With all the hype around AI currently, it’s easy to be overwhelmed by the amount of content proclaiming its benefits and warning against its risks. Amid this information overload, business leaders find themselves navigating a high-stakes landscape, compelled to swiftly harness AI's potential to carve out a competitive edge for their organizations.
It’s common to see the delivery of small-scale Proof of Concepts (PoCs) as leaders look for AI use cases that will deliver business value. But when it comes to integrating and operationalizing the technology, most organizations struggle to get started. Often, it’s a simple case of not having the right skilled resources to operationalize AI at the required scale. In large, complex organizations with siloed data, it’s challenging to share data across business functions, preventing AI tools from learning from a single, accurate data source. Plus, customers are implementing niche AI solutions to solve specific problems, but these are difficult to connect to other systems and scale to an enterprise-wide level.
Faced with this complexity and effort it’s understandable that many AI tools never make it out of the PoC stage. But there’s one area where operationalizing AI can be simpler and deliver significant business value: managed services.
The managed services AI opportunity
In managed services, enterprise data is already consolidated, with well-defined relationships and structure in a secure, contained environment. It’s an area specifically designed to enable enterprise-wide application and infrastructure management, significantly mitigating the challenges and risks associated with deploying AI in complex organizational settings. In this controlled proving ground, organizations can demystify AI, learn lessons and provide a clear example of the business impact that can be expanded to other AI use cases – uncovering new revenue opportunities, creating personalized customer experiences, or solving seemingly intractable business issues.
What’s more, the deployment of self-learning tools in managed services bridges the skills gap for Level 1 support (meaning you can hire non-tech people for IT support), as the AI itself uses existing data to identify and define potential problems, solve recurring challenges and seek out new efficiencies and cost savings. In fact, the self-learning model can become a customer self-service AI platform, resulting in a reduced number of calls made to the service desk, and productivity gains resulting in up to 50% cost reduction.
However, the real business value in embedding AI into managed services comes from harnessing the insights AI tools generate and feeding them into your digital products to continuously enhance and evolve them, improving their efficiency and effectiveness.
