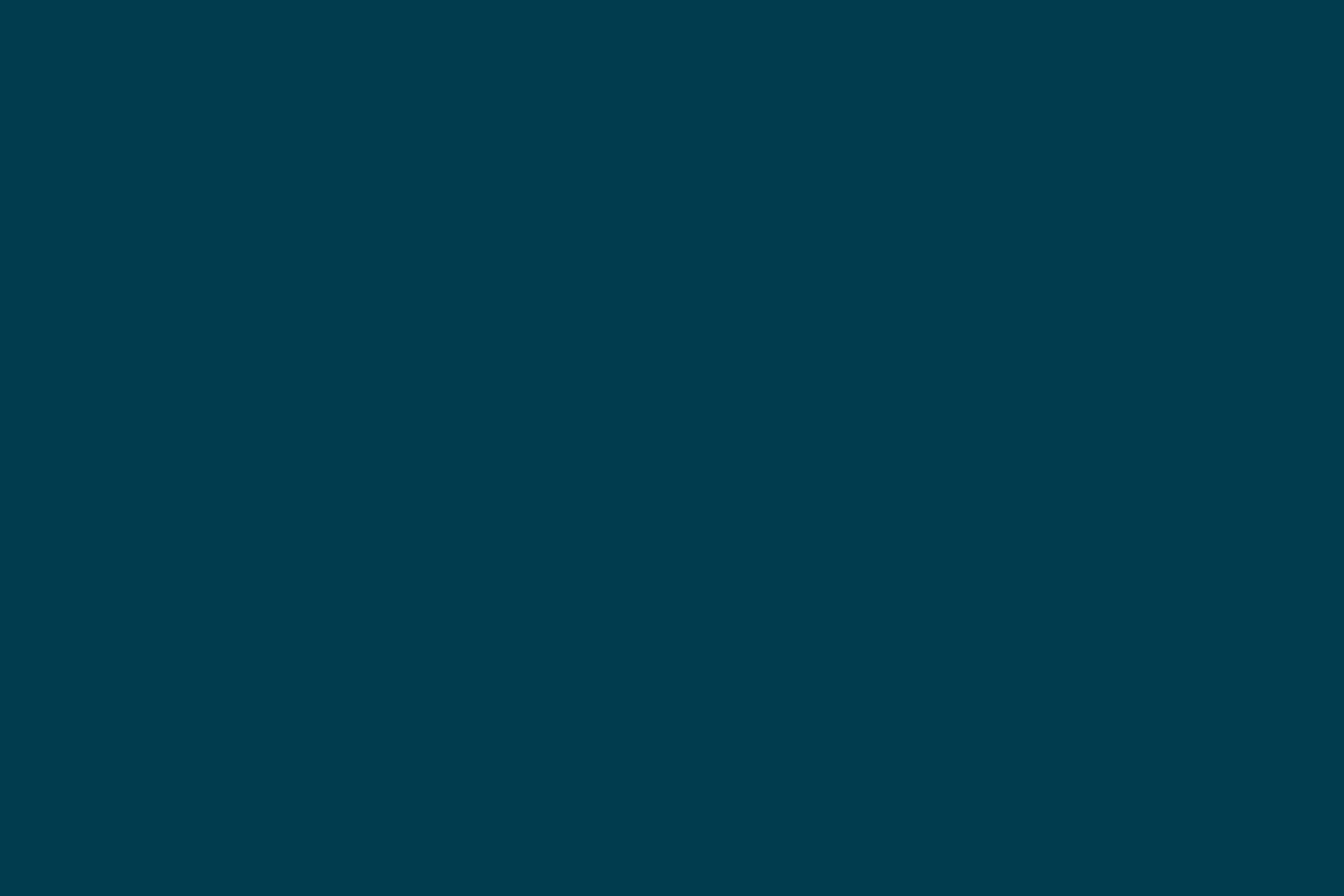
Will Big Data Make me a Designosaur?
I sometimes wonder if a robot could do my job. I’m a designer, and my role is to create digital products that humans want to use. Could a machine learn, faster than me, what humans want? Could it design better things than I could, in less time, with less cost and prettier to boot? I don’t think it’s too far-fetched, given that we’re living in the petabyte age and Big Data is automating many of the utilities in our lives. The potential of machine learning is to automate design.
Hello Games, the creators of No Man’s Sky, used algorithms to design the seemingly boundless universe of stars and planets in the video game. Services like Mark Maker generate logo concepts that are refined through user preferences. Algorithmic design has the potential to tweak a large range of different design layouts which can then be tested in real time to identify the most successful designs. Thanks to Google, Amazon and Facebook’s predictive algorithms, customers are now expecting personalized content that offers relevant options when they search for anything online.
