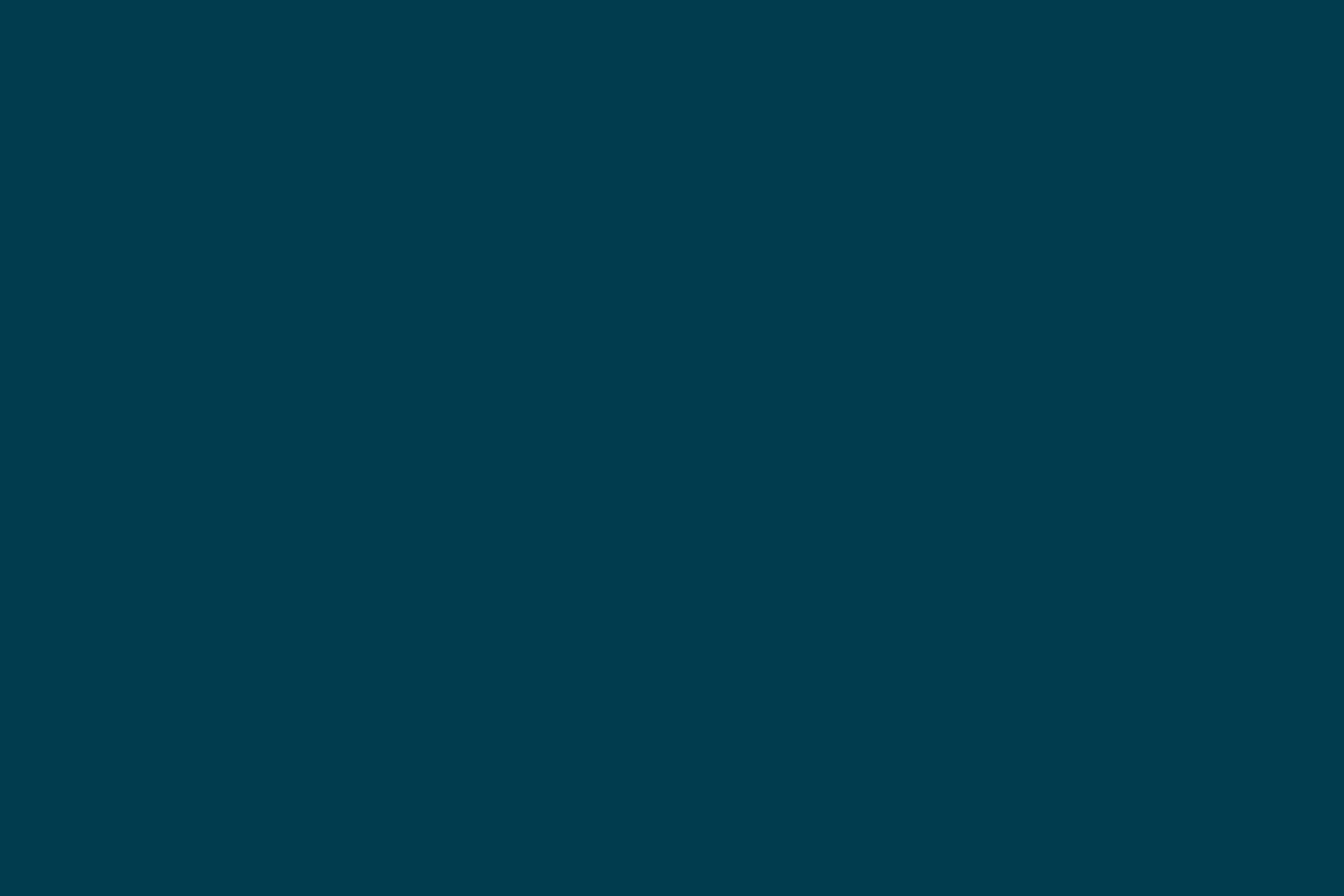
The Promise and Perils of AI in Compliance
Published: December 14, 2016
“It is no longer sufficient to do sampling for auditing, you have to boil the ocean," according to Joseph Lodato, global head of compliance technology and surveillance at Guggenheim Partners, in his keynote at the RegTech Summit US. Organizations are now required to trawl through the plethora of emails they send and receive each day to ensure they comply with regulations during an SEC examination. Making technology all the more important, a machine learning solution that flags suspicious emails would be advantageous in such a situation.
