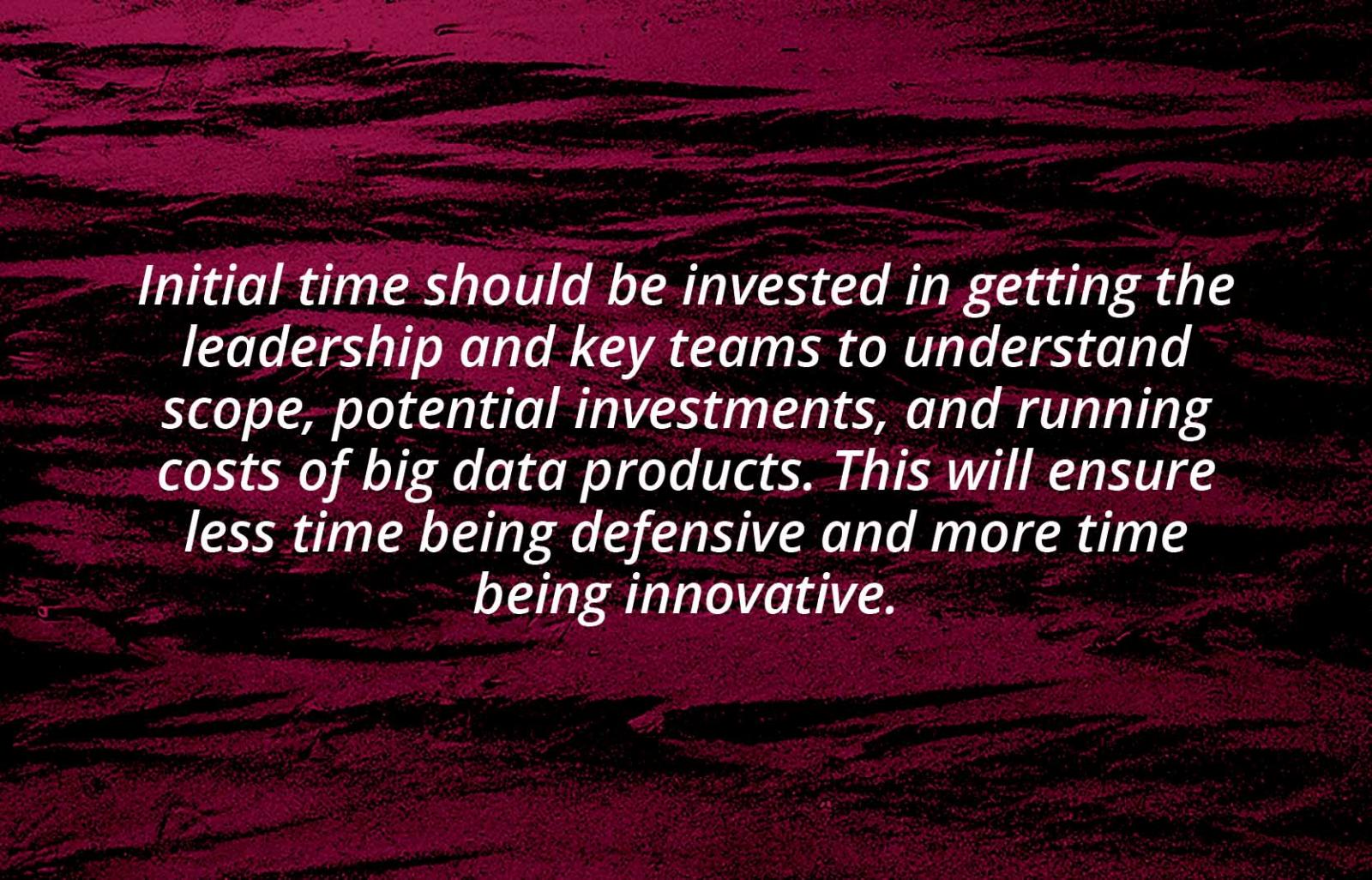
Big data analytics is a high impact area, but there are risks that can be smartly mitigated as businesses embark on their big data journeys. It all starts with answering a few pertinent questions and visualizing a few impending scenarios to get the best out of high-stake big data analytics initiatives.
This article was featured in Firstpost.
Disclaimer: The statements and opinions expressed in this article are those of the author(s) and do not necessarily reflect the positions of Thoughtworks.