AI is transforming and disrupting virtually every aspect of the modern business. From streamlining operations and transforming customer experiences, to upending business models and augmenting human expertise across every function, its reach and impact have been unparalleled.
But we’ve yet to see the full impact AI could have on modern data architectures and paradigms like data mesh. Several big questions remain: Are the two a good fit for each other? What are the risks and potential opportunities of combining them? And are we just trying to force two powerful and emerging technology areas together?
To get some answers to those questions and more, Thoughtworks hosted an in-person AI for data mesh panel with tech and business services leaders from mobile.de, Kleinenzeigen and Parloa. Here’s a quick look at some of the highlights from the group’s conversation:
Panel recording
The AI and data mesh opportunity
At the beginning of the session, the panel immediately addressed what is likely the most pressing and important question about the relationship between AI and data mesh: Are we simply trying to force two buzzwords together?
Thoughtworks Principal Data and AI Strategist Amy Raygada laid those concerns to rest. “It’s not forcing, because the two are very different,” she explained. “If you use AI to automate something like data quality pipelines or security checks within a data mesh, that’s natural. You're just applying extra technology on top of the data mesh infrastructure that you have.”
The panel then turned their attention toward some of the clearest opportunity areas for AI within a data mesh. The first opportunity identified was enhancing data products. “Data products are a big part of data mesh and can definitely be enhanced by AI,” said Csaba Tamas, VP Product from Parloa. “There's a lot of dark data around, which companies have access to physically, but they cannot make sense of it. Now, GenAI makes that easier. Teams can easily infer meaning from data at scale and easily label and tag it. This leads to data product quality that previously wasn’t achievable.”
Addressing tensions and challenges between AI and data mesh
Fundamentally, AI and data mesh are very different concepts. “AI applies the power of machines to get the most out of data, whereas data mesh is all about putting people at the center of the picture,” remarked Romain Thibault, Data Transformation Manager from mobile.de.
“Data mesh drives us to prioritize adoption matters, our data culture, user feedback and user experiences,” he continued. “That’s why AI ethics and explainability are so important. We cannot have black box machines making decisions in these environments that are built to put people at the core.”
Data mesh brings huge governance advantages. By their nature, domain-owned and governed data products are highly discoverable and sharable, which can help organizations work closely with regulators and maintain continuous compliance. If black box AI tools are added to the mix, that could send things in the wrong direction — making data and processes less explainable and creating compliance issues.
