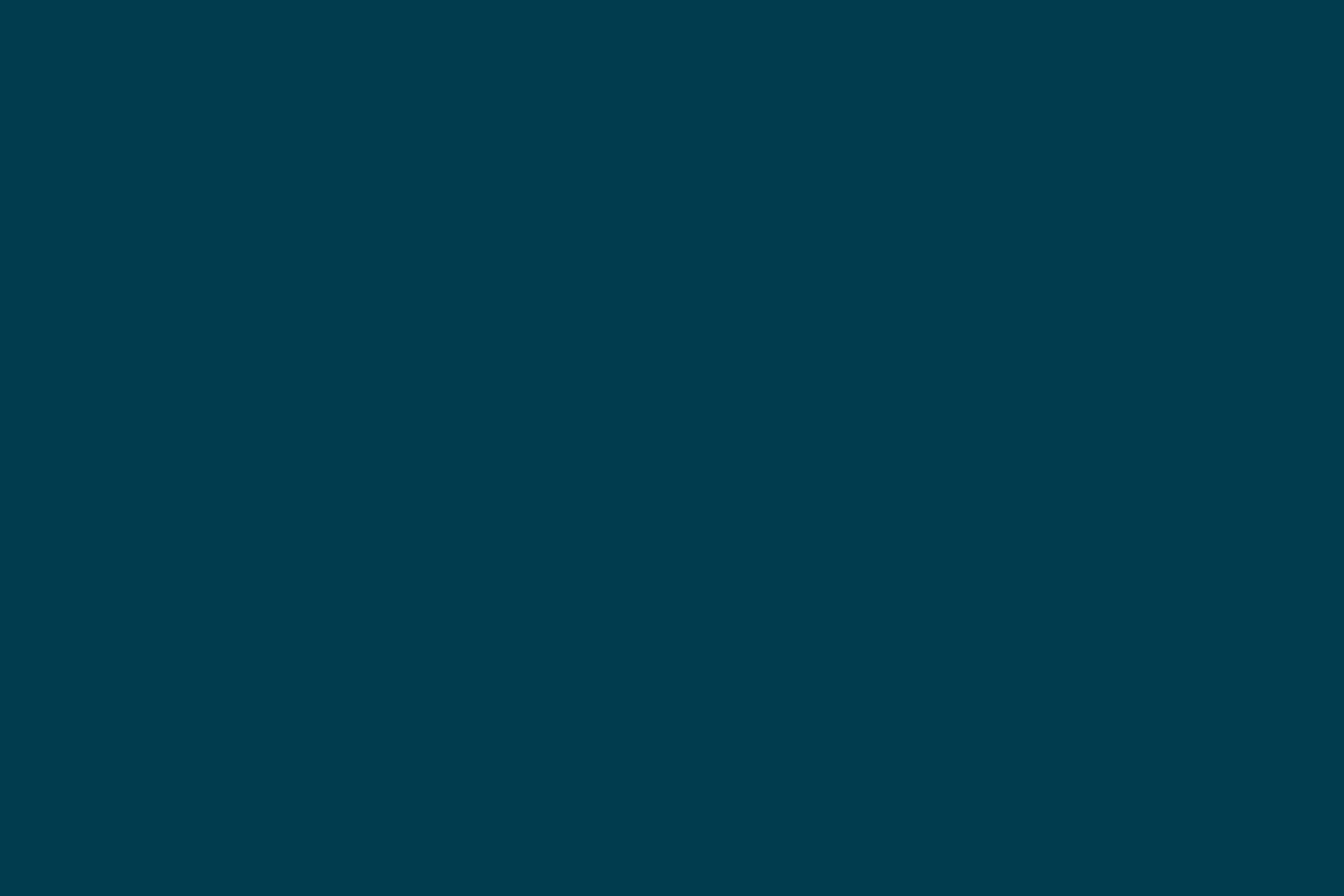
Our thoughts on the House of Lords AI Select Committee report on AI
Published: April 19, 2018
On Monday, April 16, the UK Government’s House of Lords Select Committee on Artificial Intelligence issued its report, AI in the UK: ready, willing and able? On April 26, 2018, a delegation of Thoughtworkers will present our viewpoints on this report at the House of Lords with IORMA, The Global Consumer Commerce Centre.
We’re encouraged to see government leaders taking steps to improve the U.K.’s strength in the field of AI. Given that U.K. law is used as a legal model all over the world for business, it could mean that issues surrounding ethics and privacy in AI might get closer attention and international agreement.
