Unlocking the true potential of artificial intelligence (AI) requires more than just implementing cutting-edge technologies. At Thoughtworks, we have witnessed firsthand the significant impact AI can have across various industries. For instance, we've developed AI-based systems that delivered double-digit improvements in homepage-to-funnel conversions for Marimekko, helped Mackmyra, a whisky distillery, to create the award-winning world's first AI whisky, and built Australia's most accurate property valuations tool. However, these achievements were only made possible by a strategic awareness of the immense opportunities that AI can unlock.
In this article, we delve into the AI gap analysis, outlining how organizations can get more value from investment in AI initiatives and bridge the divide between AI aspirations and tangible outcomes.
Components of value creation with AI
In our experience, several key components work in synergy to unlock the full potential of AI. Whether organizations opt for in-house development or third-party AI solutions, a crucial starting point is assessing the maturity level and identifying gaps between the current and the desired state across vital capabilities, that are outlined further. While developing new capabilities from scratch is often necessary, establishing solid foundations for exponential value creation with AI is a strategic imperative to succeed in the fast-paced landscape of our current era.
Achieving strategic alignment is a significant challenge when starting and scaling AI initiatives (Stanford AI Report 2023, p 213). AI solutions should address genuine problems and contribute to organizational goals. While evaluating AI investments in a manner similar to other strategic initiatives can justify upfront costs, quantifying overall ROI can ultimately be challenging due to the nature of AI. Measuring value early on while starting small can justify future attempts to scale.
High-quality data is vital for accurate predictions, recommendations, and automated decision-making. This means firms must not only set clearly defined goals for their initiatives, but they also need to have a good understanding of what data is required while being mindful that data needs may change during solution development due to AI's experimental nature. Adopting the principles of data ownership and self-service capabilities — emphasized in a Data Mesh approach — can ensure data is used most effectively in AI initiatives.
AI Talent. Building AI models requires specific skill sets such as data scientists, machine learning engineers, and software developers. However, achieving value from AI extends beyond technical expertise. Capabilities in design and product thinking are essential. Companies should invest in upskilling their employees to work effectively with AI, fostering a culture of learning and innovation.
Data & AI Infrastructure. AI practitioners need access to scalable computing systems, cloud-based platforms, and data storage solutions. Integration with existing tech stacks should also be considered, especially for vendor-managed solutions. Embracing continuous delivery (CD4ML) practices can ensure the efficient and reliable deployment of AI solutions.
Enterprise Adoption. Successful AI adoption often necessitates retraining employees and changing existing processes. It is crucial that change management is not overlooked, and involves targeted efforts to involve and gain buy-in from the people impacted early in the journey to overcome adoption barriers.
AI solutions - from initial implementation to monitoring and evolution. AI solutions differ from traditional software development as logic is learned from data, not predefined. Consequently, AI models require retraining when data changes. AI and ML solutions provide estimates, not 100% accuracy - hence ongoing monitoring, controls, and KPIs need to be in place to avoid unintended consequences and ensure business value continues to be generated. Processes and governance must be in place to integrate model changes into operations.
Data-driven culture. This is arguably the most challenging component to change, yet such a culture empowers organizations to leverage data as a strategic asset, driving informed decisions, actionable insights, and innovation. By fostering curiosity, experimentation, and continuous learning, a data-driven culture helps to uncover hidden patterns and opportunities. Ultimately, it's a prerequisite to unlocking the AI's full potential.
A capabilities gap analysis will accelerate value creation with AI
An AI gap analysis is an effective approach to identifying barriers and accelerating value creation with AI. It involves assessing organizational and technical components of value creation. The analysis involves identifying short-term gaps that can be solved with the right investment and resources, and larger challenges in culture and alignment.
Organizations can utilize a framework — such as the one we developed at Thoughtworks (see figure below) — for an effective AI gap analysis. This comprehensive framework assesses AI maturity and identifies gaps across important dimensions of value creation. While striving to become an ‘Industry Leader’ in every dimension may not be necessary for every firm, the framework recognizes the individual objectives and priorities that each organization has for AI in the enterprise. Tailoring the analysis to specific goals allows organizations to focus on critical gaps for success.
