Looking Glass 2025
Operationalizing AI for
business impact
The mainstreaming of AI — and generative AI in particular — is continuing apace. But as AI proliferates, it’s more evident that successfully operationalizing AI models and bringing them to production remains a challenge. From questionable output to unintended consequences, there are a host of real and projected scenarios that prevent organizations from leveraging AI to its full potential.
Enterprises continue to struggle with data quality, data accessibility and the challenges of data at scale, all of which remain foundational to robust, effective AI. As our data platform lens explores, careful data curation, and effective data engineering and architecture are essential. The importance of synthetic data, particularly in research contexts, as a tool to avoid privacy and data integrity issues is also becoming more and more apparent.
Organizations also need to develop better approaches to the evaluation and control of AI systems. Forward-looking enterprises are adopting ‘evals’ — tests of AI output to determine reliability, accuracy and relevance — and guardrails, programmed policy layers that mitigate the inherent unpredictability of generative systems.
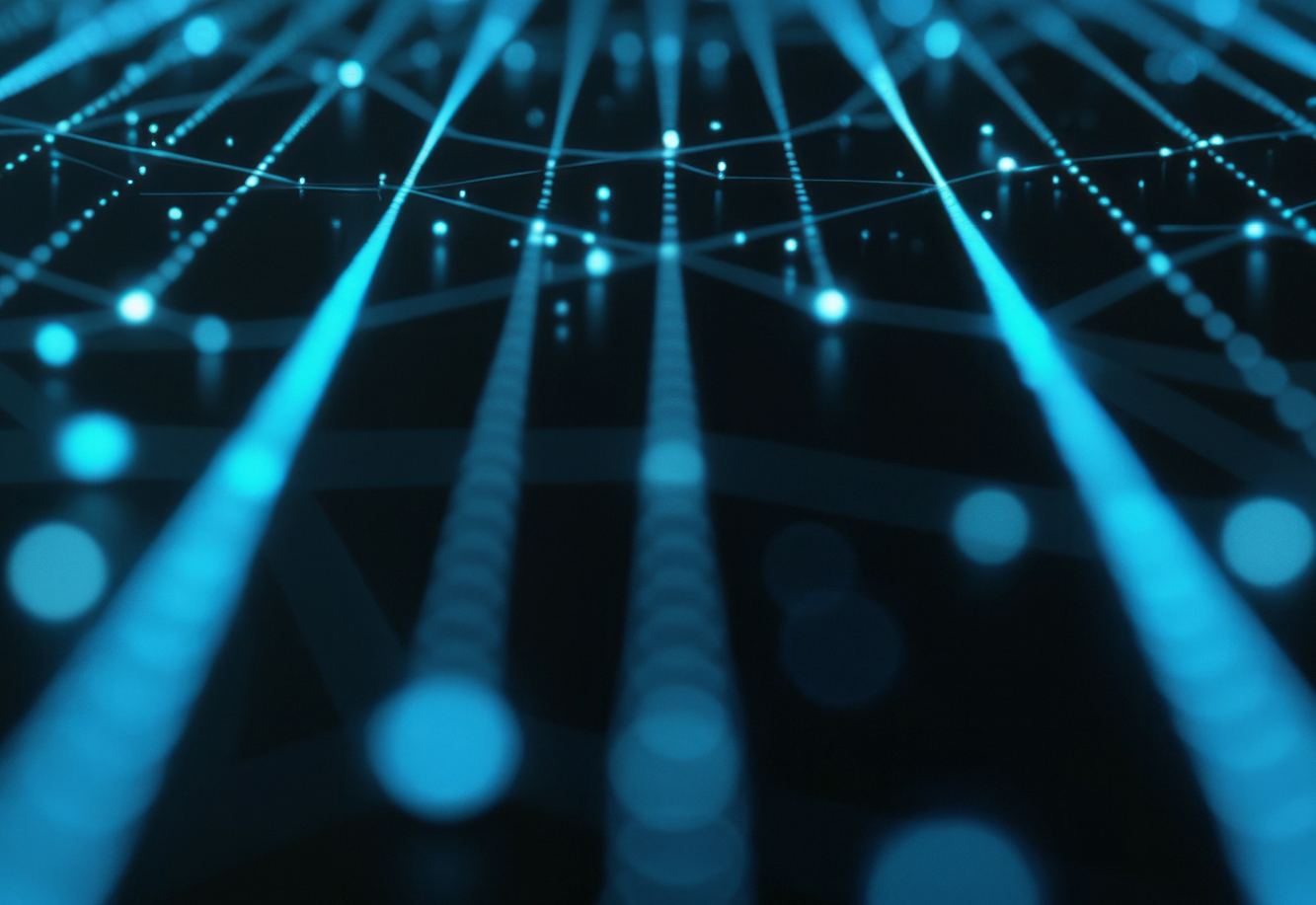
As adoption increases, improving the mechanisms through which AI systems are connected with enterprise applications grows more important. Proxy services are emerging to help developers link AI models with the applications they build.
