The Wealth management (WM) industry is in the midst of an unprecedented change — largely driven by changing customer preferences. A new generation of investors are demanding more control over their investments and better value from the wealth advisory services. Increasing participation of millennials and women is changing the dynamics of advisor and client relationships. Moreover, a challenging investment environment, with a lot of uncertainties, is making it harder for advisors to generate superior performance for their clients. This has resulted in investors switching from active fund management to passive investments such as index funds and Exchange Traded Funds. Finally, increasing regulatory oversight is posing a big risk and eating margins of the WM industry.
To address the industry-wide structural changes, traditional brick-and-mortar wealth management firms are digitalizing and automating most of their processes. However, they’re losing out to digital-only wealthtech players, who leverage technological innovation to address the challenges. The wealthtechs are challenging traditional firms not only through better technology solutions but also through innovative business models such as commission-free investing and fractional-share investing. These new wealth managers are using big data and artificial intelligence to disrupt many of the old practices. And they’re gaining market share. Also, with big tech firms such as Amazon and Alibaba having plans to foray into wealth management, the competition is going to be even more fierce.
Below is a snapshot of some of the trends — driven by cutting-edge technologies — that will reshape the wealth management industry. We’ll explore trends driven by artificial intelligence in this article. We have already covered chatbots in our previous article Conversational AI in Capital Markets.
Trends to watch in wealth management:
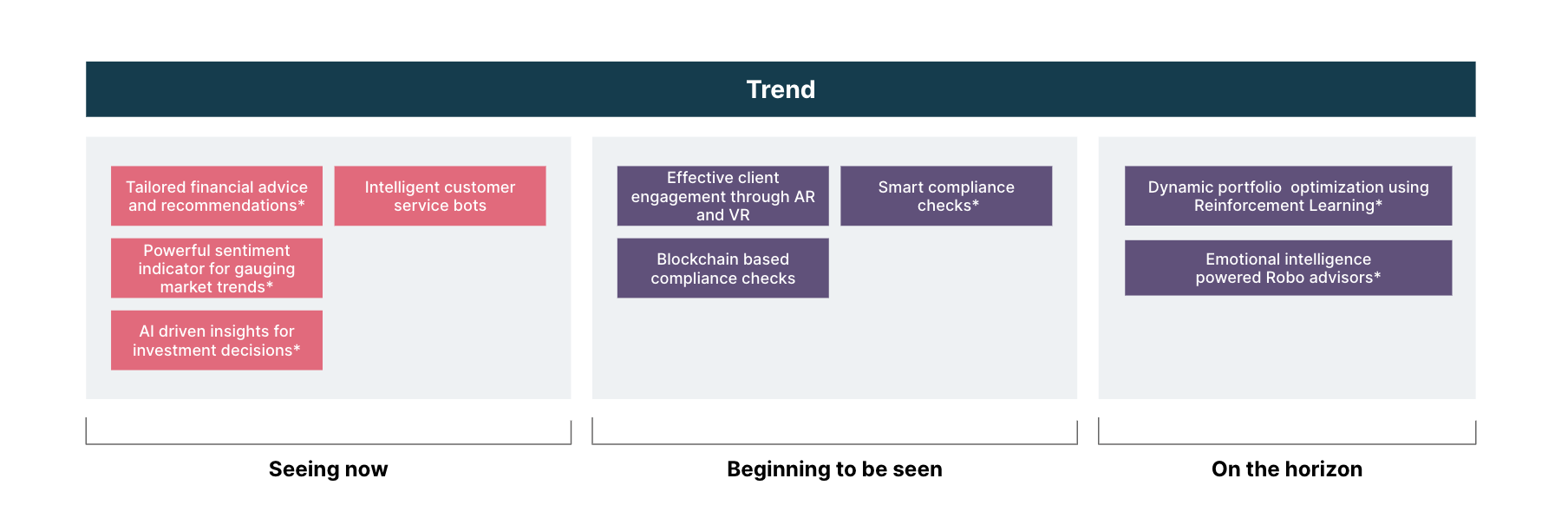
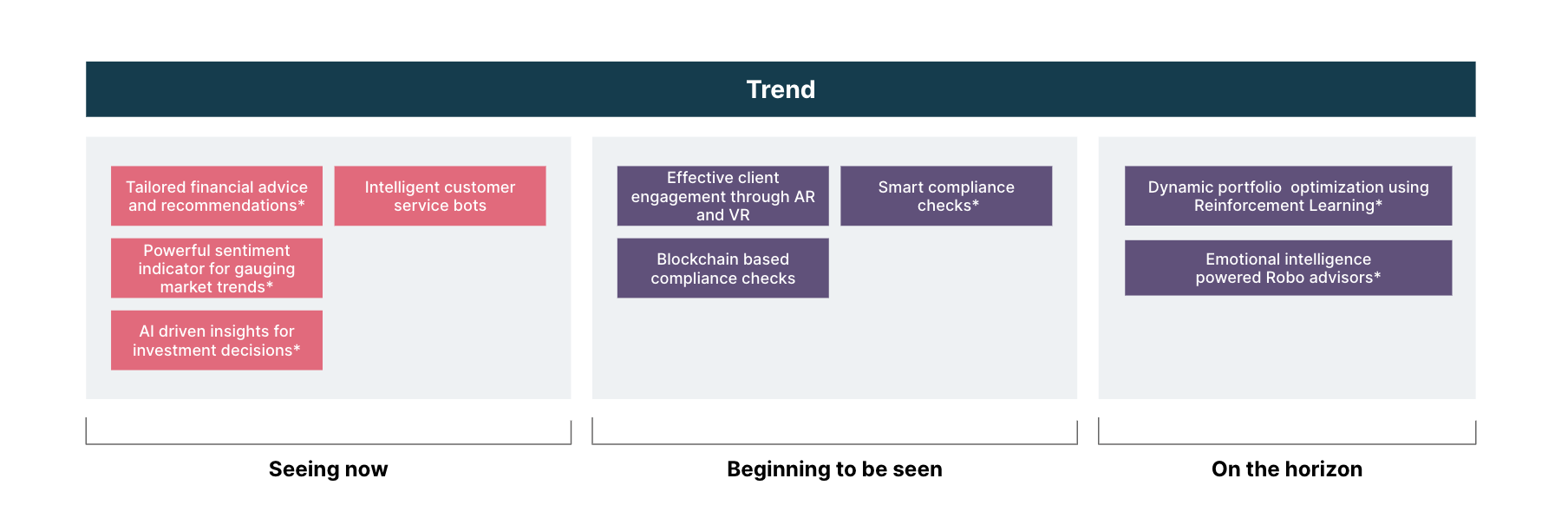
* Trends that will be touched upon in this article
Tailored financial advice and recommendation
Robo advisors or RAs use artificial intelligence tools to provide personalized and automated financial advice. These AI-powered RAs can manage all aspects of clients’ financial planning, from managing investments to suggesting whether the client has sufficient funds to spend.
Examples of these RAs include US-based Betterment and Singapore-based StashAway, which provide personalized, goal-based investing through their robo-advising platforms. Clients are able to interact with the RAs through conversational user interfaces, which are voice and chat enabled. RAs are able to service previously unprofitable client segments, because of their low fees and low upfront investments. And this has expanded the client base for wealth management firms. Deloitte reports that assets under management for robo-advisors are projected to reach $16 trillion by 2025.