It’s this focus on continuous enhancement that defines our AI-powered DAMO™ Managed Services, helping us take application and infrastructure management to the next level.
Drive efficiency and reduce YoY run costs with AIOps
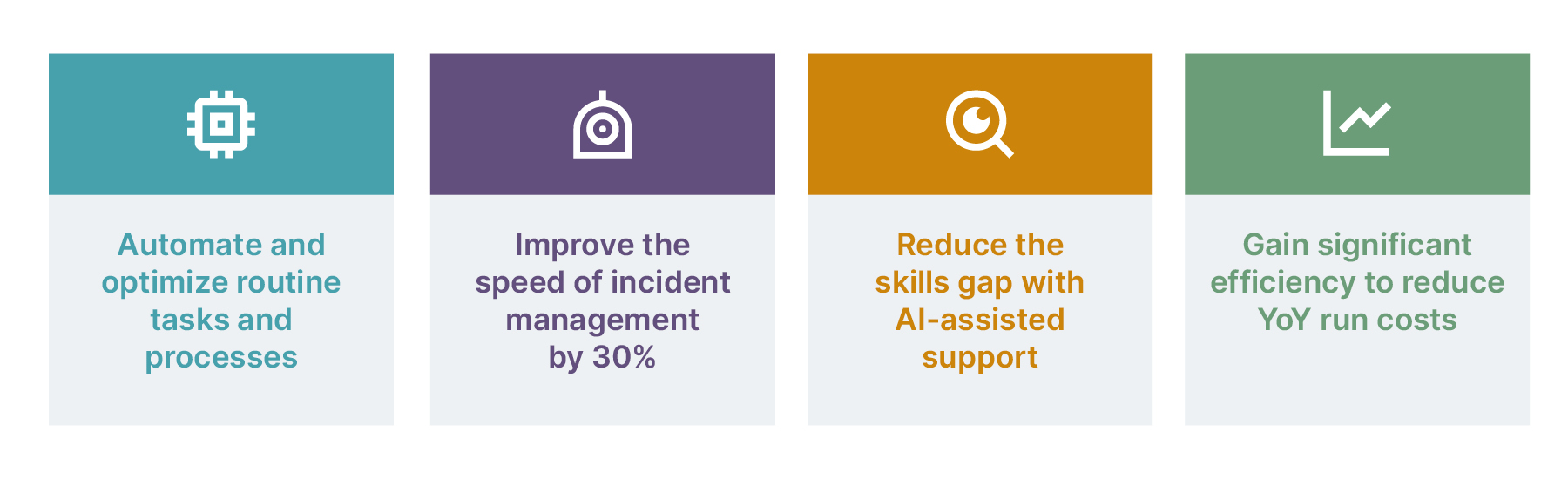
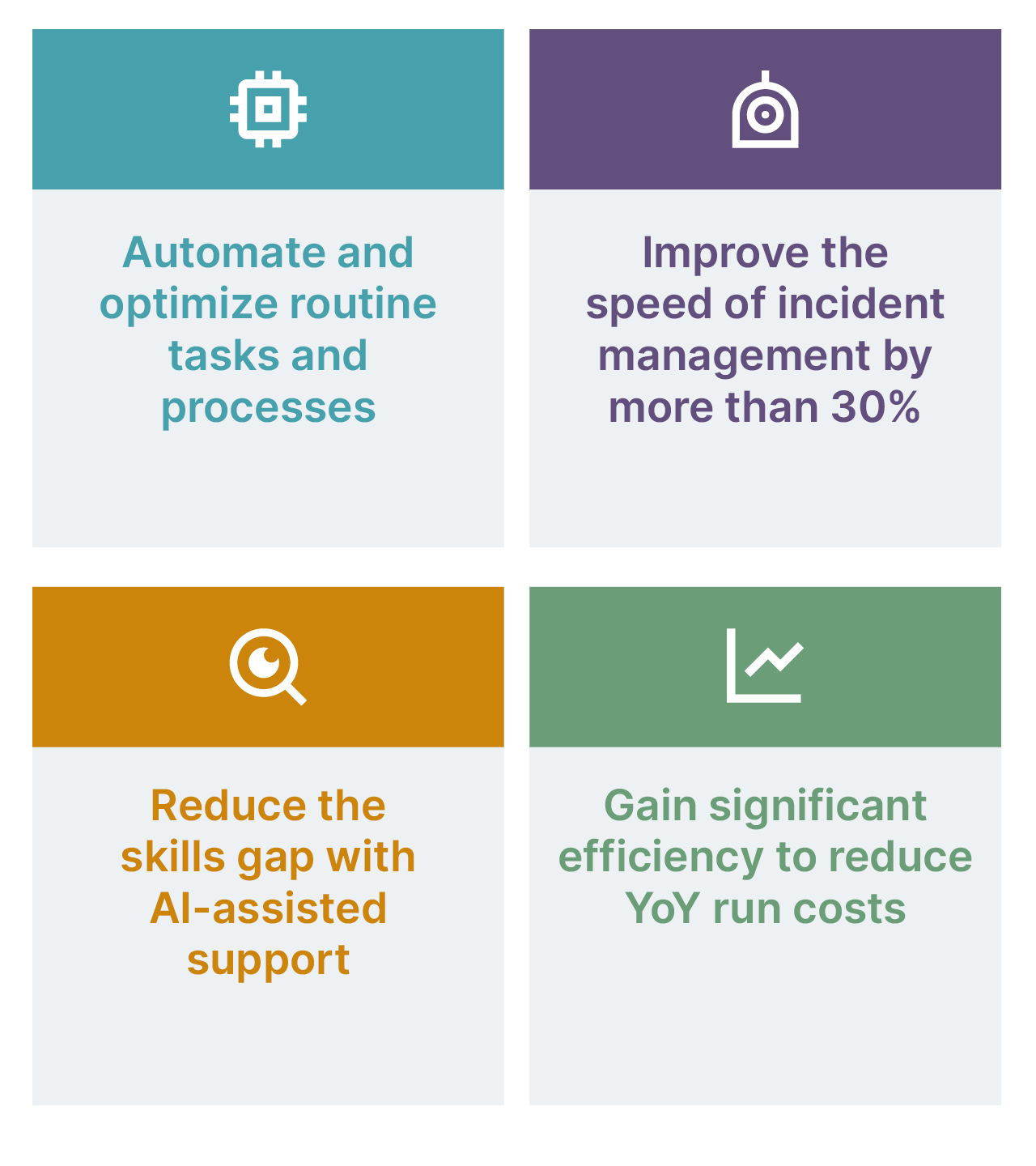
Thoughtworks’ AIOps solution infuses AI and machine learning algorithms in our fixed-cost managed services to automate and optimize routine management tasks and processes. This helps organizations operate more efficiently and effectively, accelerates issue resolution, and improves code quality.
AIOps also uses managed services data — such as call data and error logs — to recognize patterns, formulate responses, and conduct root-cause analysis. These AI-powered insights fuel a constant cycle of knowledge, discovery, and enhancement that allows us to make continuous, iterative improvements to applications. By operationalizing AI in managed services in this way, we’ve seen the speed of incident management — from problem to remediation — improve by more than 30%, in addition to significant reductions in year-over-year run costs.
This is what we mean when we talk about the next level of managed services. It’s about going beyond just sustaining applications and focusing on continuously enhancing and evolving them to eliminate the need for expensive “big bang” changes in the future. The efficiency gains and cost reductions we help our customers achieve enable them to invest in innovative initiatives and data products that deliver sustainable competitive advantage.
While integrating AI into managed services boasts notable benefits, there are several foundational capabilities that must be in place for success.
Essential foundations for AI-powered managed services
Self-learning AI will only ever be as good as the data it learns from, so consistently high standards of data quality and governance are vital. This is critical to avoiding AI hallucinations that can be caused by inaccurate training data, and incorrect assumptions.
But even with great data stewardship, simply layering AI over a broken process won’t fix the problem — and it may even exacerbate the issues. It’s important to document and understand your processes, and then redesign them where necessary to make them fit for purpose in an AI-powered world. If you haven’t already adopted CI/CD and DevOps practices or implemented testing automation, embed these things first before applying AI. Without modern data and engineering practices, AI tools could still provide a short-term efficiency gain by automating a limited set of routine tasks.
Want to learn more about how you can operationalize AI in managed services to realize long-term business value and tangible ROI? Get in touch with one of our AIOps experts today.