AI agents are sometimes positioned as the next step in the evolution of AI, due to their capacity to mimic human reasoning. However, the technology remains relatively new, and finding applications for agents requires domain expertise, as well as the ability to precisely map and model complex processes and interactions. To build a sustainable and productive AI practice, it’s vital that the organization doesn’t resort to shortcuts, acquires the requisite skills and keeps innovation rooted in business realities.
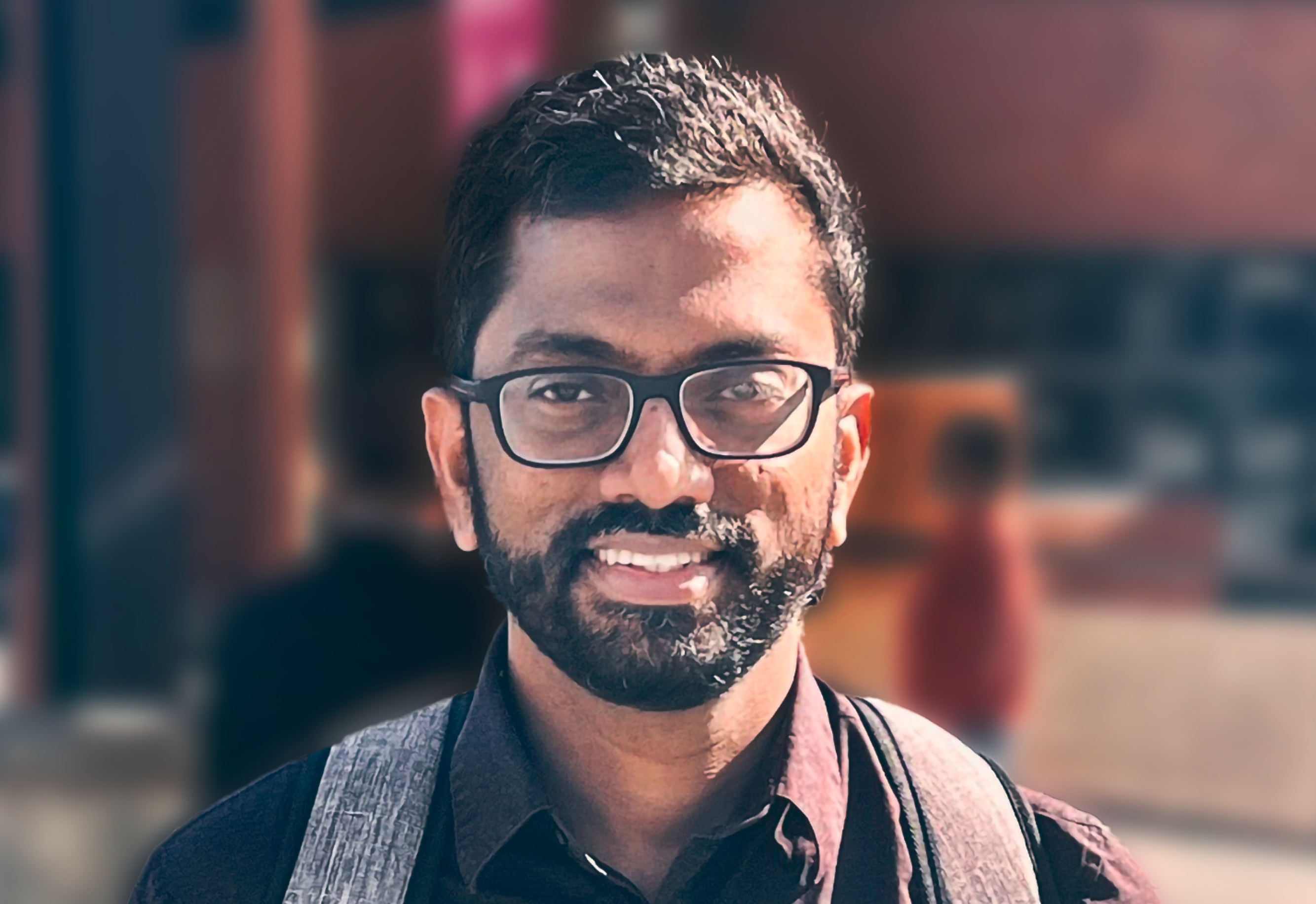
The lessons from automation endeavors in the ‘80s could help to build the right level of human-AI agent handovers. We must focus on augmenting humans rather than trying to substitute their current tasks completely.