As the remit for compliance officers continues to expand, they are increasingly looking at technology to augment their capabilities. Artificial intelligence powered by machine learning and big data has the potential to completely revolutionize the compliance world.

Machine learning solutions are already widely used in front office activities such as dynamic portfolio rebalancing and high-frequency trading. We are now witnessing the first wave of regulatory compliance solutions that use artificial intelligence for delivering efficiency through automation and comprehensive risk coverage.
Solutions exist today to understand and analyze the high volume of regulatory changes. Natural Language Processing (NLP) solutions can parse regulatory text and pattern match with a cluster of keywords to identify the changes relevant to the organization.
Capital stress testing solutions use predictive analytics and scenario builders to help organizations remain compliant with regulatory capital requirements.
Huge volumes of conversations from phone recordings, chats and emails can now be analyzed using voice and text analysis algorithms to determine unusual employee behavior. Contextual analysis of these conversations can help identify potential market manipulation and collusion activities.
Money laundering transactions have traditionally been uncovered using old-school investigative methods and static business rules to highlight suspicious activities. Using deep learning techniques on the transactions, the business rules can become more sophisticated and significantly reduce the volume of activities flagged for investigation.
The current wave of these types of solutions highlight what is possible for addressing specific needs. They are heavily focused on efficiency, reduction of false positives, accuracy and providing better coverage than sampling.
The next wave of solutions will need to be more comprehensive and cover the lifecycle of all events that matter to compliance teams. Spanning across the customer journey, these solutions would need to solve for the actual concern of the compliance officer. For example, to establish collusion amongst market participants, employee activity data would need to be contextualized with communication logs as well as market movements.
Many executives are keen to latch on to this promise of machine learning solutions and leverage the rich data that is being collected. A word of caution though—acquiring the technology is not the panacea. Fred Brooks sums it up well in his popular paper No Silver Bullet. “There is no single development, in either technology or in management technique, that by itself promises even one order-of-magnitude improvement in productivity, in reliability, in simplicity”. Every organization needs to start with the problem they need to solve, before they embark on technology choices.
As executives focus on the problems they need to solve with AI, they also need to be mindful of the risks that an AI solution can bring. Some of these risks are not new. For example herding behavior, out-of-sample extrapolation, and spurious correlations, to name a few. However, there are some additional risks to consider as compliance teams become more technology driven and reliant on machines to do their jobs.
1. Amplified impact of misinformation
In 2013, a single bogus tweet from a verified account about an explosion in the White House briefly wiped out about $140 billion in US market value. The incident serves to highlight the systemic risk posed by trading algorithms vulnerable to fake, unverifiable news.
We have already seen that the proliferation of fake news during the election may have influenced the final outcome. As is often the case, the advantage is often the curse: tons of information processed and widely transmitted instantly.
In an AI driven world, the ability to manipulate the market using fake news or social media takeover is significantly amplified.
2. Codification of bias
Discrimination against a particular gender, race or social class can be perpetuated by technology. AI learns this discrimination through historical data, which can often be skewed for decision making. Take for instance word embedding algorithms in NLP. These algorithms have the ability to carry historical stereotypes (sexism for example) into the future simply by learning patterns regarding words that often appear together in historical data. Use of AI can result in such biases creeping into business practices such as lending or hiring.
3. The distance problem
Automating decisions based on known conditions can deliver massive efficiency for the compliance professionals. On the flip side, this increases the distance of the compliance professional from the actual decision-making process. For example, an NLP solution that tracks thousands of regulatory changes can deliver a false sense of confidence about the comprehensiveness of coverage.
AI also creates an opacity for the regulators in establishing whether due process is being followed for the different business practices such as sanctions screening or suspicious activity reporting.
4. Ethics
Mercedes-Benz’s self-driving car chooses passenger safety over pedestrian safety by design. In the banking world, AI can be used for activities that have potential conflicts of interest such as portfolio allocation, trading, and investment advice. The organization needs to have policies and principles that guide the design of such algorithms. Industry leaders like Satya Nadella of Microsoft have suggested ten laws that should govern the design of an AI.
