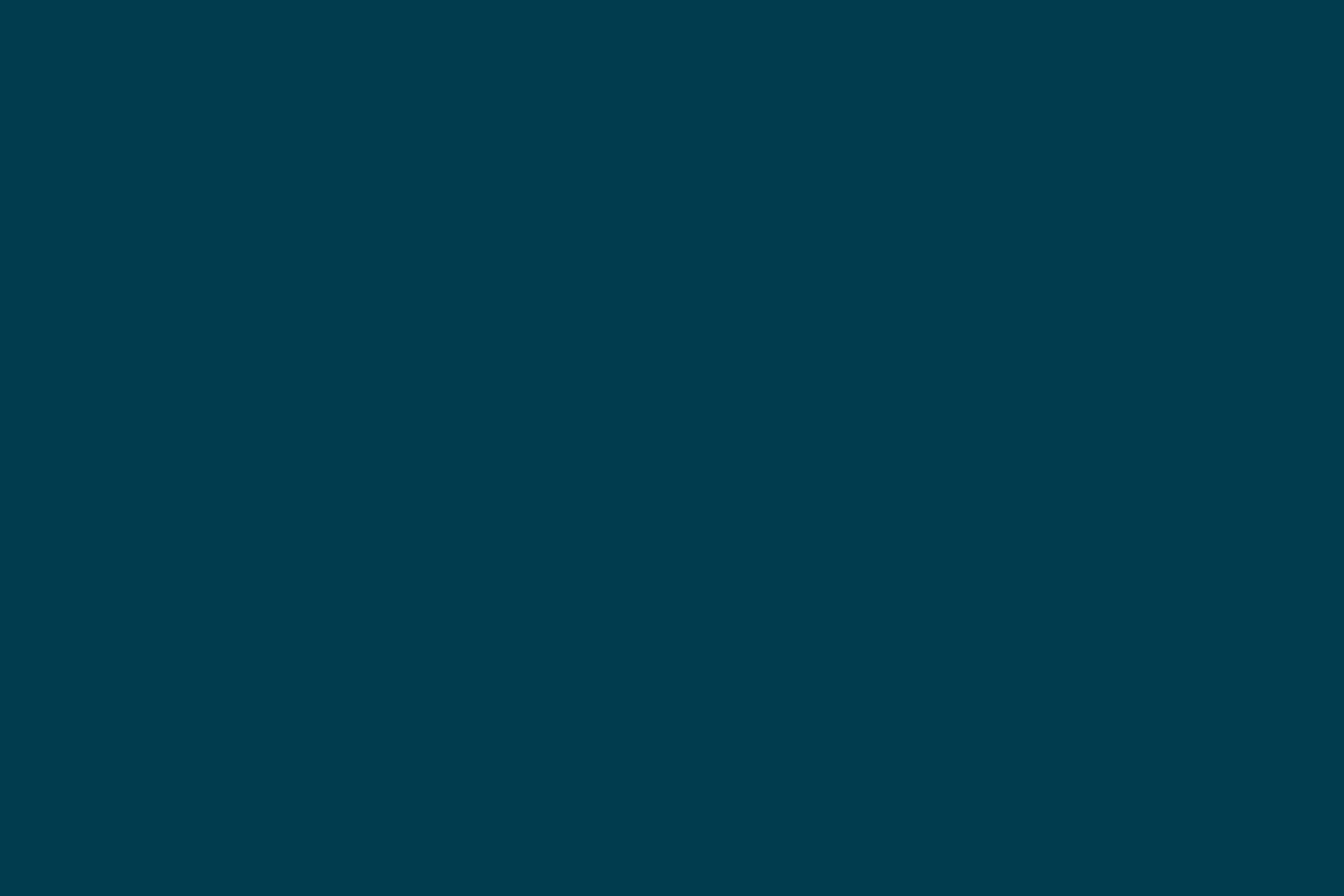
A Data Scientist's Advice to Business Schools
In my role at Thoughtworks, I assist enterprises by driving data-driven approaches into their operations, developing market-aware products that learn from data, and encouraging data-smart cultures among the c-suite of executives. I have had the privilege to work with many talented professionals looking to disrupt their industries while remaining true to their organization's values. As you might expect, many of these professionals hail from business schools that have prepared them to work with the variety of domain experts that exist within a company.