The lessons from automation endeavors in the ‘80s could help to build the right level of human-AI agent handovers. We must focus on augmenting humans rather than trying to substitute their current tasks completely.
Signals
- The emergence of small language models, such as Microsoft’s phi-3, and AMD’s AMD 135. These make it possible to run AI models at the edge of networks on devices like mobile phones, and because they are relatively lightweight, focused and efficient, have a range of positive business, security and sustainability implications. LLMs also continue to evolve, with Anthropic’s Claude 3.5 Sonnet LLM, which has set industry benchmarks in terms of performance, recently upgraded to include computer use capabilities.
- Research showing that for many organizations, AI investments and adoption aren’t necessarily translating into deployment or business impact. While interest in (and spending on) AI solutions remains high, businesses are beginning to pay more attention to the cost of AI projects, and stepping up efforts to ensure they deliver value.
- The coming into force of the European Union’s AI Act, which sets an international benchmark by laying out obligations around data governance, documentation, human oversight and security for businesses adopting AI systems.
- Sustained, massive investment in data centers, with Google even turning to nuclear power to generate the vast amounts of power its AI offerings are likely to require. This indicates AI is a long-term bet that will continue to gain momentum in the business context, and in society as a whole.
- The growth of tools simplifying how engineers and others interface with AI models, such as LiteLLM and Langchain.
- Renewed focus on tackling LLM ‘hallucinations’ and fabrications, with novel techniques like ‘semantic entropy’ being applied to root out errors, and LLMs policing the output of other LLMs.
- Rising awareness of ‘shadow AI,’ or the use of unsanctioned AI tools in the enterprise context, which could pose significant problems for companies if sensitive information is leaked to LLMs by employees. In one recent survey a third of organizations admitted to finding it hard to monitor the illicit use of AI among their teams.
Trends to watch
Adopt
-
AI-ready data is data that has been structured and organized in a way that makes it easy for it to be integrated with AI systems. It has a number of specific qualities: high-quality (auditable and verifiable), consistent across different platforms and robust, comprehensive metadata.
-
More granular access controls for data, such as policy-based (PBAC) or attribute-based (ABAC) that can apply more contextual elements when deciding who has access to data.
Analyze
-
AI systems are notoriously opaque. Their complexity can make it very difficult to determine the relationship between inputs and outputs. AI observability is the broad practice of monitoring and analyzing an AI systems behaviors and performance to increase understanding and confidence that it is working as intended.
-
An emerging set of techniques to certify the provenance of data and to govern its use across an organization.
-
A new capability of generative AI tools to execute and automate computer-based tasks through natural language. They enhance digital workflows by enabling intuitive, conversational interactions with operating systems and applications. Examples include Claude's "computer use" feature and Auto-GPT, among others.
-
Technologies enabling the direct interaction of devices and information sharing between them, usually in an autonomous fashion. This enables to decision making and action with little or no human intervention.
-
Systems that monitor metrics across complex distributed systems and take corrective action if a problem is detected. They are often used for security, but increasingly also for resilience and recovery in the face of an outage.
-
An alternative to large language models (LLMs) that are more lightweight and efficient. While they aren't as powerful compared to their larger siblings, because they require less memory and computational power they can be used in devices at the edge of a network.
-
Talk to data (T2D) is a technology that allows users to interact with and analyze data using natural language queries as opposed to, say, the kinds of analytics and business intelligence dashboards that have become commonplace over the last two decades. It makes it easier to uncover insights and has a lower barrier to entry, giving more employees the ability to explore and ask questions about data.
Anticipate
-
These are attacks on (or using) machine learning systems. Attackers may tamper with training data or identify specific inputs that a model classifies poorly to deliberately create undesired outcomes.
-
A collective term for systems and devices that can recognize, interpret, process, simulate and respond to human emotions.