These risks are particularly important for compliance divisions, whose raison d’etre is to protect the institution rather than introduce “hidden” risks. Such risks can undo the whole institutions performance, wiping out the edge on its revenue generation side. The tech world including Microsoft, Amazon and Facebook have recognized these risks and have been developing ethical best practices for AI development.
The volume of regulatory changes, increased sophistication of fraudsters as well as increased scrutiny from regulators will inevitably make compliance teams turn to AI. Given the additional considerations, here are some of our recommendations for adopting a machine learning solution within a compliance division:
1. Change the team mix
The revenue side of financial institutions have always been early to adopt the latest technologies that have the potential to give a competitive edge, be it from the world of mathematics, computer science and data science. That has been reflected in the evolution of the “typical” trader over time: from college graduates that learned the art of trading next to the veterans 20 years ago, to quants with PhDs in math and science in more recent times.
Compliance teams have traditionally comprised of lawyers and risk professionals. As these activities becomes more tech driven, there is a need to change the mix to include more technical people and data scientists to bridge the knowledge gap. Active involvement during the development and integration, such as specifying and testing the outcomes, go a long way to ensure that the solution does not remain inscrutable.
2. Build confidence gradually
Business leaders need to be thoughtful about where and how to apply machine learning. Confidence on such solutions needs to be built gradually in the algorithm and the data. We have already witnessed how wide adoption of a not-so-well understood formula can lead to total catastrophe with the Gaussian Copula function to price mortgage-backed securities.
Executives need an experimental mindset to validate the data insights and automate the decisions. “Garbage in Garbage out” is an industry clichè but nevertheless true. A good data engineering approach that delivers clean and timely data is absolutely essential.
A phased approach should be adopted for developing the algorithmic solution. Automate the more mundane decisions initially, followed by the more critical ones later. Parallel run the solution to ensure the consistency of decision making and make active efforts to continue training the solution.
To confirm the experiments, a cross-validation of conclusions should occur via different data sources well before any action is taken before the misinformation ends up reinforcing itself.
3. Maintenance
Regulations and their interpretations and consequences are always changing. Thus, it is important to continually update the suite of tests to reflect these changes and continuously adapt the algorithms themselves when needed to avoid getting blindsided. The model itself can become stale over a period of time as the data it has learned from is no longer relevant.This can be particularly true when it comes to organized financial criminal activity, for example, money laundering, which by design is meant to escape detection. To achieve that, it is conceivable that the methods used for such criminal activities are constantly evolving; that is to say that in principle, the same advances that can help enforce regulatory compliance can also enable smarter financial crimes.
4. Manage expectations
Stakeholders need to recognize the limitations of machine learning and AI in solving a problem. It may find hidden insights from a vast amount of data, but it will be unable to solve the problem on its own or point out the problems we should actually be solving. In the context of compliance problems, the machine learning is operational rather than cognitive. The learning depends on the data being fed into it and the algorithms we direct it to use.
Adopting an experimentation mindset with the expectation to fail frequently, and the hope to fail fast will also be a necessity in this journey. Experiments will fail, either because of suboptimal choice of algorithm or because of data unavailability and quality issues. Often because of both.
It is important that stakeholders understand that all learning occurs from past experience. Therefore, they need to acknowledge and budget for the risk of missing black swan events.
There will be a proliferation of AI solutions in the future as computing gets cheaper, data availability becomes higher and technology becomes democratized. AI will pervade the compliance world by augmenting professionals in their jobs. A thoughtful approach to implementing AI solutions with a close eye on risks posed can deliver significant business value for the organizations.
As the remit for compliance officers continues to expand, they are increasingly looking at technology to augment their capabilities. Artificial intelligence powered by machine learning and big data has the potential to completely revolutionize the compliance world.