Unlike human advisors, who would look at traditional data such as age and income, an RA can dig deeper by looking at alternate data sources, such as an individual’s social media feed and behavioral patterns from spending data. Based on the various data, RAs can create a ‘personalized investment plan’ for each investor. Some RAs also try to bring behavioral changes in saving habits, by looking at patterns of spending and suggesting ways to optimize it. US-based Wealthfront segregates client’s cash across different categories (like spending, savings and investments) based on the limits set by the client. They automatically invest extra money and periodically rebalance portfolios to bring them inline with the individual’s investment goals and risk profile, minimizing transaction costs and maximizing return.
Challenges with robo advisors
Regulatory
One major challenge that RAs face, which could limit adoption, is increased regulatory oversight. Like traditional investment advisors, robo advisors have to be registered with the local regulator in most countries. This means that robo advisors have to act ethically and in the best interests of their clients. Many of the AI tools that underpin these RAs — for instance artificial neural networks — are complex and opaque. This makes it difficult to provide explanation for how investment decisions are made — and wouldn’t therefore meet the firms’ fiduciary responsibility. As a result, we recommend testing algorithms underpinning RA in different market conditions and decisions made are recorded and audited. Ensure that design and design-making processes are documented clearly highlighting how potentially unintended consequences will be detected.
Behavioral Bias
Human advisors have certain biases, such as home bias — the tendency to invest the majority of portfolio in domestic securities — which impact their investment decisions. As robo advisors are algorithm based and act based on data, they’re free from most of these biases. However, the belief that robo advisors are fully rational is not true. An RA algorithm can be biased if it is developed using a dataset that is biased or if it gets feedback that is biased. For example, an RA algorithm can suffer from home bias, if it is trained on a dataset that is biased against international assets. It may only include domestic ETFs in the investor's portfolio — missing out on the benefits of international diversification. To prevent biases impacting RAs, use diversified, reliable, and accurate data for creating the underlying algorithm.
We recommend a hybrid model
Many investors are still not comfortable with the idea of an algorithm managing their money. They might like the support of a human advisor, who can guide them in their investments. Hence, we recommend that investment advisors should try a hybrid approach, in which robo advisors can be used to augment the capabilities of a human advisor. Tasks such as portfolio construction and rebalancing can be done through algorithms, whereas human advisors can act as ‘investment coaches’ and take up tasks such as answering investor questions and coaching for the behavioral changes needed to maintain discipline when making investments.