-
Bringing today's AI capabilities into robotics is bringing new levels of 'intelligence'. It can help robots better respond to situations and external stimuli and ostensibly make decisions about what actions to take in relation to its environment.
Adopt
Analyze
-
A digital representation of a person. The use of artificial intelligence allows the avatar to mimic the person it represents, thus making it ostensibly more convincing and life-like.
Anticipate
-
The concept of artificial general intelligence (AGI) refers to an AI system that possesses a broad range of capabilities across a range of intellectual tasks — it’s often compared to human-level intelligence. Debates about the threshold for AGI remain, and research into ways of achieving it continues and will play a part in wider discussions about AI and humanity.
The business opportunities for AI
By getting ahead of the curve on this lens, organizations can:
- Enhance knowledge management and transfer by adopting GenAI to help employees sift through, summarize and analyze stores of enterprise data, whether structured or unstructured. A wide range of products are emerging to facilitate the retrieval and dissemination of important information in industries like property.
- Harness AI to accelerate processes like legacy modernization and coding. Thoughtworks is already successfully applying GenAI to assist teams with one of the most difficult aspects of modernization: understanding and unpacking the intricate web of connections that typically underpin legacy systems and codebases. AI assistants can also significantly boost the productivity of software development and other teams by taking over frequent, repetitive tasks.
- Explore AI agents to elevate automation, potentially transforming how employees perform tasks like scheduling and customer support, and raising the bar for engagement and personalization in customer interactions.
- Boost the speed at which LLMs are brought into production, and their effectiveness when deployed through emerging practices and tools like LLMOps, which accelerate model development; retrieval-augmented generation (RAG), which can enhance models’ reliability; and AI gateways or smart endpoints to connect AI systems to applications.
- Develop and communicate a joined-up AI strategy that empowers employees to experiment with AI in a structured way, while preventing the emergence of ‘shadow AI’ that could pose a threat to the organization’s intellectual property or reputation.
