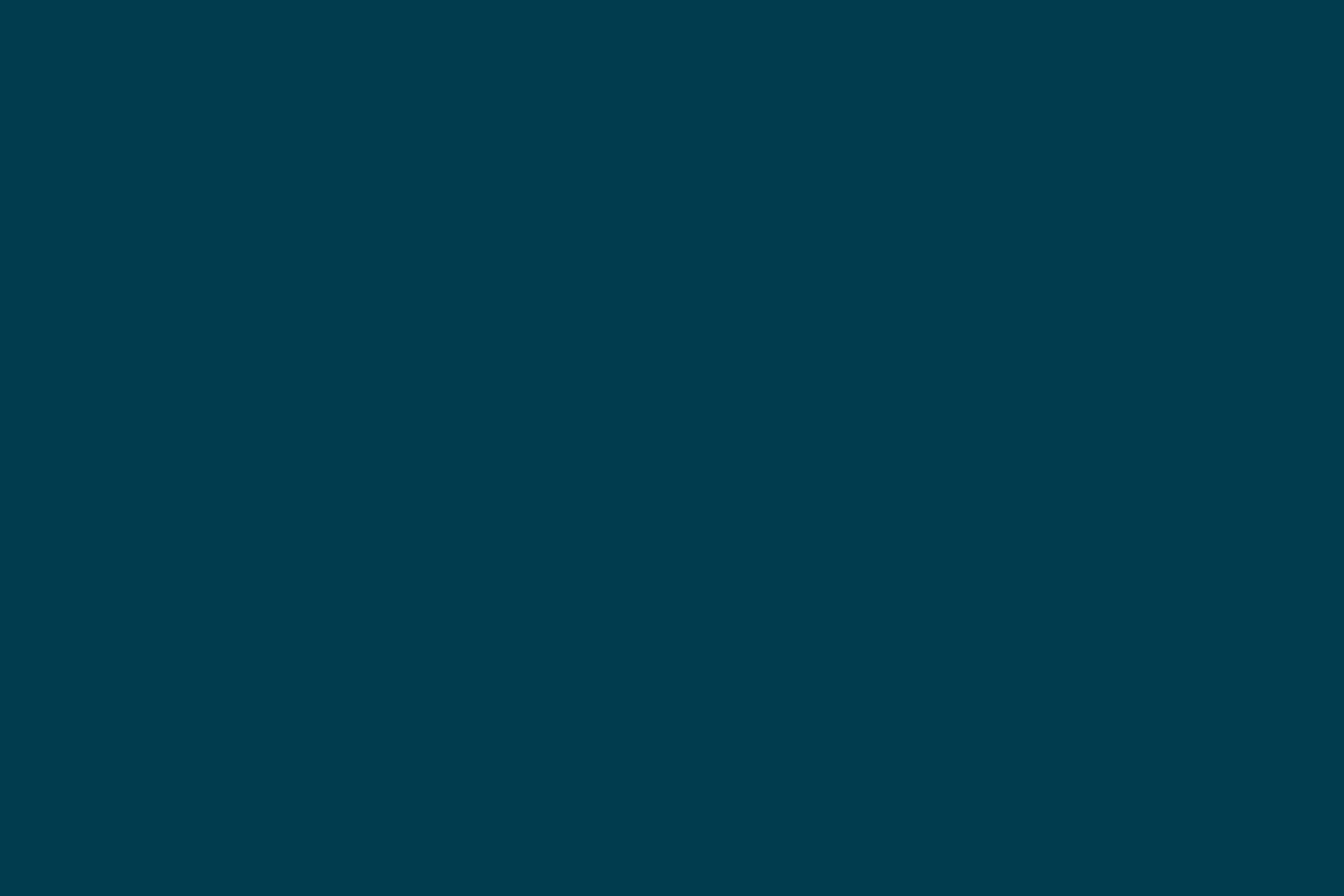
Will AI ever win a Nobel Prize?