Vanguard in its personal advisor service uses a mix of human and robo advisor in the below way:
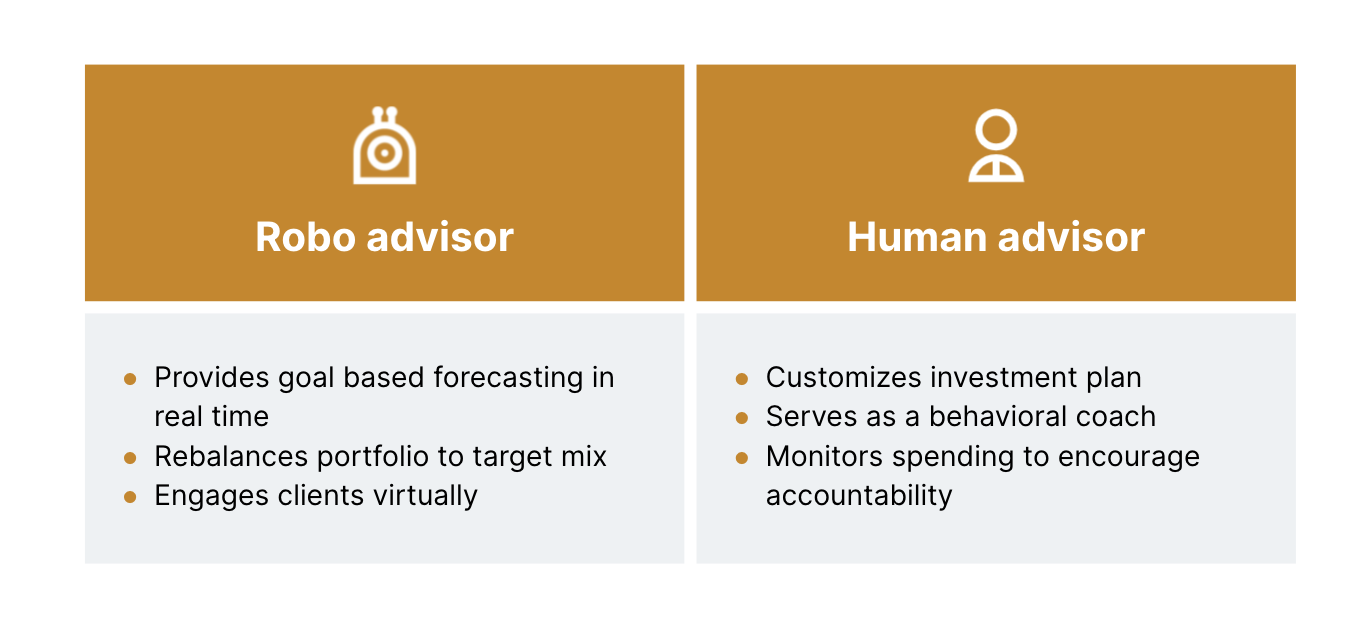
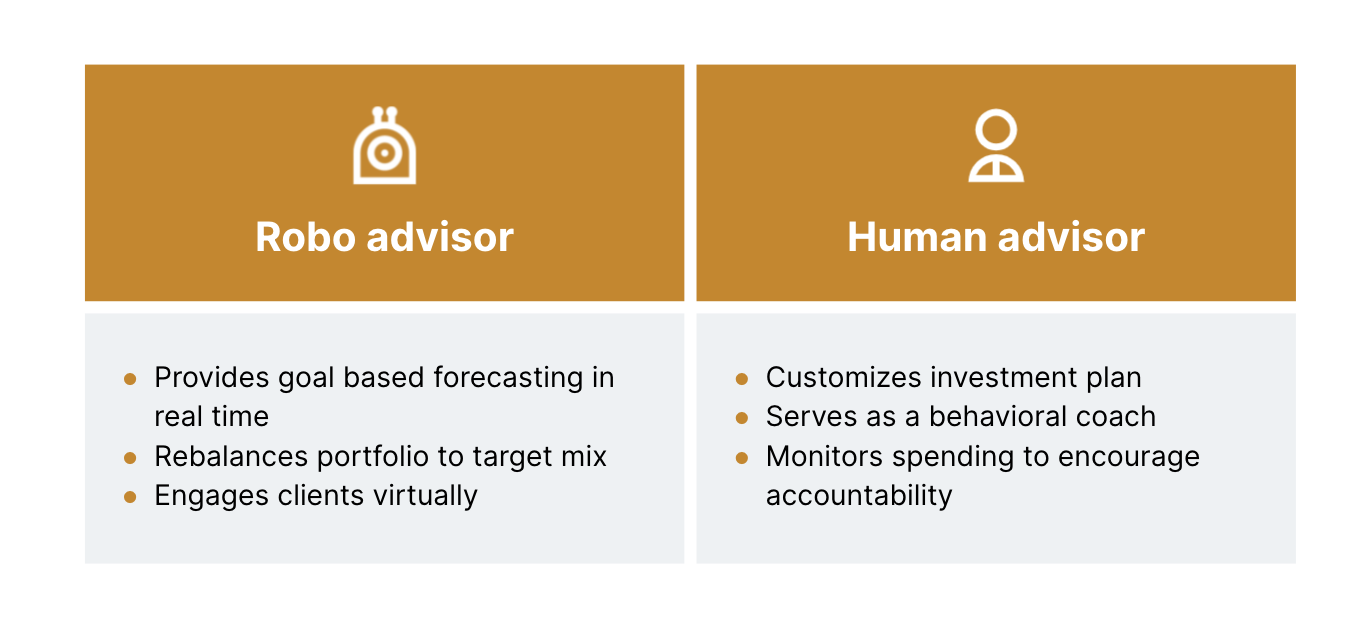
Source: Vanguard Group (as mentioned in a HBR article)
Below is a robo advisors maturity curve that shows key considerations at each maturity stage for a robo advisor:
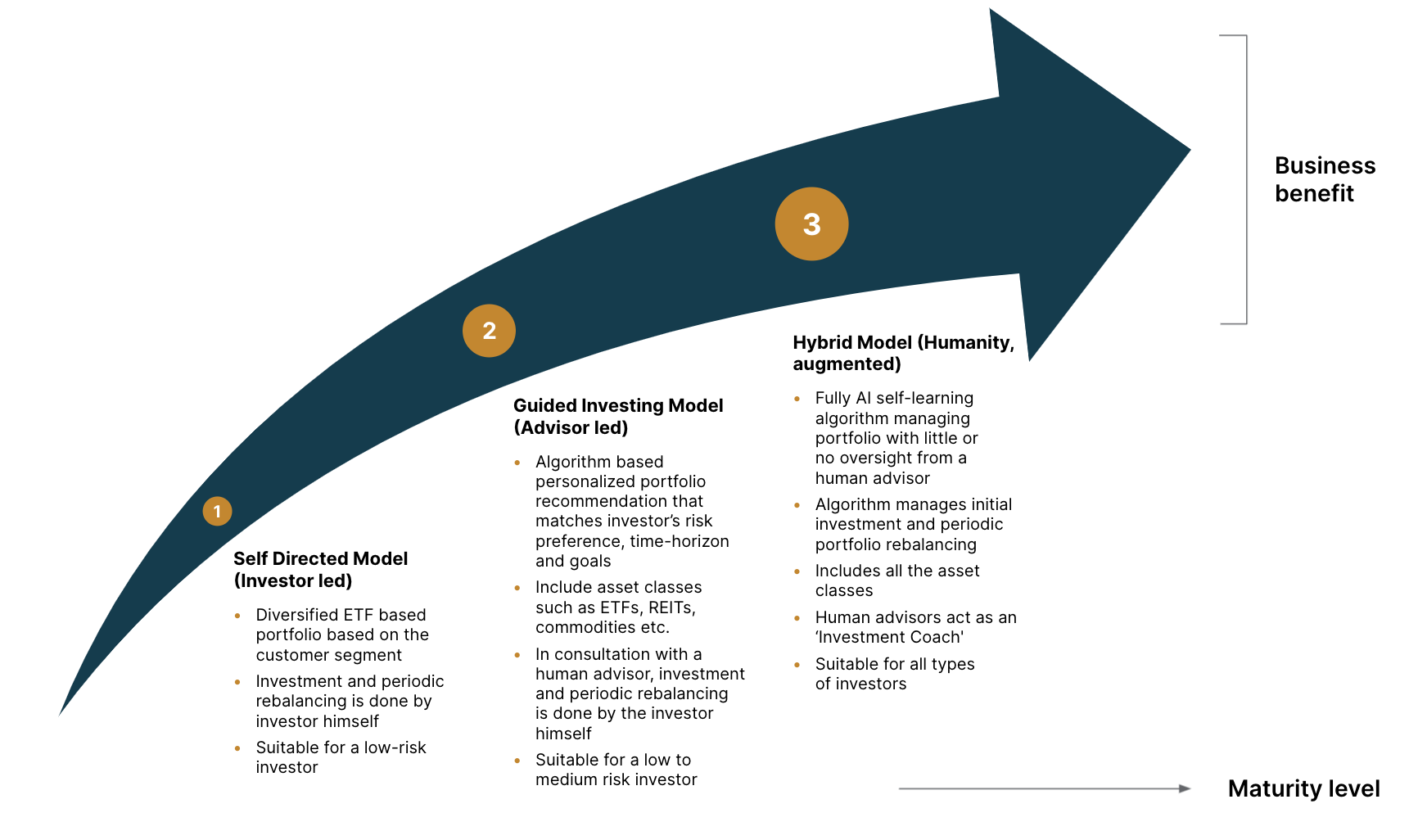
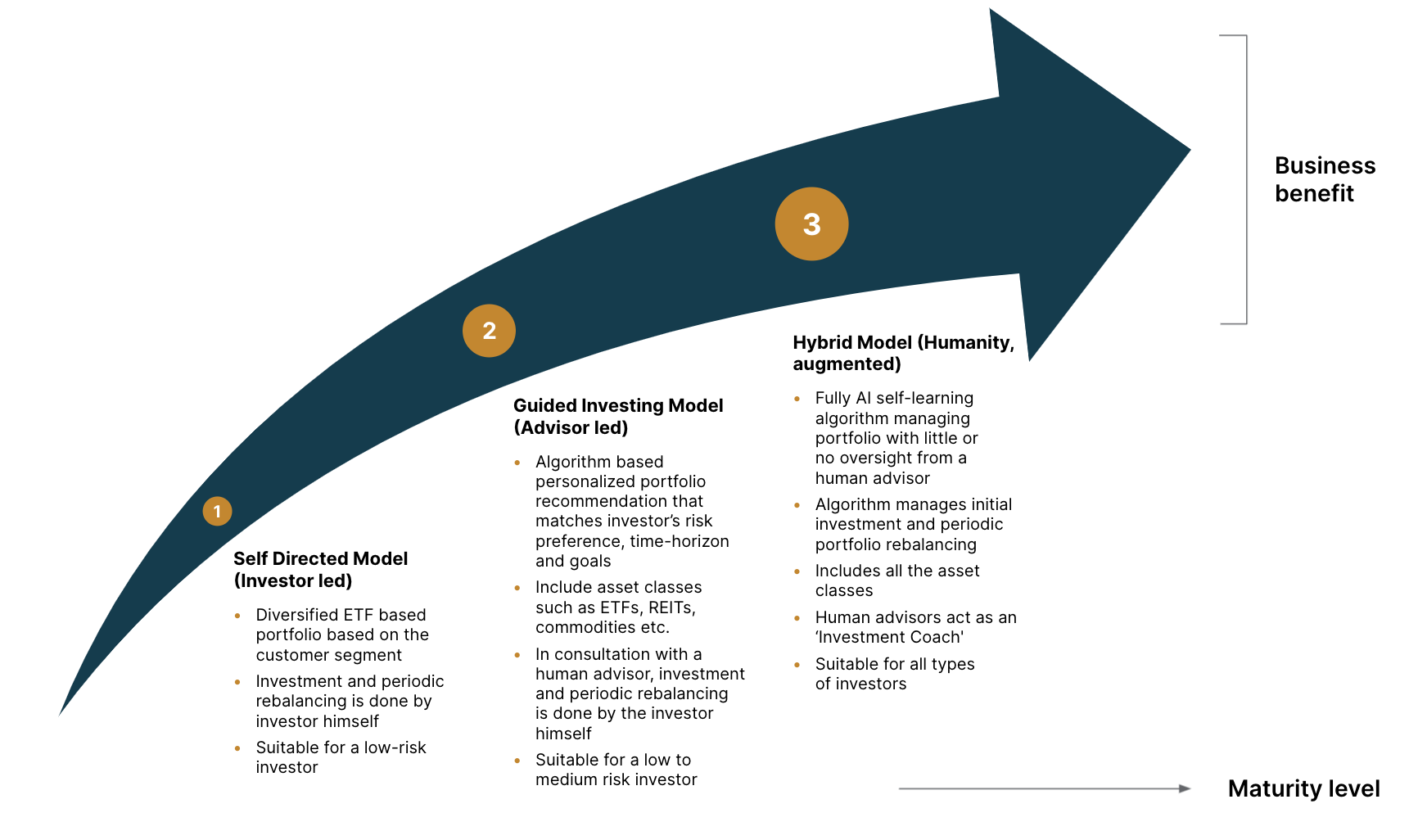
Smart compliance checks
A critical area for a wealth management firm is meeting compliance requirements, such as those related to Anti Money Laundering (AML) and Know Your Customer (KYC). ‘RegTech’ as it is referred to is a bouquet of technologies and solutions that can be used for meeting the regulatory needs.
AML and KYC are intended to prevent criminal activities such as money laundering and terrorist financing. The checks are performed when a new account is opened as well as periodically afterwards. Money laundering presents a big reputational risk to wealth management firms. Legacy rule-based AML monitoring systems are not effective as they result in a high volume of false positive alerts, resulting in manual intervention and wasted efforts. Money launderers are now using sophisticated methods to avoid detection and thus regulators are demanding robust AML systems from financial institutions.
Solutions for KYC and AML:
To improve the efficacy of AML and KYC systems and processes, the following advanced analytics techniques can be used:
Create a single customer view: Using data from internal and external sources coupled with techniques such as record linking and deduplication, a single holistic profile of a customer can be created. For example, a customer might be investing directly or through an advisor and might also be investing via the workplace investing route. Data from different business units can be combined with external data sources, such as the government's sanctions list, to create a single customer view. The fuller, richer and consistent customer views can enable more streamlined KYC processes.
Creating links between disparate parties: Money launderers now use a complex web of money trail so that it is difficult to track the ultimate beneficiary. Advanced statistical techniques of network analysis can be used to find relationships between customers, businesses, accounts and transactions. The established links can be used to uncover beneficial owners and detect financial crime. These interconnected entities can also be displayed graphically as depicted below.