- Leverage small language models to bring AI innovations to edge devices, offering opportunities for everything from operational analytics to personalization — without compromising privacy, since data doesn’t have to be moved to the center of a network.
- Lead the way in terms of compliance and ethical AI practices. We urge our clients not just to follow but embrace regulations like the EU AI Act, as such legislation often reflects wider societal sentiment and concerns — and potential customers take notice of businesses that are responding.
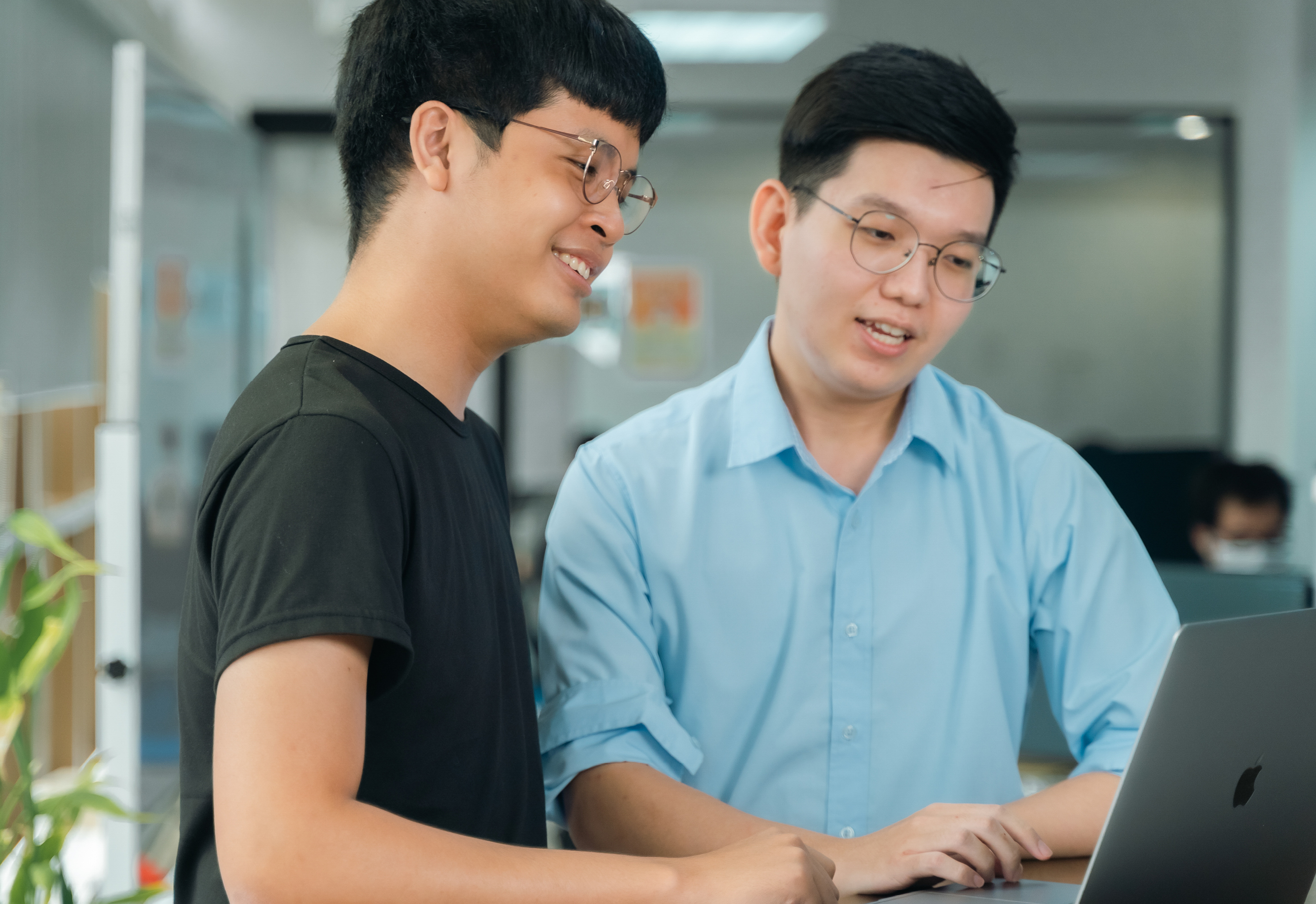
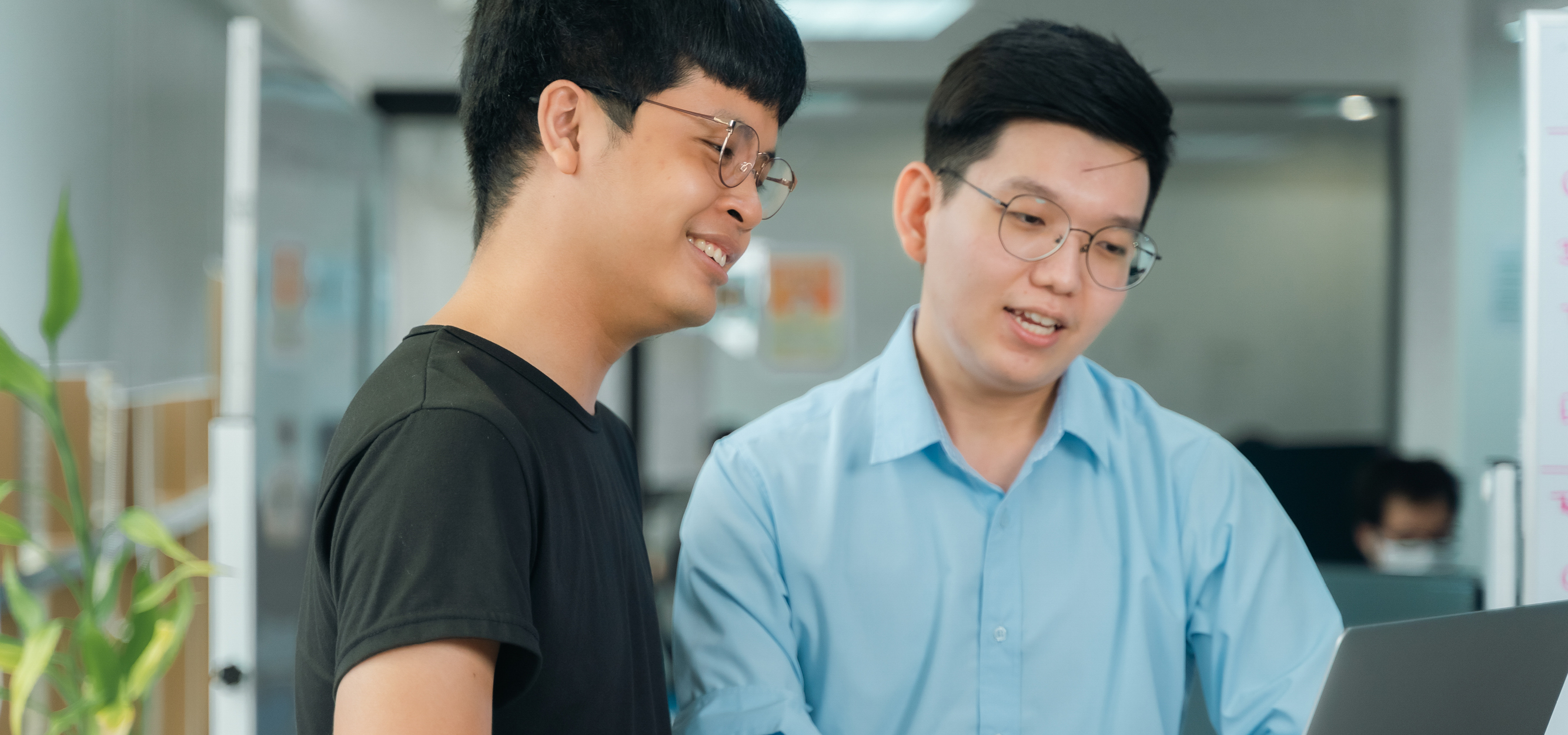
What we've done
PEXA
Thoughtworks partnered with digital property technology company PEXA, AWS and Redactive to develop an innovative and versatile AI assistant that has boosted the productivity of PEXA’s employees by providing personalized answers to queries and augmenting tasks like information discovery.
Seamlessly integrated with PEXA’s internal systems, the solution also met robust requirements for data security and privacy by equipping the assistant with permissions awareness, ensuring employees are only able to access information cleared for sharing.
Actionable advice
Things to do (Adopt)
- Identify AI champions who can help guide and teach your organization about the potential use cases for emerging solutions — but understand that AI can and will be applied in different ways in almost every part of the enterprise, which means these champions need to keep an open mind. Having people with a clear idea of what ‘good’ looks like can reduce risks and ensure AI initiatives focus on meaningful business results.
- Implement a holistic and comprehensive AI strategy for your organization that includes guidelines on permitted tools and the contexts in which AI can be used, to minimize the risks of shadow AI.
- Adopt retrieval-augmented generation (RAG) when developing AI systems, to give reliability an uplift and position models to create more specific outputs. Integrating evals and observability can further enhance the resilience of systems over the long term.
- Embed AI throughout the software development lifecycle. Maximum results are achieved when the role of AI isn’t just limited to coding, but assists with processes like testing and documentation.