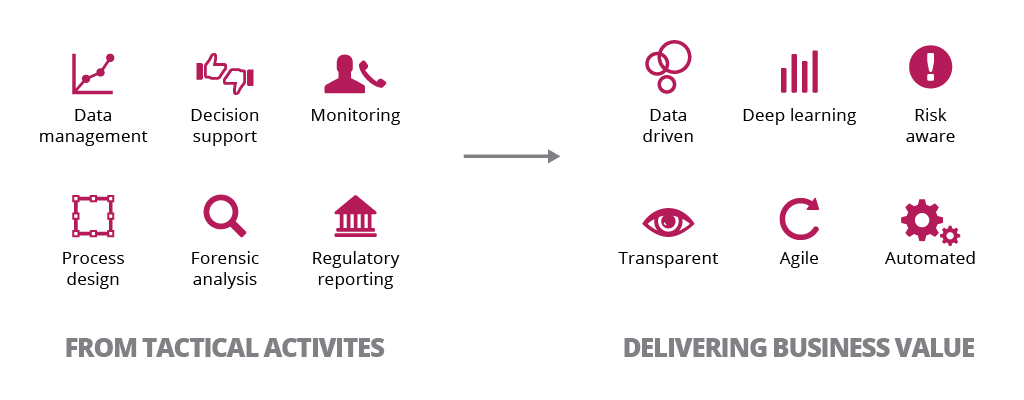
Machine learning solutions are already widely used in front office activities such as dynamic portfolio rebalancing and high-frequency trading. We are now witnessing the first wave of regulatory compliance solutions that use artificial intelligence for delivering efficiency through automation and comprehensive risk coverage.
Solutions exist today to understand and analyze the high volume of regulatory changes. Natural Language Processing (NLP) solutions can parse regulatory text and pattern match with a cluster of keywords to identify the changes relevant to the organization.
Capital stress testing solutions use predictive analytics and scenario builders to help organizations remain compliant with regulatory capital requirements.
Huge volumes of conversations from phone recordings, chats and emails can now be analyzed using voice and text analysis algorithms to determine unusual employee behavior. Contextual analysis of these conversations can help identify potential market manipulation and collusion activities.
Money laundering transactions have traditionally been uncovered using old-school investigative methods and static business rules to highlight suspicious activities. Using deep learning techniques on the transactions, the business rules can become more sophisticated and significantly reduce the volume of activities flagged for investigation.
The current wave of these types of solutions highlight what is possible for addressing specific needs. They are heavily focused on efficiency, reduction of false positives, accuracy and providing better coverage than sampling.
The next wave of solutions will need to be more comprehensive and cover the lifecycle of all events that matter to compliance teams. Spanning across the customer journey, these solutions would need to solve for the actual concern of the compliance officer. For example, to establish collusion amongst market participants, employee activity data would need to be contextualized with communication logs as well as market movements.
Many executives are keen to latch on to this promise of machine learning solutions and leverage the rich data that is being collected. A word of caution though—acquiring the technology is not the panacea. Fred Brooks sums it up well in his popular paper No Silver Bullet. “There is no single development, in either technology or in management technique, that by itself promises even one order-of-magnitude improvement in productivity, in reliability, in simplicity”. Every organization needs to start with the problem they need to solve, before they embark on technology choices.
As executives focus on the problems they need to solve with AI, they also need to be mindful of the risks that an AI solution can bring. Some of these risks are not new. For example herding behavior, out-of-sample extrapolation, and spurious correlations, to name a few. However, there are some additional risks to consider as compliance teams become more technology driven and reliant on machines to do their jobs.
1. Amplified impact of misinformation
In 2013, a single bogus tweet from a verified account about an explosion in the White House briefly wiped out about $140 billion in US market value. The incident serves to highlight the systemic risk posed by trading algorithms vulnerable to fake, unverifiable news.
We have already seen that the proliferation of fake news during the election may have influenced the final outcome. As is often the case, the advantage is often the curse: tons of information processed and widely transmitted instantly.
In an AI driven world, the ability to manipulate the market using fake news or social media takeover is significantly amplified.
2. Codification of bias
Discrimination against a particular gender, race or social class can be perpetuated by technology. AI learns this discrimination through historical data, which can often be skewed for decision making. Take for instance word embedding algorithms in NLP. These algorithms have the ability to carry historical stereotypes (sexism for example) into the future simply by learning patterns regarding words that often appear together in historical data. Use of AI can result in such biases creeping into business practices such as lending or hiring.
3. The distance problem
Automating decisions based on known conditions can deliver massive efficiency for the compliance professionals. On the flip side, this increases the distance of the compliance professional from the actual decision-making process. For example, an NLP solution that tracks thousands of regulatory changes can deliver a false sense of confidence about the comprehensiveness of coverage.