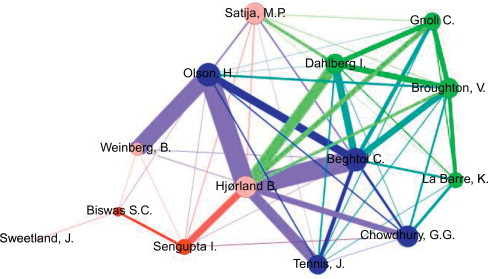
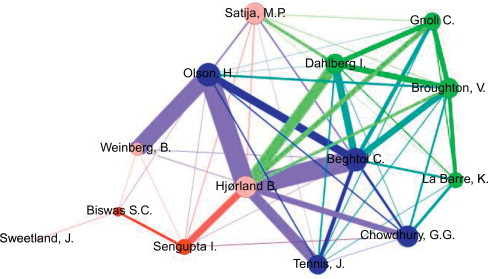
Source: Science Direct
Maximizing active returns
Another core business vertical in which artificial intelligence can have an impact is active investment management. Due to challenging market conditions, beating a benchmark’s return is becoming difficult. Now, many firms are making investment decisions that are driven in part by insights discovered using AI. Below are few ways in which artificial intelligence can be used for extracting useful information from unstructured data — which can in turn be used to maximize the performance of a portfolio relative to its benchmark.
Sentiment indicator for gauging market trend
Wealth management advisors deal with tons of unstructured data. This includes broker reports, company’s annual and quarter statements and analyst calls. It is difficult to manually go through all the data and determine the sentiment around the future prospects of a sector or security. Advanced natural language processing (NLP) techniques for voice and text recognition can be used to generate sentiment from these different sources of data and develop a view about the security’s forward returns. The sentiments can also be rolled up to sector sentiment heat maps.
Sector sentiment heat maps
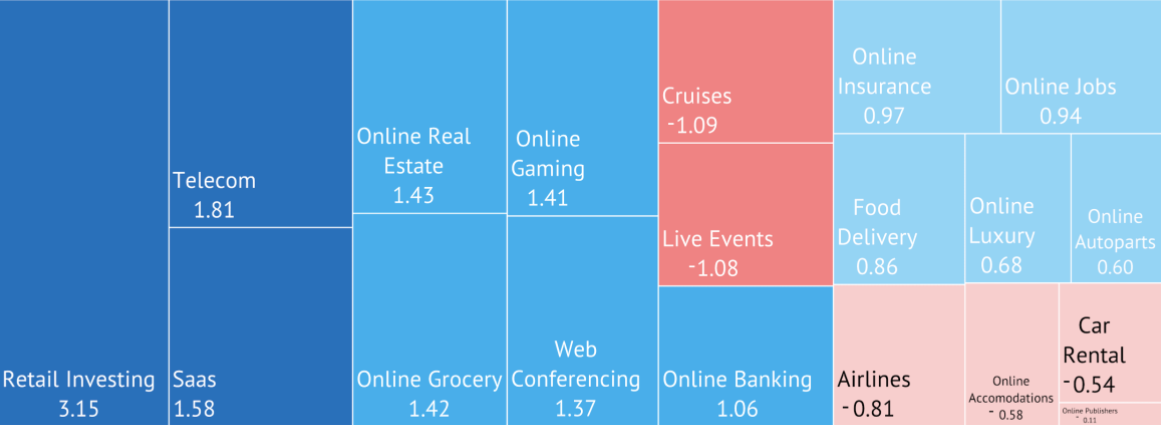
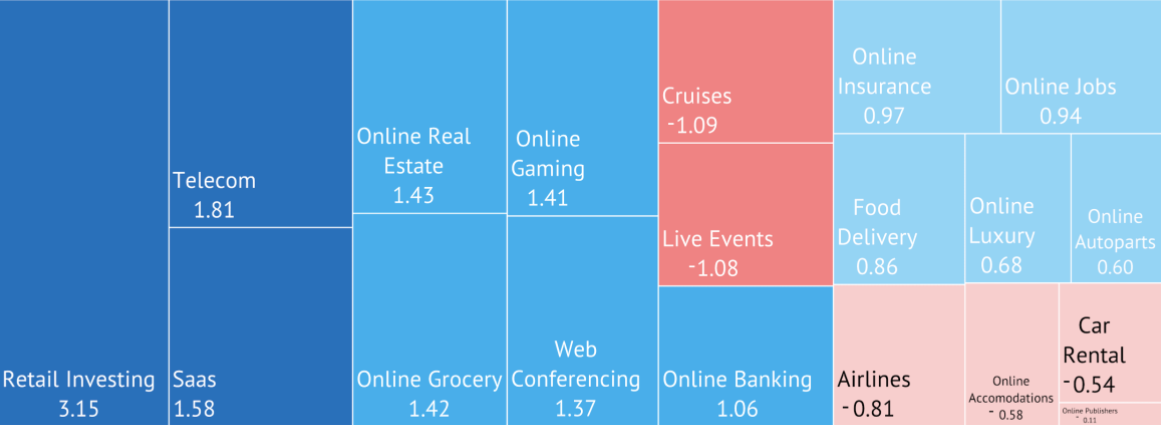
Source: Knoema (SimilarWeb)
The CFA Institute, in its publication mentions that the US-based American Century Investments uses sentiment analysis on companies’ quarterly management conference calls. Its model analyzes four components: omission (failure to disclose key details), spin (exaggeration from management and overly scripted language), obfuscation (overly complicated storytelling), and blame (deflection of responsibility).