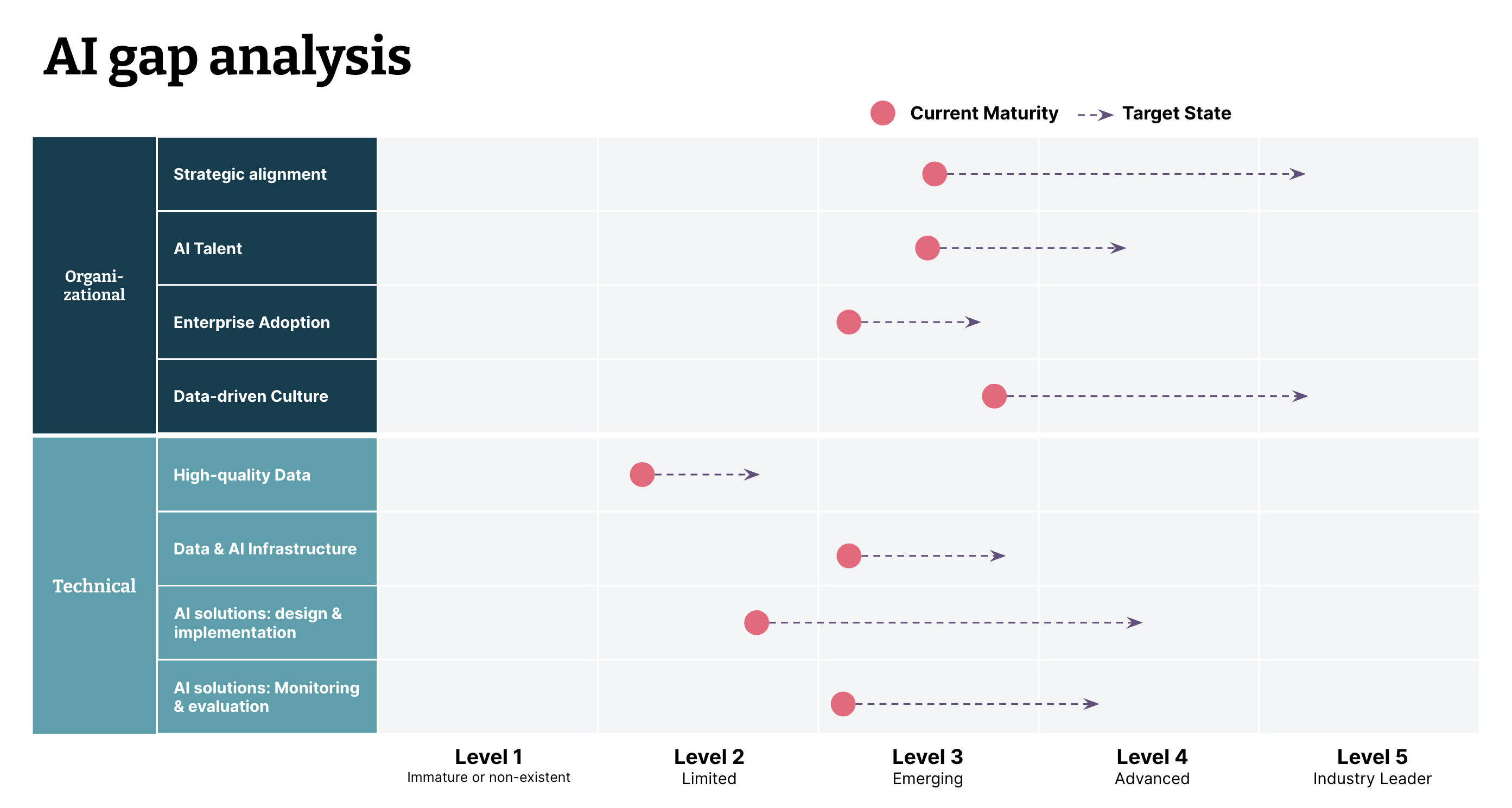
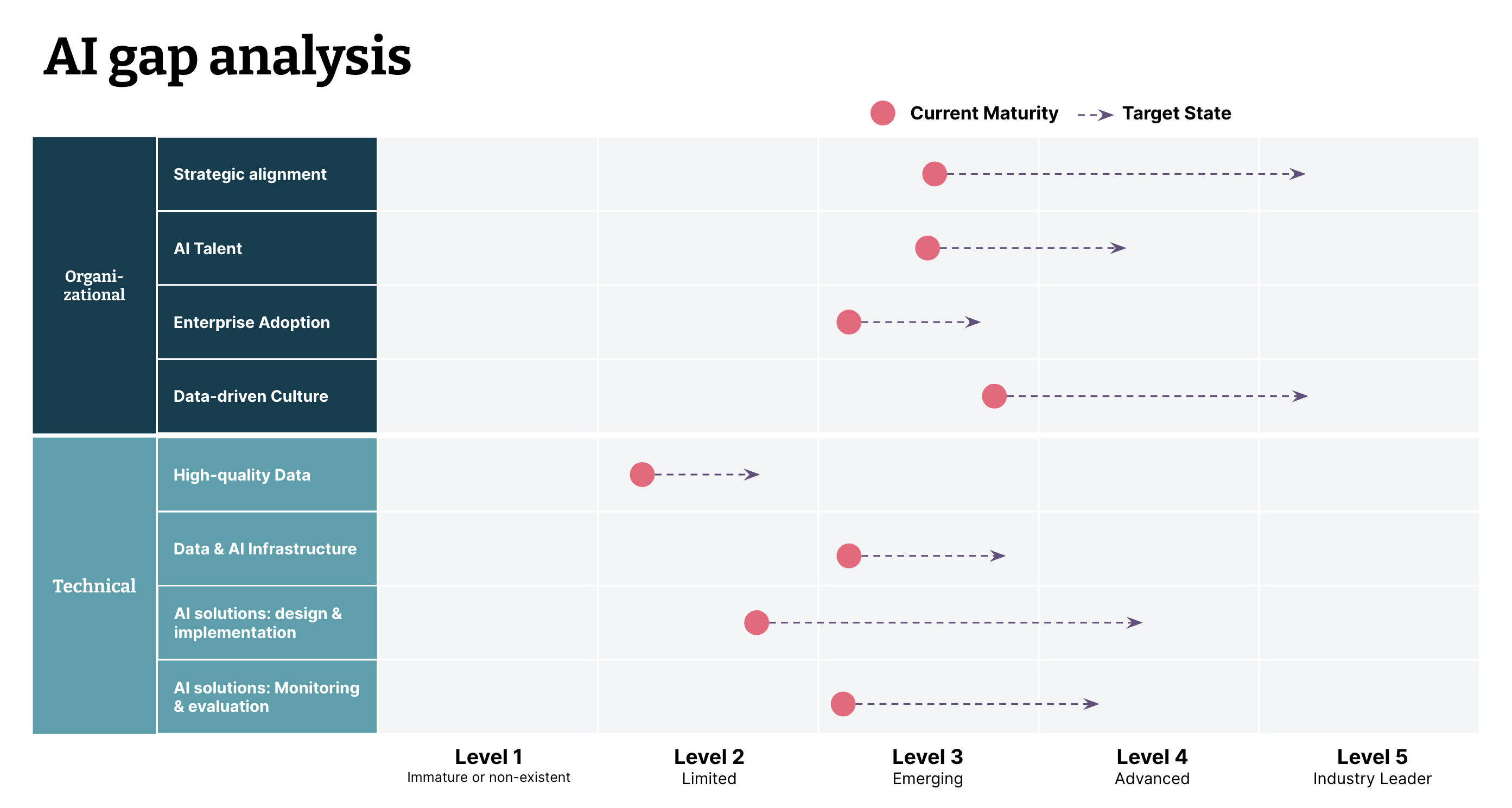
Businesses struggle to generate value with AI (HBR, 2023). Thoughtworks recently conducted an AI gap analysis for a diversified conglomerate to help them unlock value from AI faster. Our assessment revealed that the reasons for the value gap were not rooted in technology or AI models, but were instead, due to problems with alignment between AI practitioners and business strategy, a lack of incentives to prioritize business value over complex technical solutions, and a cultural aversion to experimentation and product thinking. Consequently, this hampered attempts to execute AI initiatives. Notably, our findings demonstrated that these organizational factors had a greater impact on value than technical challenges alone.
A Roadmap for maximizing value
Organizations would benefit from following a roadmap to counter the challenges inherent in AI initiatives, whilst maintaining a sharp focus on delivering value to the organization. In our experience, the successful roadmap includes the following steps:
1. Define an actionable AI vision
It is crucial to define a clear and actionable vision that aligns with your business strategy and goals. A robust AI vision goes beyond a mere statement of intent; it outlines the types of value your firm intends to pursue, the level of transformative change you aim to achieve, and the extent to which you are willing to invest resources to accomplish it.
2. Conduct AI gap analysis
Utilize a gap analysis framework (such as the one mentioned earlier) to evaluate your organization's current AI maturity level and identify the gaps between where you are and where you want to be. Understanding these gaps and their root causes is vital for developing a realistic roadmap to create value with AI. Armed with these insights, executives can make informed decisions on where to invest.
3. Craft an AI strategy tailored to the unique challenges and opportunities of AI