Published: June 04, 2021
In the 17th century, Sir Isaac Newton formulated the three-body problem – How do three objects orbit one another under their gravity? For centuries, this problem has remained unsolved until a couple of years ago, when AI solved it.
“So, who solved the problem? Did the algorithm solve the problem? Or did the people who wrote the program solve it?,” asks Dr. Williams, Distinguished Research Professor and Core Member, AAII - Australian Artificial Intelligence Institute.
This is one of the many questions and promises we explored about artificial intelligence (AI) and scientific discoveries in the third edition of Thoughtworks’ Engineering for Research Symposium, held in October 2020.
“What's different about the boom today is that some of the biggest businesses on the planet use AI as their core technology, for example to sell advertising,” says Dr. Ross D. King, Turing Fellow, The Alan Turing Institute, UK, and one of the most experienced AI researchers in Europe.
He also adds, while AI is ‘pretty generic’ and can be repurposed to solve any imaginable problem, the most rampant use of the tech is in solving narrow and deep problems – not the broad ones.
For instance, when leveraged for scientific research, AI makes tactical decisions while the strategic decisions are still made by humans. The assumption is that AI cannot (yet) deeply understand and reflect on the world.
Another example is how AI ‘reads’ books. The tech can extract information but can’t get a keen sense of the world the way humans can. This is because AI can process data and information to build knowledge but lacks ‘understanding.’
And, this limited ability to decide what is interesting and what is not is crucial to making scientific breakthroughs.
The above discussed developments have required one to reimagine technology solutions, and/or change the way we believed something or some principle worked. So, it begs the question, 'How do humans make scientific discoveries?'
The fact is much of today’s scientific discovery is attributed to ‘human intuition’.
Scientists have consistently been trying to make computers represent knowledge in a way that they could learn from, and reason with. Today, this effort involves combining knowledge representation, reasoning and learning that combines various schools of thought (i.e. Connectionism – neural networks, Symbolism, Evolutionarism, Bayesian Heuristics, and Analogizing) from the AI space.
When it comes to the nature of scientific discovery, Prof. Sundar Sarukkai, Founder of Barefoot Philosophers, poses some very interesting questions around how machine intelligence can truly be creative without human-like characteristics such as weakness, ambition or drive – all of which have ‘helped, led or inspired’ humans to forge breakthroughs across scientific disciplines. He wonders if we should ‘divorce the psychological from the logic.’
Sarukkai discusses how dreams allow human minds to indulge in the meaningless – flying, travelling into the past or future, etc. He asks if such meaninglessness also contributes to discovery. And, if yes, then how would AI be able to aspire to such tactics or even make mistakes that sometimes turn into path breaking scientific discoveries. He also emphasizes the importance of social interactions for scientists and how one might be able to replicate this with AI.
In the face of such questions, Dr. Kitano, President, Systems Biology Institute, and CEO, Sony AI Inc., interestingly believes that by 2050, AI could perform discovery in a manner that’s completely different from the way humans do it today. “An alternative form of scientific discovery,” he believes will bring about the anticipated paradigm shift.
DL works well when its neural networks are fed with a lot of data, and a lot of compute power to process all of that data. For example, a DL network for image classification will need to be fed millions of images with appropriate labeling, and advanced computer hardware to process the voluminous feed.
Human intelligence does not work this way. ”Kids don't need to see millions of pictures of kittens of all sorts from different angles to recognize one because there's an inbuilt prior knowledge in the system,” Dr. King says.
If AI needs to make a truly path-breaking scientific discovery, it needs to absorb prior knowledge. And, humans have yet to come up with a simpler way to do this than the data-heavy neural networks.
This is an especially pressing concern in areas where there is a scarcity of data, like in most drug research, for instance. “If you look at a biomedical research lab, equipment is not connected. Before we think of AI, we need to connect the lab, get all of that data etc. We’re not there yet,” says Dr. Kitano.