AI-driven insights for investment decisions
Deep learning approaches such as artificial neural networks can be used to predict security-forward returns. Apart from traditional data sources such as security's historic returns and macroeconomic variables such as GDP growth forecast, these techniques can consider alternate data sources such as high resolution satellite images. For example, US based RS Metrics can determine revenue potential of a solar solution provider by analyzing satellite images of the project sites and by determining percentage completion of its projects.
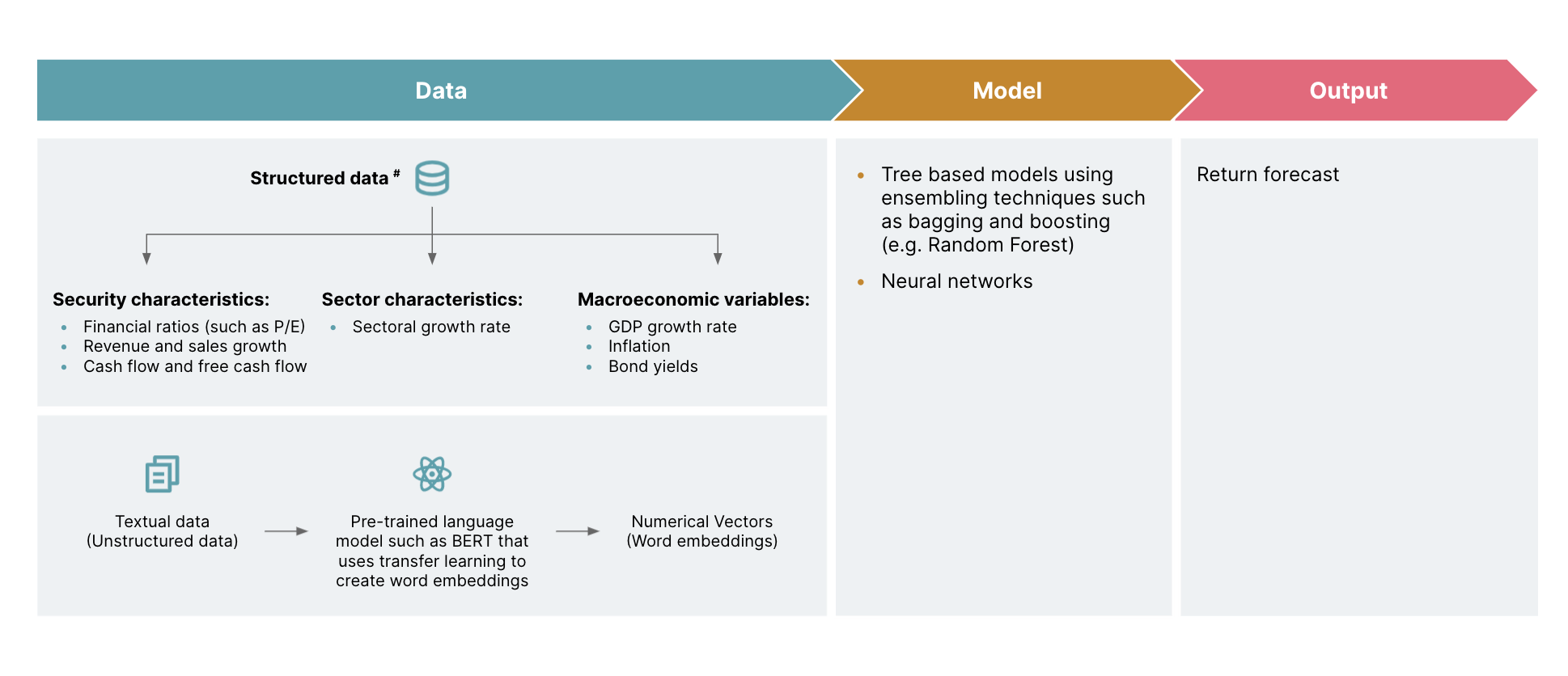
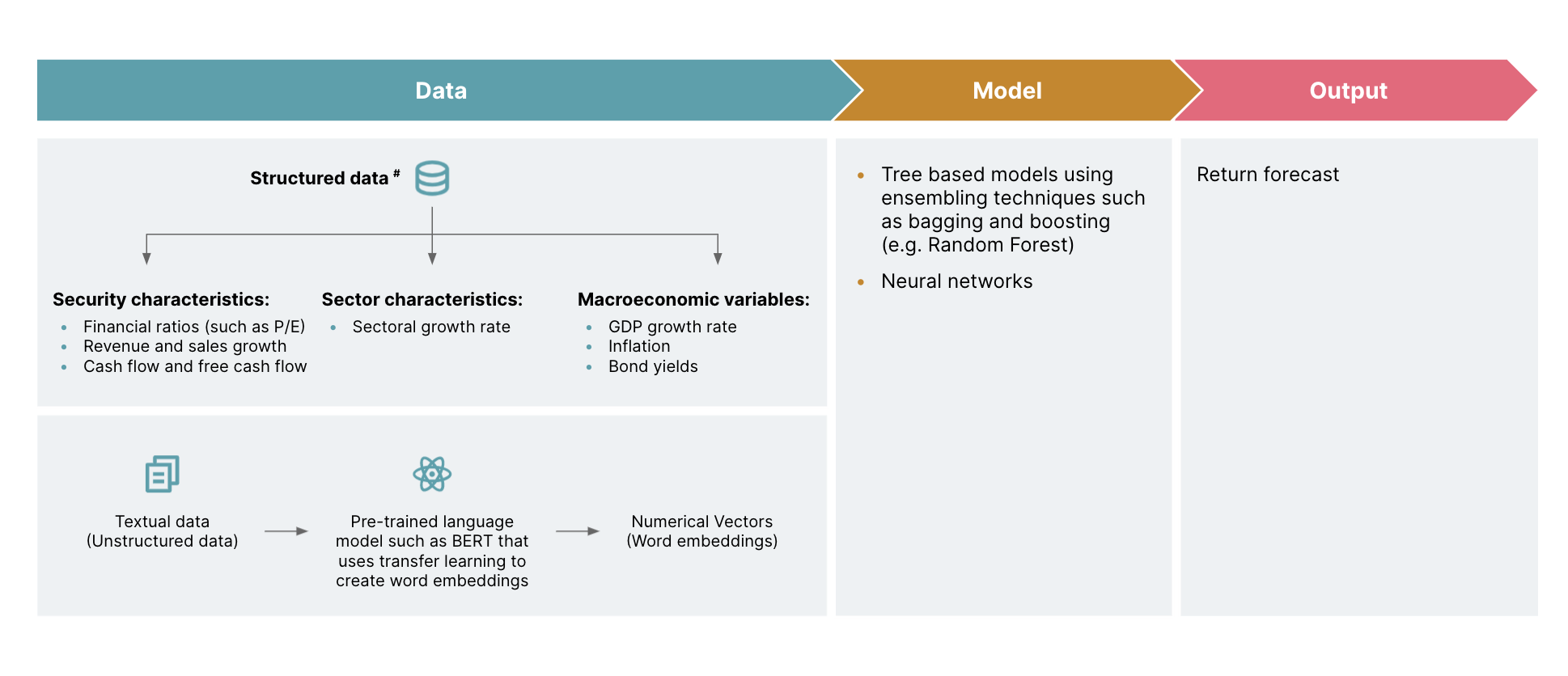
# An indicative list of data points. Data that is useful for prediction will be determined through ‘Feature Selection’
Usage of these models are also gaining traction in fixed-income investments. For example, as per a CFA publication, China’s biggest AMC, China Life Asset Management Company applies statistical models such as logistic regression on credit risk data, third-party credit ratings data, and publicly available information such as a company’s annual reports, financial statements, regulatory filings news etc. for determining likelihood of default on a particular issue.
The future and way ahead
The adoption of artificial intelligence in wealth management is an emerging trend. Despite various challenges — such as availability of quality and reliable data, and scarcity of talent for building AI solutions — innovations are happening at a breakneck pace. Increasingly, machines are playing an important role in augmenting the capabilities of portfolio managers, back-end operations and investment advisors. Some of the trends that will shape the future of the WM industry are:
Dynamic portfolio optimization using Reinforcement Learning
Due to the advances in technology, we anticipate that the wealth management industry will move beyond goal-based investing and towards guiding investors on the path of financial independence through wealth creation. As more and more people seek wealth management services to create long-term wealth, solving the problem at scale will become a huge challenge. We expect the emerging field of reinforcement learning — which has been used to develop machines that can outperform humans at video games — can play a key role in solving the problem. Research has shown that reinforcement learning can be used to dynamically optimize portfolio wealth over long time horizons.
Emotional intelligence powered robo advisors
AI technologies will soon start recognizing, interpreting and responding to human emotions. This branch of AI, known as affective computing, will help robo advisors to connect with investors at a much deeper and personal level.
In our previous article on Conversational AI in Capital Markets, we provided a roadmap on building compelling AI applications (see section ‘A staged approach to launching conversational AI’). This is an effective mechanism to build strong AI systems and will set you on your journey of building intelligent applications. We believe that in the coming decade, with advances in big data technologies, increasing computational powers and powerful research will help to develop advanced solutions that will completely change the way people plan and make their investments.