Aligning the AI strategy with the overall business strategy is a fundamental step in realizing value from AI and ensuring a cohesive approach. Begin formulating the strategy by identifying the specific "how" behind realizing your AI vision — gaps identified through the gap analysis can serve as input to the strategic roadmap. This roadmap should be actionable, with clearly defined milestones. It needs to be designed in such a way that it can be understood and embraced by your people.
4. Drive execution: start small, scale strategically, and foster an AI-centric ecosystem
Build momentum by initiating targeted proof of concepts (PoCs) and implementing local AI-enabled enhancements, while employing robust change management practices. This builds trust, boosts confidence, and mitigates risks. Integrate proven AI solutions into the organization, leveraging past experiences.
Aim to promote AI expertise, collaboration, and continuous innovation. It creates an AI-centric ecosystem, enabling adoption across departments and functions, evolving from isolated projects to a sustainable framework.
Align AI strategy with execution through a value-driven portfolio, connecting initiatives to organizational goals. This will enable monitoring of AI's contribution to driving business value.
5. Regularly evaluate business impact
Continuously monitor and curate the underlying data and evolve AI solutions based on market signals, employee feedback, and clearly defined KPIs. This iterative approach ensures that AI initiatives remain relevant and effective, and contribute to long-term value creation.
Accelerating value generation from AI investments requires a strategic approach that encompasses seeking strategic alignment, addressing data and infrastructure needs, nurturing AI talent, managing solutions evolution, and developing effective enterprise adoption strategies. By conducting an AI gap analysis and following a well-defined roadmap, organizations can overcome barriers, maximize value delivery, and unlock the full potential of AI.
At Thoughtworks, we have developed a comprehensive approach to helping firms deliver value with AI, leveraging principles of operating models for the digital age and our proprietary accelerators such as CD4ML, Data Mesh, AI Augmented, Decision Factories, and more.