- Apply data mesh and data product thinking to ensure AI applications are built on the robust data foundation needed to ensure they deliver business or customer value. Disciplines like data curation, which creates, organizes and manages data sets so they’re transparent and easily accessible, also contribute to the success of AI.
- Use proxies to simplify the way teams interact and leverage AI models, paving the way for the enhancement of applications they develop with AI features and capabilities.
Things to consider (Analyze)
- Avoid what’s known as the ‘substitution myth’ — the idea that AI can simply directly replace a human. Instead, build and implement systems that augment roles to make teams more productive and engaged, while acknowledging the continued importance of human judgement and oversight.
- Be cognizant of varied expectations around AI. Research suggests people may approach AI differently depending on cultural background, with some wanting a high degree of control and others prioritizing a sense of connection. These differences, as well as variances in context or situation, need to be understood and acknowledged when planning and implementing AI.
- Pay close attention to costs, and try to identify the approaches most likely to meet your needs while generating return on investment. Running AI models can be expensive, especially if expenses like employee compensation are factored in. Keeping spending in check requires active financial monitoring (i.e. FinOps) and consideration of things like small language models.
- Monitor AI regulation and future policy developments, particularly how these intersect with privacy laws, which could have a massive impact on the data resources available for AI projects. Multiple US states, and countries from Canada to India and Japan, are planning to enhance or roll out legislation that will set guardrails around AI use and development.
Things to watch for (Anticipate)
- Questions around legal liability and accountability for the negative consequences of AI use. As issues such as AI misleading customers and the associated legal challenges emerge, authorities like the EU are moving to make organizations more culpable.
- The potential growth of AI companions, designed to provide emotional support, friendship or even intimacy. While these could help combat loneliness and isolation, they may also have troubling implications for human interaction, requiring businesses to think carefully about the introduction of AI with companion-like features.