AI also creates an opacity for the regulators in establishing whether due process is being followed for the different business practices such as sanctions screening or suspicious activity reporting.
4. Ethics
Mercedes-Benz’s self-driving car chooses passenger safety over pedestrian safety by design. In the banking world, AI can be used for activities that have potential conflicts of interest such as portfolio allocation, trading, and investment advice. The organization needs to have policies and principles that guide the design of such algorithms. Industry leaders like Satya Nadella of Microsoft have suggested ten laws that should govern the design of an AI.
These risks are particularly important for compliance divisions, whose raison d’etre is to protect the institution rather than introduce “hidden” risks. Such risks can undo the whole institutions performance, wiping out the edge on its revenue generation side. The tech world including Microsoft, Amazon and Facebook have recognized these risks and have been developing ethical best practices for AI development.
The volume of regulatory changes, increased sophistication of fraudsters as well as increased scrutiny from regulators will inevitably make compliance teams turn to AI. Given the additional considerations, here are some of our recommendations for adopting a machine learning solution within a compliance division:
1. Change the team mix
The revenue side of financial institutions have always been early to adopt the latest technologies that have the potential to give a competitive edge, be it from the world of mathematics, computer science and data science. That has been reflected in the evolution of the “typical” trader over time: from college graduates that learned the art of trading next to the veterans 20 years ago, to quants with PhDs in math and science in more recent times.
Compliance teams have traditionally comprised of lawyers and risk professionals. As these activities becomes more tech driven, there is a need to change the mix to include more technical people and data scientists to bridge the knowledge gap. Active involvement during the development and integration, such as specifying and testing the outcomes, go a long way to ensure that the solution does not remain inscrutable.
2. Build confidence gradually
Business leaders need to be thoughtful about where and how to apply machine learning. Confidence on such solutions needs to be built gradually in the algorithm and the data. We have already witnessed how wide adoption of a not-so-well understood formula can lead to total catastrophe with the Gaussian Copula function to price mortgage-backed securities.
Executives need an experimental mindset to validate the data insights and automate the decisions. “Garbage in Garbage out” is an industry clichè but nevertheless true. A good data engineering approach that delivers clean and timely data is absolutely essential.
A phased approach should be adopted for developing the algorithmic solution. Automate the more mundane decisions initially, followed by the more critical ones later. Parallel run the solution to ensure the consistency of decision making and make active efforts to continue training the solution.
To confirm the experiments, a cross-validation of conclusions should occur via different data sources well before any action is taken before the misinformation ends up reinforcing itself.
3. Maintenance
Regulations and their interpretations and consequences are always changing. Thus, it is important to continually update the suite of tests to reflect these changes and continuously adapt the algorithms themselves when needed to avoid getting blindsided. The model itself can become stale over a period of time as the data it has learned from is no longer relevant.This can be particularly true when it comes to organized financial criminal activity, for example, money laundering, which by design is meant to escape detection. To achieve that, it is conceivable that the methods used for such criminal activities are constantly evolving; that is to say that in principle, the same advances that can help enforce regulatory compliance can also enable smarter financial crimes.
4. Manage expectations
Stakeholders need to recognize the limitations of machine learning and AI in solving a problem. It may find hidden insights from a vast amount of data, but it will be unable to solve the problem on its own or point out the problems we should actually be solving. In the context of compliance problems, the machine learning is operational rather than cognitive. The learning depends on the data being fed into it and the algorithms we direct it to use.
Adopting an experimentation mindset with the expectation to fail frequently, and the hope to fail fast will also be a necessity in this journey. Experiments will fail, either because of suboptimal choice of algorithm or because of data unavailability and quality issues. Often because of both.
It is important that stakeholders understand that all learning occurs from past experience. Therefore, they need to acknowledge and budget for the risk of missing black swan events.
There will be a proliferation of AI solutions in the future as computing gets cheaper, data availability becomes higher and technology becomes democratized. AI will pervade the compliance world by augmenting professionals in their jobs. A thoughtful approach to implementing AI solutions with a close eye on risks posed can deliver significant business value for the organizations.
Disclaimer: The statements and opinions expressed in this article are those of the author(s) and do not necessarily reflect the positions of Thoughtworks.