We are particularly interested in what the opportunity is for the UK to differentiate on AI? Increasingly companies have to think more like the tech companies that are encroaching on their markets. How can the dimensions of innovation, business growth, and regulation come together to give the UK an advantaged position in pushing a broad AI agenda that encompasses all these things and so leading adoption across non-US markets? Similarly for businesses, how can they find differentiation on a micro scale?

AI has struggled to be taken seriously in many circumstances, indeed many business leaders don’t yet know what its true capabilities are. AI has become so common in popular culture that its hard for the layperson to distinguish between the genuinely possible and sci-fi magic. And it’s not yet possible to regulate or legislate for magic! Nonetheless, AI has become a core part of business steadily over the last few years and that trend is set to steadily continue.
We believe it’s important to apply the same principles that we recommend for the internet itself (the independence of which is itself threatened); namely a decentralised, distributed, and open source approach to data that hinders dominance of the few and enables easier accountability of the source and provenance of the data itself.
Furthermore, changes in regulations, compliance, and legislation place increasing demands on data, leaving many companies at a disadvantage to those that have invested in a data strategy and infrastructure which takes full advantage of data-driven support systems powered by machine learning and artificial intelligence.
We believe that companies need to become data-driven. But this term lacks an adequate definition. A company needs to be able to make informed decisions based on data provided from all of its systems. In other words, they need to be able to track, trace and retain the data and its provenance, as well as all the decision making history. All business-related data needs to be readily available for both audit and query purposes downstream from all systems.
It might be helpful to think in terms of systems that store or change data needing to emit an exhaust of transactions that are subsequently available for further analysis. Some call this a ‘data lake’ — a centralization of data for further use. It does not necessarily need to be centralized — in some ways that can be a disadvantage. It just needs to be made available.
Of course, we see automated decision making using machine learning and other forms of AI growing in both the public (legal) and private (medical, education) sectors. It’s possible to see chains of decisions that lead to a particular outcome; however, much of that decision-making process happens within a black box. Therefore, it’s imperative that transparency of the decision-making process is present throughout.
The emergent field of Explainable Artificial Intelligence - ‘XAI’ — is being investigated to address some of these concerns. Additionally, questions to consider central to your data strategy include:
People are also starting to speak more about ethical decision-making AI. There are many popular examples of decision making that might result in an outcome that excludes or indeed benefits one part of the population unfairly.
There’s also emergent thought on biases within data sets, as well as biased outcome decisions that isolate part of society due to naive, incorrect or somewhat ignorant algorithm design. It’ll be important to have full transparency to prevent an ethical drift that might occur, and that consumers and subsequent social media sentiment data detects before the decision makers do, resulting in irreparable political damage. We have seen many references that suggest Millennials and Generation Z consumers demand an ethical corporate stance; they expect both good value for their money and trustworthiness among the brands they choose.
Finally, companies need to consider ‘architecting for good’.
This means:
In closing, we welcome much of the sentiment of the report. It talks of the investment in capabilities required to prepare for the future, additional overseas talent required (which is counter to some perceptions of Brexit) and allows us to express our own viewpoints alongside.
However, terms such as data as currency have been adopted for a while now. Usage and manipulation of data are big business. We cannot underestimate the chance of people being swept along somewhat unaware of exactly what is being done with information about them. Ethics bring the discussions back to the fact that real people’s information is being used here. While we need to be careful that we respect people’s privacy there is growing evidence that doing things right can be commercially beneficial. It may well pay to be good.
We’re encouraged to see government leaders taking steps to improve the U.K.’s strength in the field of AI. Given that U.K. law is used as a legal model all over the world for business, it could mean that issues surrounding ethics and privacy in AI might get closer attention and international agreement.
We are particularly interested in what the opportunity is for the UK to differentiate on AI? Increasingly companies have to think more like the tech companies that are encroaching on their markets. How can the dimensions of innovation, business growth, and regulation come together to give the UK an advantaged position in pushing a broad AI agenda that encompasses all these things and so leading adoption across non-US markets? Similarly for businesses, how can they find differentiation on a micro scale?