However, this interesting tension between AI and data mesh could be exactly what makes them complement one another well. AI augments human expertise by handling huge and complex processing, cleansing and analysis tasks, and making data products exceptionally strong. Then, data mesh augments AI by providing vital human verification, ownership and governance to ensure AI ultimately has the right impact on data products, the organization and on society at large.
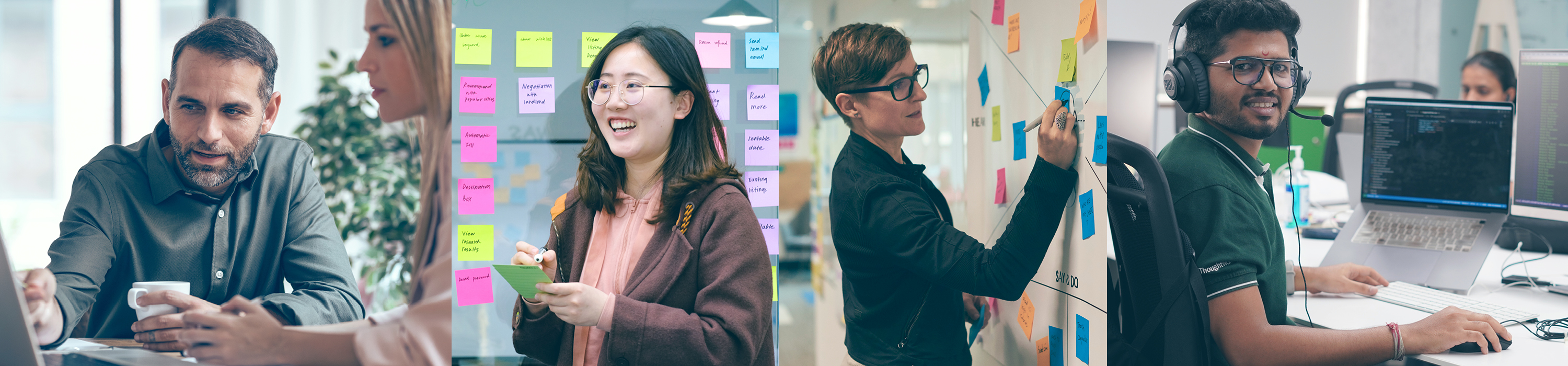
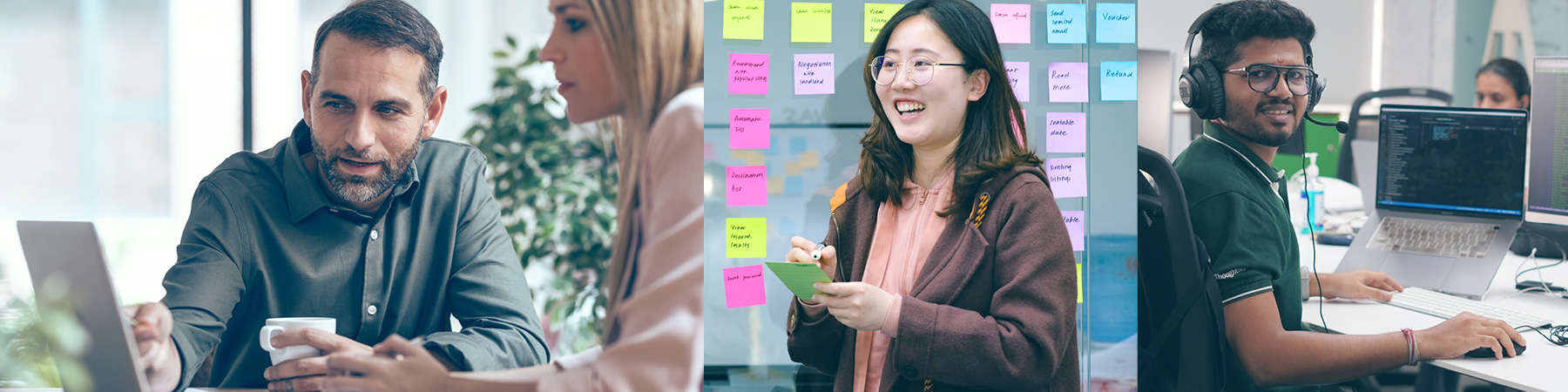
If you’re going to apply AI within a data mesh, you need to keep a close eye on consumption
The other major challenges discussed by the panel were the costs and sustainability implications of applying AI within data mesh environments.
“When you empower people with AI tools, there’s a perception that they’ll just turn up and make as many queries as they need and get immediate answers,” explained Amy. “They don't have a notion of how much this is going to cost and it’s up to us, as data people, to understand our tools’ impacts on budgets and make budget-friendly solution and model decisions.”
Sustainability and consumption go hand in hand. “Today, the biggest things keeping customers up at night are sustainability and climate change,” remarked Csaba. “If your organization has high ESG goals and your customers have high expectations, it may not be right to apply AI in certain areas like data mesh.”
Three steps for successfully augmenting a data mesh with AI
Overall, the panel concluded that AI has the potential to augment data meshes in powerful ways that could significantly boost the quality and value delivered by data products. However, it must be carefully implemented.
Here are three pieces of advice shared by the group to help ensure that AI is applied strategically within data mesh environments — and that it ultimately supports what the data mesh was first designed to achieve:
- Conduct AI readiness assessments: Readiness assessments can help determine whether AI is a good fit for you based on the needs of your organization, customers and the specifics of your current architecture and data environment.
- Keep the principles of data mesh in mind: As the panel pointed out, one of the key advantages about data mesh architectures is that they put people at the core and in control of their data. AI must be applied in ways that augment human capabilities and humans must always stay in the loop to validate AI outputs — otherwise, some of the key benefits of data mesh could be undermined.
- Prioritize security, safety and compliance: If you’re going to layer AI capabilities on top of your data mesh, make sure they’re explainable, secure, open and able to securely generate ethical outputs at scale. Data mesh is an open paradigm which supports governance and compliance, so ensure any AI you implement also supports those goals.
Don’t forget to watch the recording of the panel discussion to learn more about how AI could help augment data mesh and drive the paradigm’s next evolution.