Today’s employers expect that MBA graduates possess an ability to strike a middle language between the priorities of a business and the deep domain knowledge of a company's experts. They should carry that 'generalist's touch' and be able to synthesize myriad high-level approaches into real-world utility for their organization.
To produce graduates like this a business school must find ways to teach the general high-level approaches used by domain experts across a company's departments. Graduates should have an understanding of how an expert's deep expertise in their field adds value to the overall strategic direction of the company. Only then can value-producing conversations and disruptive ideation exist between the business graduate and the domain expert.
Up until recently this has been straightforward in business schools since the traditional departments have fairly obvious overlaps with business operation. For example, an organization's marketing department contains domain experts in communicating the value of the company's product or service to its customers. Finance has experts in managing the financial functions of the business. Public relations have experts in maintaining the public view of the organization, while IT ensures systems, databases and software are operating as expected.
For many years, striking the middle language between these specialist concerns and a generalist's high-level understanding of them has allowed business schools to produce graduates who can help their employer answer to market demands.
But something is changing. The market isn't what it used to be. Virtually every industry is being touched by a new currency, and no business is immune. It's called data, and it is connecting people and process in new and disruptive ways.
Because of this, there is an undercurrent of concern flowing throughout business schools. To remain relevant their graduates must learn to speak to a new professional whose domain expertise is playing a vital role in an organization's ability to compete in today's market. Someone whose background is anything but traditional in the world of business. They are called Data Scientists. Professionals who have spent their careers learning how to convert raw data into real-world value by applying advanced scientific methods to data. As is the case with every other domain expert within a company, a middle language must be developed between the graduates of business schools and these domain experts.
The nontraditional aspect of the Data Scientist role in business means the overlap between the approaches this expert takes to solve problems, and the operations a business is concerned with, is less obvious. But in a world fast becoming 'data everything' this represents a serious problem. For graduates tasked with striking effective conversations between themselves and the domain experts, that much-needed high-level language is critical.
There are two facts that lead to the undercurrent of concern within business schools:
- The reality of today's world is that more and more businesses require software that houses models built by scientists; scientists who have analyzed and modeled the complex data the organization has collected; and,
- Many business graduates are not coming out with an understanding of what it is data scientists do, and therefore do not contain the middle language necessary to integrate one of the most important domain experts into their employer's strategic vision.
That...is a problem.
Business schools must learn where the overlaps between data science and business operations exist. This can only be accomplished by learning how the data scientist approaches problem solving within an organization. More importantly, it requires an understanding of how data science differs from any other approach used to solve challenges.
If business graduates do not understand the conceptual distinction between data science and other forms of analysis, they will be unequipped to strike meaningful and value-producing conversations with one of the key domain experts in modern enterprise.
Advice to Business Schools
To assist business schools, here are questions that are high level, yet get to the heart of what it means to do data science. They highlight the conceptual distinctive functions data science brings to the table that make it an altogether different approach to solving challenges.
I encourage professors, administrators, or anyone concerned with the future of business school education, to invite an experienced Data Scientist in for a real-world discussion with their students about what data science is, and what it is not. Through discussions like this a middle language can be built and business graduates can ensure their relevance in today's data-driven companies.
- What type of software is impossible to build in the absence of data science?
- How does data science elevate staff to work on more value-delivering, creative tasks?
- What is the difference between what a software developer automates and what a data scientist automates?
- How can data science augment marketing efforts, sales engagements, operations, and inventory management in a way that is not possible using more traditional forms of analysis?
- How could data science reduce a task that requires 20 levers to pull, down to one that only requires five levers?
- What is it about human decision-making that makes it limited when using large amounts of data?
- Why is there very little overlap between business intelligence and data science?
- What are the differences between Data Science and Big Data? Where do they overlap?
Contact the Data Science team for more information.
Disclaimer: The statements and opinions expressed in this article are those of the author(s) and do not necessarily reflect the positions of Thoughtworks.