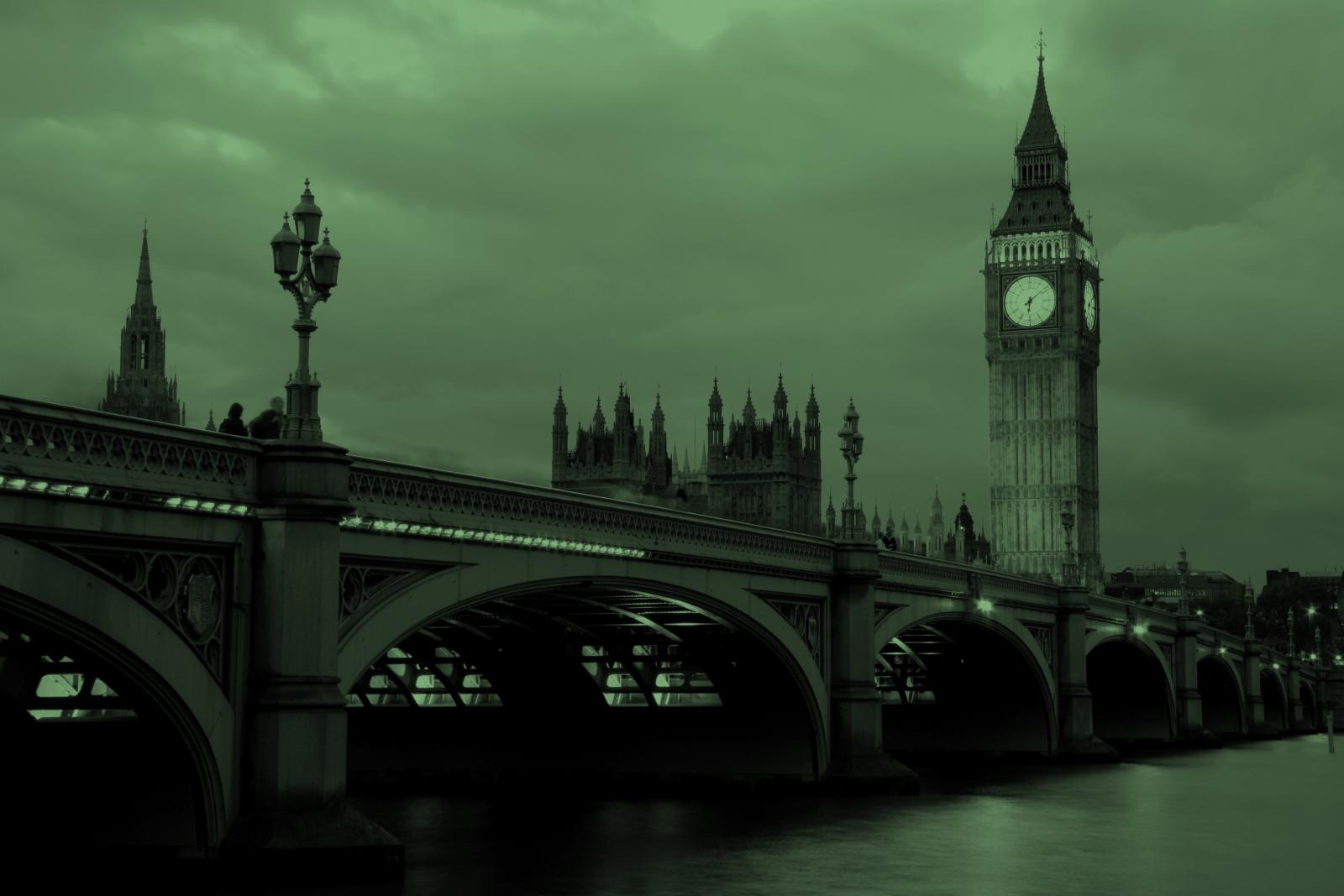
AI has struggled to be taken seriously in many circumstances, indeed many business leaders don’t yet know what its true capabilities are. AI has become so common in popular culture that its hard for the layperson to distinguish between the genuinely possible and sci-fi magic. And it’s not yet possible to regulate or legislate for magic! Nonetheless, AI has become a core part of business steadily over the last few years and that trend is set to steadily continue.
The dangers of a data monopoly
The report calls out the probable dangers inherent in the fact that a small number of companies currently own the lion’s share of the world’s data. We would further add that there will be a race to achieve dominance which could result in a monopoly. In all cases, companies such as Apple, Amazon, Google, Facebook, and Microsoft have tools and developer platforms that make machine learning and AI techniques more easily available. All of them are trying to entice product makers to use their cloud platforms.We believe it’s important to apply the same principles that we recommend for the internet itself (the independence of which is itself threatened); namely a decentralised, distributed, and open source approach to data that hinders dominance of the few and enables easier accountability of the source and provenance of the data itself.