Can a Machine Cry?
Despite the ever-increasing potential of AI and machine learning, I’ve got a few more useful design years before my inevitable obsolescence. There is one factor in my favor—while machines can beat me at chess, I can beat them at empathy. My motivation to design is inspired by a visceral understanding of the human condition. I understand what it feels like to be a person who each day has to endure the abject experiences of transacting with life; people negotiating their daily grind, trying to do their digital banking, pay their bills and apply for their business visa online.
I share their pain and that’s both inspiring and motivating. I want to fix things and make them simple, or at least, pain-free. I don’t see machines sharing this urge of mine, this strange passion. Machines aren’t yet good at understanding the emotions of humans: our weird, messy, unpredictable and illogical behaviors. But that isn't to say that machines can’t see the patterns in human behavior. This is what machine learning is really good for—seeing patterns on a grand scale.
But I can see patterns too. Humans are pretty attuned to finding and attributing meaning to patterns. My best design decisions come from identifying patterns in user feedback and observation, but the ultimate validation is when I see the online metrics in the data. Big Data is the statistical fire hose that can be captured, queried and analyzed to extract meaning and insight about what customers are doing online. I believe that good design draws upon both qualitative and quantitative research.
Chris Anderson predicts that “Big Data will make traditional scientific methods obsolete.” That may well happen, but "the singularity" is still a way off, and the scientific method of positing a hypothesis, measuring and discovering your mistakes, continues to be valid. The trick is to discover your mistakes before you invest too much in building your product. The other is to do it at speed.
Machines Don’t Make Creative Mistakes
To be effective as a designer, I need more than just empathy. I need to be able to test new ideas, quickly. By building technology platforms that allow real-time experiments with new product concepts, we can learn from our mistakes at speed.
One of our clients, a fast-moving consumer retailer, was able to ditch the time-consuming market-testing phase with sample products and skip ahead to testing hypothetical products in the online ordering platform. Using pretotyping, for example, we can segment a percentage of website customers who are shown test products, alongside real products. When users put a test product into their shopping cart, they are informed that the product is still in the concept phase and rewarded with a complimentary item instead. Within days, we have the ability to confidently select the product concepts that customers desire and then commercialize them.
It is relatively easy to take advantage of online customer metrics, but how do you innovate in a market where your customers are still doing things the old-fashioned way? Our Thoughtworks retail team recently had an opportunity to work with a very traditional bricks-and-mortar retailer to develop a mobile app for their customers. That probably sounds like a solution in need of a problem, and we are often presented with those sorts of briefs—an app for customers who don’t think they need one.
Working closely with the retailer's Heads of Customer Experience, Technology and eCommerce, the team decided to turn the brief around and find the real problems that their customers experience. Working at speed, the executive team agreed that we would jointly talk to customers. Senior executives talking to customers! Crazy right? Anyone who has worked in customer research will tell you of the many perils of face-to-face interviews: the biases, the leading questions. So our research team first developed a simple interview script in partnership with the executive team, and then rehearsed the script together to iron out the potential pitfalls. We accompanied the executives to the first of six stores, to conduct interviews in pairs.
On that first day, over 50 customer interviews were completed, and the process was repeated six times over the course of the first two weeks. At the end of each research sprint, the insights were captured; such as the pain customers experience when the product they have been looking for is out-of-stock. With the executive in the room, it was quick to prioritize the opportunities to solve the problems that customers complained of; like missing out on in-store events and incorrect pricing. Within two weeks, a simple prototype of the shopping app was tested with customers before a single line of code had been written. The team was confident that what they were building was useful to customers. Further validation was gathered through online surveys to customers, delivering statistical validity to the designs.