However, there is tangible excitement in another data-heavy field - astronomical research. AI has been able to find patterns that are beyond a human’s capacity to perceive.
Just like how telescopes help us view something from extremely far away or infrared sensors help us capture data about rays that cannot be sensed by humans, AI has been able to discover patterns and anomalies that humans haven’t even considered.
“This data can support new theories, helping us make sense of the universe,” says Dr. Williams, “Or disprove an existing theory, which is science too.”
AI can solve problems efficiently, more economically and quicker. For instance, pharmaceutical companies don't invest in the research of many diseases because of the significantly low return on such investments. But, if AI can make it cheaper, “a particular treatment for a particular genotype of patients in their particular condition is probability,” he imagines.
But, given AI’s progress and the way it’s pervading scientific research, we could be looking at a future where AI could autonomously make a Nobel-worthy scientific discovery. If it could successfully solve Newton’s centuries-old three-body problem in a matter of seconds, the possibilities for a better world with AI are endless.
To get there, we need to build AI tools that we trust. As Dr. Williams says, “humans work with a lot of technology that doesn’t explain anything. When one is riding a bike and falls off, no one thinks the bicycle is plotting their downfall. We understand it. And, this should happen with AI as well. Because trust is so critically important.”
This article is an excerpt from a series of talks given by Dr. King, Dr. Kitano, Prof. Sarrukai and Dr. Williams at the Engineering for Research Symposium, hosted by Thoughtworks in October 2020. You can watch each of their talks here.
“So, who solved the problem? Did the algorithm solve the problem? Or did the people who wrote the program solve it?,” asks Dr. Williams, Distinguished Research Professor and Core Member, AAII - Australian Artificial Intelligence Institute.
This is one of the many questions and promises we explored about artificial intelligence (AI) and scientific discoveries in the third edition of Thoughtworks’ Engineering for Research Symposium, held in October 2020.
Going past the booms and busts
AI is half a century old with a history riddled with booms and busts. The last boom was perhaps in the 1980s, which laid the foundation for the emerging tech’s impact and its rapid evolution.“What's different about the boom today is that some of the biggest businesses on the planet use AI as their core technology, for example to sell advertising,” says Dr. Ross D. King, Turing Fellow, The Alan Turing Institute, UK, and one of the most experienced AI researchers in Europe.
He also adds, while AI is ‘pretty generic’ and can be repurposed to solve any imaginable problem, the most rampant use of the tech is in solving narrow and deep problems – not the broad ones.
For instance, when leveraged for scientific research, AI makes tactical decisions while the strategic decisions are still made by humans. The assumption is that AI cannot (yet) deeply understand and reflect on the world.
Another example is how AI ‘reads’ books. The tech can extract information but can’t get a keen sense of the world the way humans can. This is because AI can process data and information to build knowledge but lacks ‘understanding.’
And, this limited ability to decide what is interesting and what is not is crucial to making scientific breakthroughs.
Powering the paradigm shift
As Dr. Williams points out, the Nobel Prize is awarded for ‘breakthroughs’, not inventions. Or, as Dr. King puts it, the prize is given for ‘changing the representation of the problem’ in some way, as Einstein did with the theory of relativity. Today, AI isn’t mature enough to make that paradigm shift.The above discussed developments have required one to reimagine technology solutions, and/or change the way we believed something or some principle worked. So, it begs the question, 'How do humans make scientific discoveries?'
The fact is much of today’s scientific discovery is attributed to ‘human intuition’.
Scientists have consistently been trying to make computers represent knowledge in a way that they could learn from, and reason with. Today, this effort involves combining knowledge representation, reasoning and learning that combines various schools of thought (i.e. Connectionism – neural networks, Symbolism, Evolutionarism, Bayesian Heuristics, and Analogizing) from the AI space.
