Even in a challenging economic environment, companies are pouring investment into artificial intelligence and machine learning projects in the hope of gaining critical business insights, or staying one step ahead of the competition. Yet evidence shows this spending doesn’t always drive value, or the desired results. The typical firm will wait months, even years, to see payback on an ROI project.
The wait for AI ROI
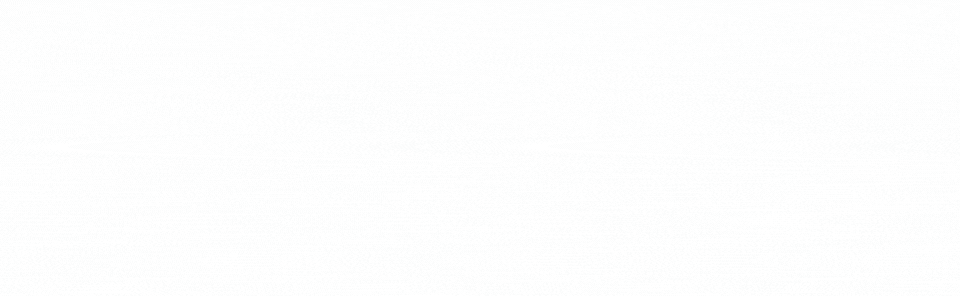
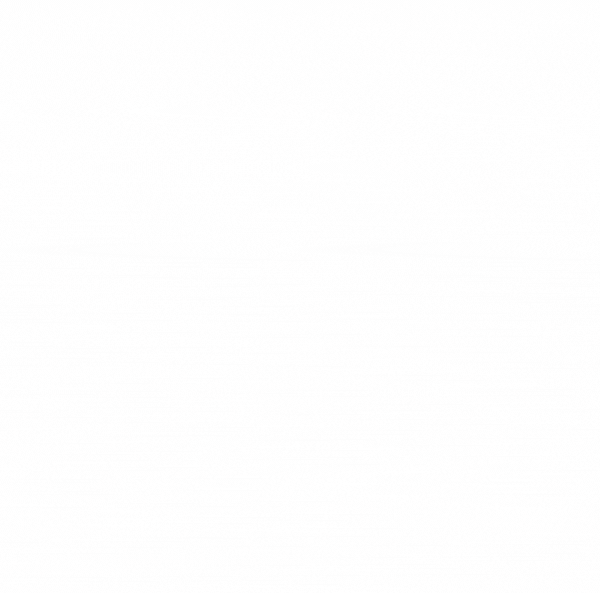
In this edition of Perspectives, Thoughtworks’ experts unpack techniques and practices to help ensure AI is not just capable of delivering return on investment, but also contributes to the organization’s strategy and innovation portfolio.
i. Identifying and acting on AI opportunities
AI has never been more powerful, or more accessible - but that doesn’t mean it’s always the right solution to a business challenge. Understanding the best general use cases for automation, identifying specific processes that are ripe for improvement, and careful planning and testing should all be part of an evaluation that precedes any major AI plunge.
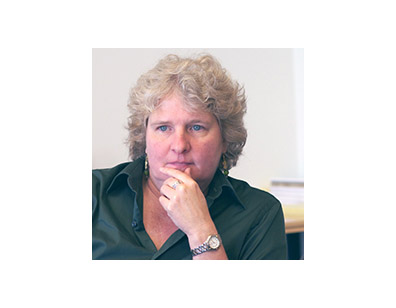
“More often than not, AI is a distinctive tool looking for a problem. People have spent a lot of money trying to do something they thought was the right thing and it didn’t work. That doesn’t necessarily mean the technology is wrong - it might just be that it’s being misused.”