Becoming a data-driven company
This brings us to our view of data-driven systems in the commercial world. Many companies’ technical estates don’t enable readily, flexible access to the data to satisfy their reporting, system, or ad-hoc needs — it simply takes too long to get new answers to questions they wish to ask of their data, and the quality of the data may not be worth the trouble.Furthermore, changes in regulations, compliance, and legislation place increasing demands on data, leaving many companies at a disadvantage to those that have invested in a data strategy and infrastructure which takes full advantage of data-driven support systems powered by machine learning and artificial intelligence.
We see this as a key ingredient of gaining advantage through digital transformation.
We believe that companies need to become data-driven. But this term lacks an adequate definition. A company needs to be able to make informed decisions based on data provided from all of its systems. In other words, they need to be able to track, trace and retain the data and its provenance, as well as all the decision making history. All business-related data needs to be readily available for both audit and query purposes downstream from all systems.
It might be helpful to think in terms of systems that store or change data needing to emit an exhaust of transactions that are subsequently available for further analysis. Some call this a ‘data lake’ — a centralization of data for further use. It does not necessarily need to be centralized — in some ways that can be a disadvantage. It just needs to be made available.
Privacy by design
Decisioning, either manual or automated, is only as good as the quality of its source data. That quality depends on a number of factors including how information is obtained, sifted for relevance, and filtered. Having a clear idea of what data is actually required means that compliance or public opinion on privacy concerns might be more easily addressed if data’s stored carefully, employing privacy by design concepts from the outset — something we believe’s a fundamental right of the individual. Given the recent increase in national regulations on data, we foresee a future with increased consumer awareness and the primacy of individuals’ rights to control data; this in turn leads to an increase in regulations such as GDPR. Re-architecting systems to treat people more fairly according to their rights means that companies are better placed to protect against brand damage or regulatory or legislative change in the future.Of course, we see automated decision making using machine learning and other forms of AI growing in both the public (legal) and private (medical, education) sectors. It’s possible to see chains of decisions that lead to a particular outcome; however, much of that decision-making process happens within a black box. Therefore, it’s imperative that transparency of the decision-making process is present throughout.
The emergent field of Explainable Artificial Intelligence - ‘XAI’ — is being investigated to address some of these concerns. Additionally, questions to consider central to your data strategy include:
- What data sets were used?
- How were they filtered?
- When were they sampled?
- What modeling techniques were used?
- What configuration parameters fed in?
- And what version of the algorithms were required to reproduce results either in root cause analysis or in checking past predictions with reality?
People are also starting to speak more about ethical decision-making AI. There are many popular examples of decision making that might result in an outcome that excludes or indeed benefits one part of the population unfairly.
There’s also emergent thought on biases within data sets, as well as biased outcome decisions that isolate part of society due to naive, incorrect or somewhat ignorant algorithm design. It’ll be important to have full transparency to prevent an ethical drift that might occur, and that consumers and subsequent social media sentiment data detects before the decision makers do, resulting in irreparable political damage. We have seen many references that suggest Millennials and Generation Z consumers demand an ethical corporate stance; they expect both good value for their money and trustworthiness among the brands they choose.
Finally, companies need to consider ‘architecting for good’.
This means:
- Transitioning the technical estate to provide fine-grained data events for downstream analysis
- Carefully selecting and storing data for precise (and provable) needs rather than collecting massive amounts of data ‘in case it’s needed’
- Tracking all identifiable data held, its uses and movements — especially where shared with any third party, so that this can be shared with data subjects, both individuals and organisations
- Adopt privacy by design principles from the outset to allow maximum future flexibility of demands on data
- Consider the ethical position brands need to adopt in terms of privacy and AI decision making, test with various demographics to prevent intrinsic bias
- All decision making needs to be transparent and reproducible for analysis and risk
- Build upon lean principles to facilitate short iterative planning, production and testing cycles with consumers. In other words: get out there testing impact with real people
- Further, build using Continuous Delivery principles to ensure rigorous quality assurance and frequent releases. This gives the engineering foundation and rigor to facilitate the previous point.
In closing, we welcome much of the sentiment of the report. It talks of the investment in capabilities required to prepare for the future, additional overseas talent required (which is counter to some perceptions of Brexit) and allows us to express our own viewpoints alongside.
However, terms such as data as currency have been adopted for a while now. Usage and manipulation of data are big business. We cannot underestimate the chance of people being swept along somewhat unaware of exactly what is being done with information about them. Ethics bring the discussions back to the fact that real people’s information is being used here. While we need to be careful that we respect people’s privacy there is growing evidence that doing things right can be commercially beneficial. It may well pay to be good.
Disclaimer: The statements and opinions expressed in this article are those of the author(s) and do not necessarily reflect the positions of Thoughtworks.