When it comes to the nature of scientific discovery, Prof. Sundar Sarukkai, Founder of Barefoot Philosophers, poses some very interesting questions around how machine intelligence can truly be creative without human-like characteristics such as weakness, ambition or drive – all of which have ‘helped, led or inspired’ humans to forge breakthroughs across scientific disciplines. He wonders if we should ‘divorce the psychological from the logic.’
Sarukkai discusses how dreams allow human minds to indulge in the meaningless – flying, travelling into the past or future, etc. He asks if such meaninglessness also contributes to discovery. And, if yes, then how would AI be able to aspire to such tactics or even make mistakes that sometimes turn into path breaking scientific discoveries. He also emphasizes the importance of social interactions for scientists and how one might be able to replicate this with AI.
In the face of such questions, Dr. Kitano, President, Systems Biology Institute, and CEO, Sony AI Inc., interestingly believes that by 2050, AI could perform discovery in a manner that’s completely different from the way humans do it today. “An alternative form of scientific discovery,” he believes will bring about the anticipated paradigm shift.
Too much data; too little data
Data-driven Machine Learning has been driving the most recent AI boom, alongside the potential of approaches like Deep Learning (DL).DL works well when its neural networks are fed with a lot of data, and a lot of compute power to process all of that data. For example, a DL network for image classification will need to be fed millions of images with appropriate labeling, and advanced computer hardware to process the voluminous feed.
Human intelligence does not work this way. ”Kids don't need to see millions of pictures of kittens of all sorts from different angles to recognize one because there's an inbuilt prior knowledge in the system,” Dr. King says.
If AI needs to make a truly path-breaking scientific discovery, it needs to absorb prior knowledge. And, humans have yet to come up with a simpler way to do this than the data-heavy neural networks.
This is an especially pressing concern in areas where there is a scarcity of data, like in most drug research, for instance. “If you look at a biomedical research lab, equipment is not connected. Before we think of AI, we need to connect the lab, get all of that data etc. We’re not there yet,” says Dr. Kitano.
However, there is tangible excitement in another data-heavy field - astronomical research. AI has been able to find patterns that are beyond a human’s capacity to perceive.
Just like how telescopes help us view something from extremely far away or infrared sensors help us capture data about rays that cannot be sensed by humans, AI has been able to discover patterns and anomalies that humans haven’t even considered.
“This data can support new theories, helping us make sense of the universe,” says Dr. Williams, “Or disprove an existing theory, which is science too.”
Bringing possibilities to life
“Today, in many parts of the world, the average person lives a much better life than a king did a thousand years ago. If we want everyone to live like that, we need even better science and technology. I think the only way to achieve that is through AI to make science more efficient and robust,” says Dr. King.AI can solve problems efficiently, more economically and quicker. For instance, pharmaceutical companies don't invest in the research of many diseases because of the significantly low return on such investments. But, if AI can make it cheaper, “a particular treatment for a particular genotype of patients in their particular condition is probability,” he imagines.
So, will AI win a Nobel Prize?
The most likely answer is no — to win a Nobel, the most basic requirement is ‘one must be alive.’But, given AI’s progress and the way it’s pervading scientific research, we could be looking at a future where AI could autonomously make a Nobel-worthy scientific discovery. If it could successfully solve Newton’s centuries-old three-body problem in a matter of seconds, the possibilities for a better world with AI are endless.
To get there, we need to build AI tools that we trust. As Dr. Williams says, “humans work with a lot of technology that doesn’t explain anything. When one is riding a bike and falls off, no one thinks the bicycle is plotting their downfall. We understand it. And, this should happen with AI as well. Because trust is so critically important.”
This article is an excerpt from a series of talks given by Dr. King, Dr. Kitano, Prof. Sarrukai and Dr. Williams at the Engineering for Research Symposium, hosted by Thoughtworks in October 2020. You can watch each of their talks here.
Disclaimer: The statements and opinions expressed in this article are those of the author(s) and do not necessarily reflect the positions of Thoughtworks.