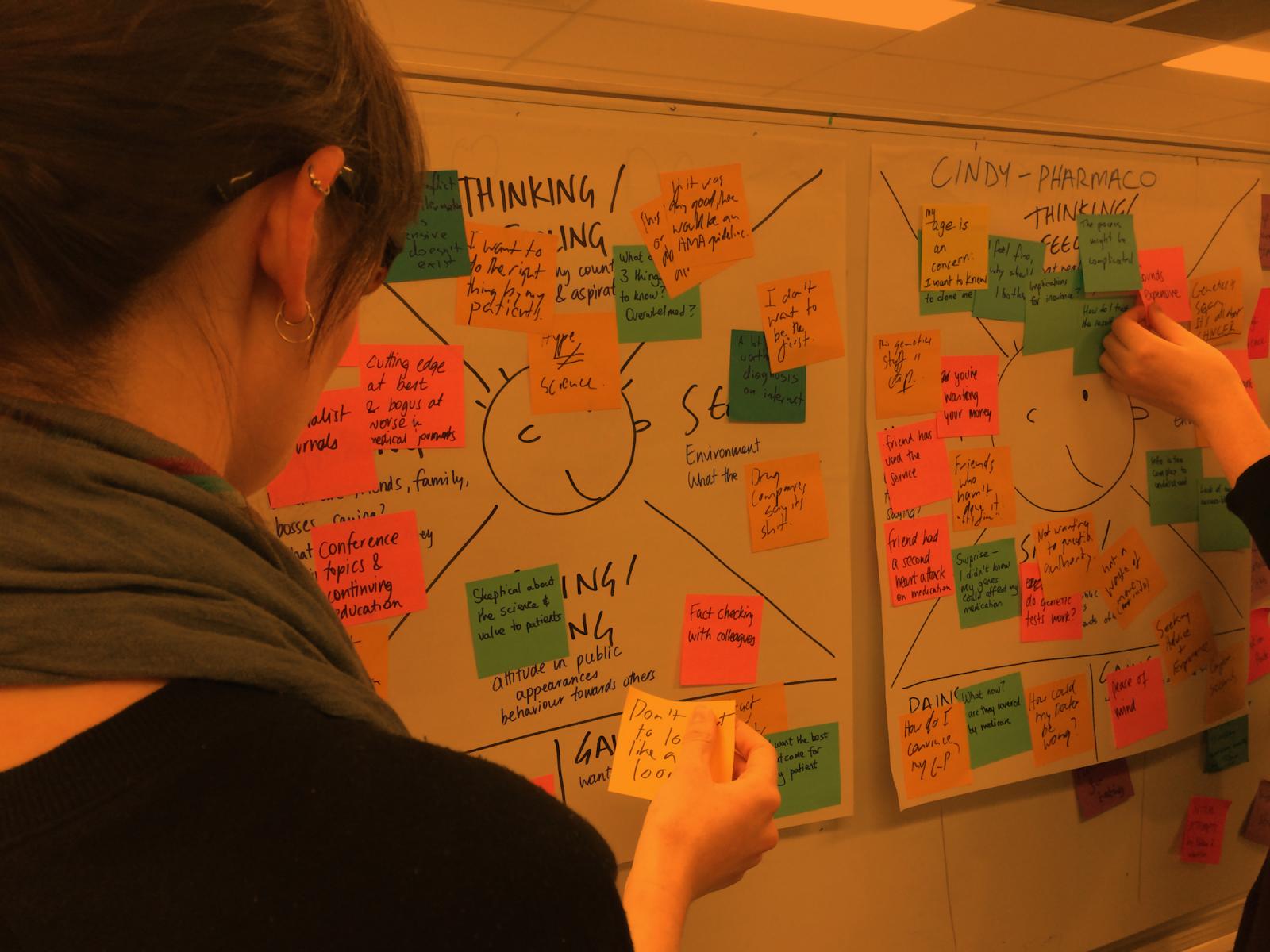
Appealing to the Heart
For businesses that are competing in a teeming market, offering a differentiated customer experience can mean the difference between winning or losing. The brands that get it right encourage a company-wide shared understanding of customer purpose. In a customer-centric organization, it is hard to imagine how machine learning could replace the understanding and empathy that is afforded by directly interacting with your customers. If we only focus on the quantitative metrics, then we risk optimizing our businesses purely for efficiency and speed, over delivering lasting customer value that can build loyalty and trust.
Machines are great for automated, precise, repetitive work, not so great for creative, expressive work. In the same way that designers can craft a brand experience that is memorable, unique and differentiated, it is hard to imagine a day when software algorithms can design either a logo or a brand that can appeal to the heart.
As customer experiences increasingly exploit connected devices and sensors, I think there will be an even greater need to design holistically, for the individual, taking into account a single view of the customer journey. For consumer brands, I think there will be an increasing need to employ multi-disciplinary designers who can design experiences, not just touch-points or interfaces. Tomorrow’s product designers will need to understand their customer, be able to design experiments to validate their ideas and work alongside data scientists and delivery teams to build solutions that may involve a combination of materials, software, and hardware. We will need to increasingly design connected experiences that are forgiving of human behavior.
For the time being, there is still a role for the designer who practices human-scale research methods. There is a need for the type of creative intelligence that can only be inspired by human behavior. Big Data and machine learning will empower design thinking, but it won't replace the empathy derived from human conversations.
Disclaimer: The statements and opinions expressed in this article are those of the author(s) and do not necessarily reflect the positions of Thoughtworks.