Rebecca Parsons
Chief Technology Officer, Thoughtworks
A convergence of technology forces lays the foundation for AI to shine
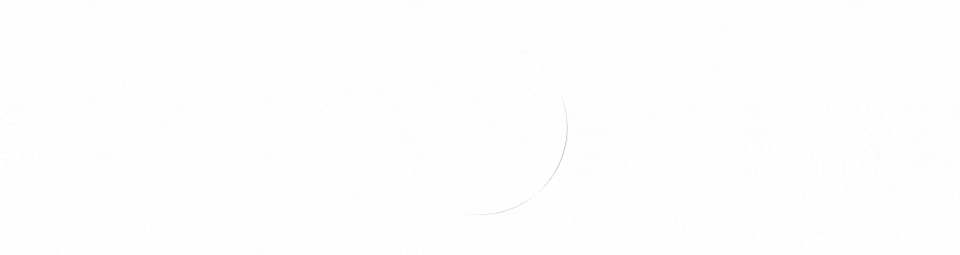
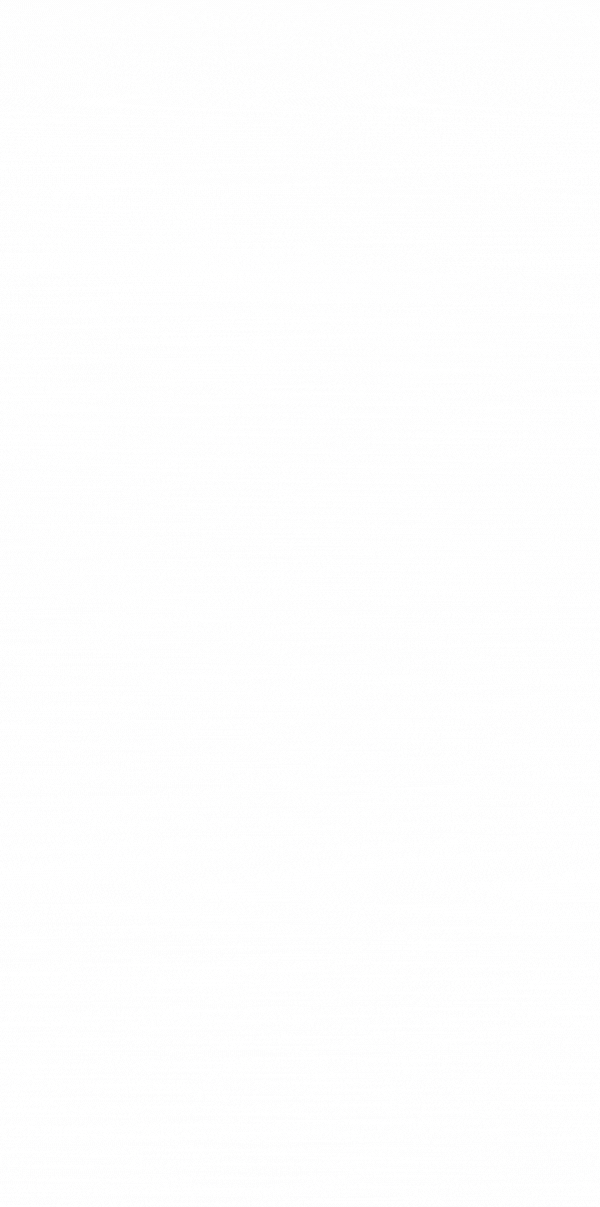
ii. From automation to augmentation - and creativity
Automation is the standard goal in AI/ML practice, but is in fact just one aspect of a much broader potential value chain. By graduating from automation to augmentation companies can reap gains that go beyond productivity and efficiency. At its best, AI becomes a tool to help organizations solve complex problems, anticipate and adapt based on real-time data, and even create new products or business lines.
The AI value continuum
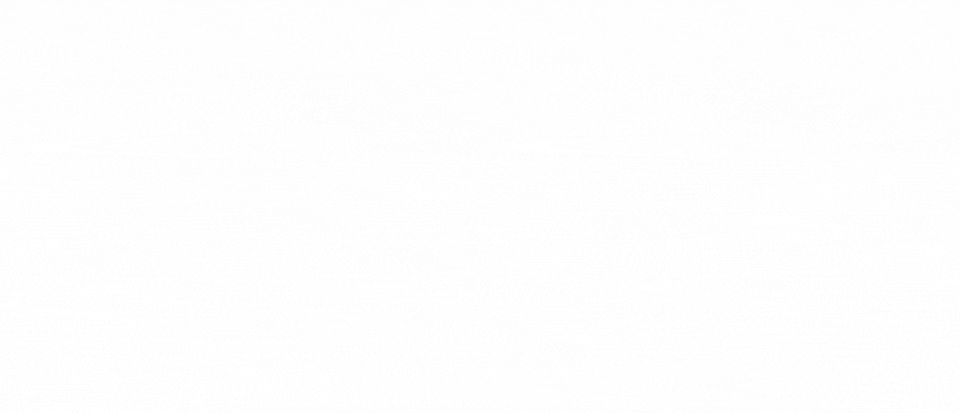
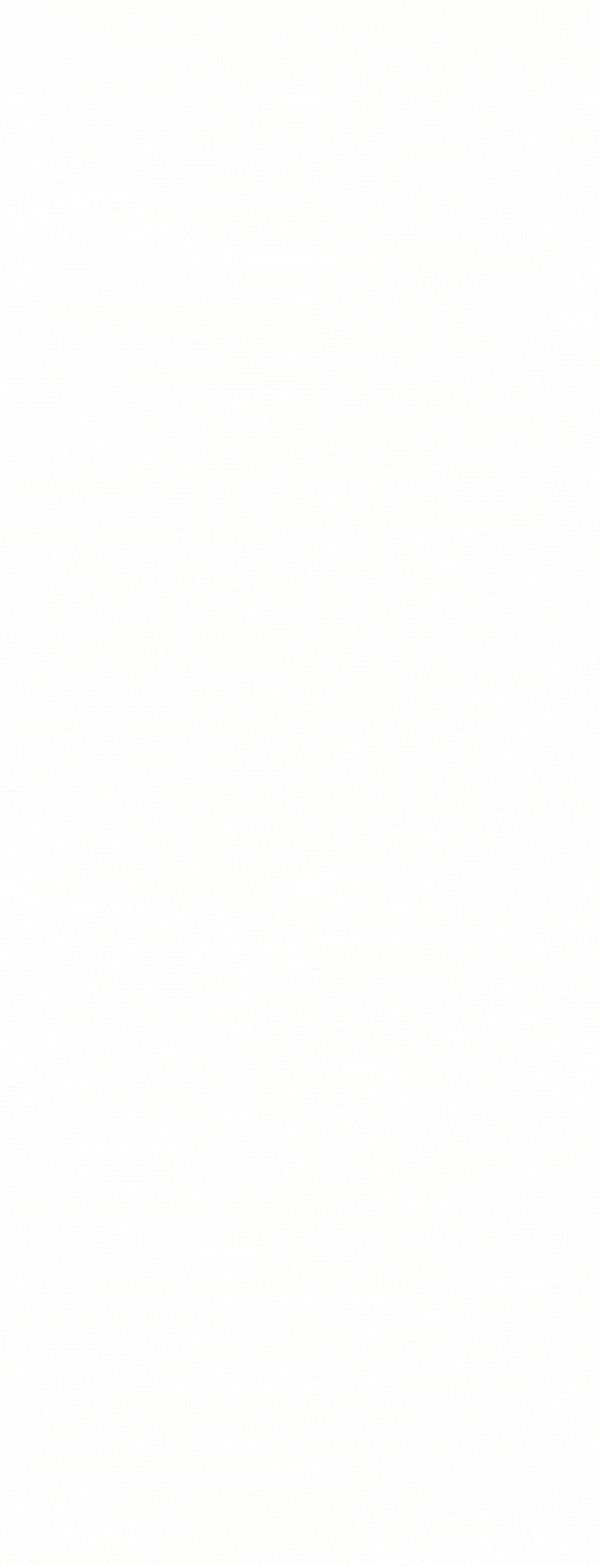
iii. Navigating data, human and ethical dimensions
Building a successful AI practice for the long term requires organizations to manage three key challenges:
Data: If data sources are disparate, distorted or simply unavailable, AI systems can generate incomplete or problematic outcomes that spiral into major issues, as machines learn based on previous faulty conclusions. Sometimes the best approach can be to start fresh.
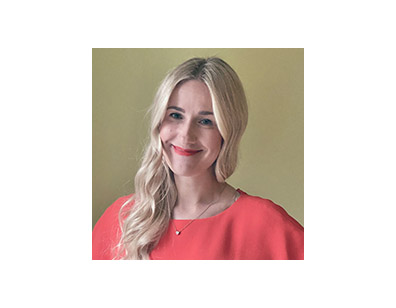
"It can be easier to get started with real-time insights than historical data because the solutions are typically more lightweight. The idea is to try out different things, and see what works and what doesn’t…. to react to fast-developing trends.”
Maria Pusa
Principal Consultant at Fourkind, part of Thoughtworks
People: AI projects inevitably touch on human roles, and those impacted need a say in how they’re rolled out and a degree of education to secure the necessary organizational buy-in. Broad-based support is vital in AI implementations because organizations are likely to find themselves short of dedicated talent.
Talent shortage weighs on AI adoption
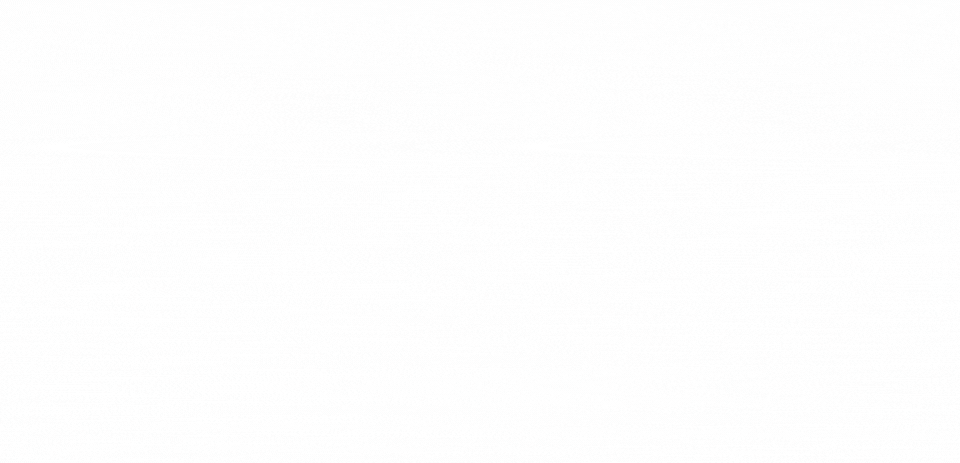
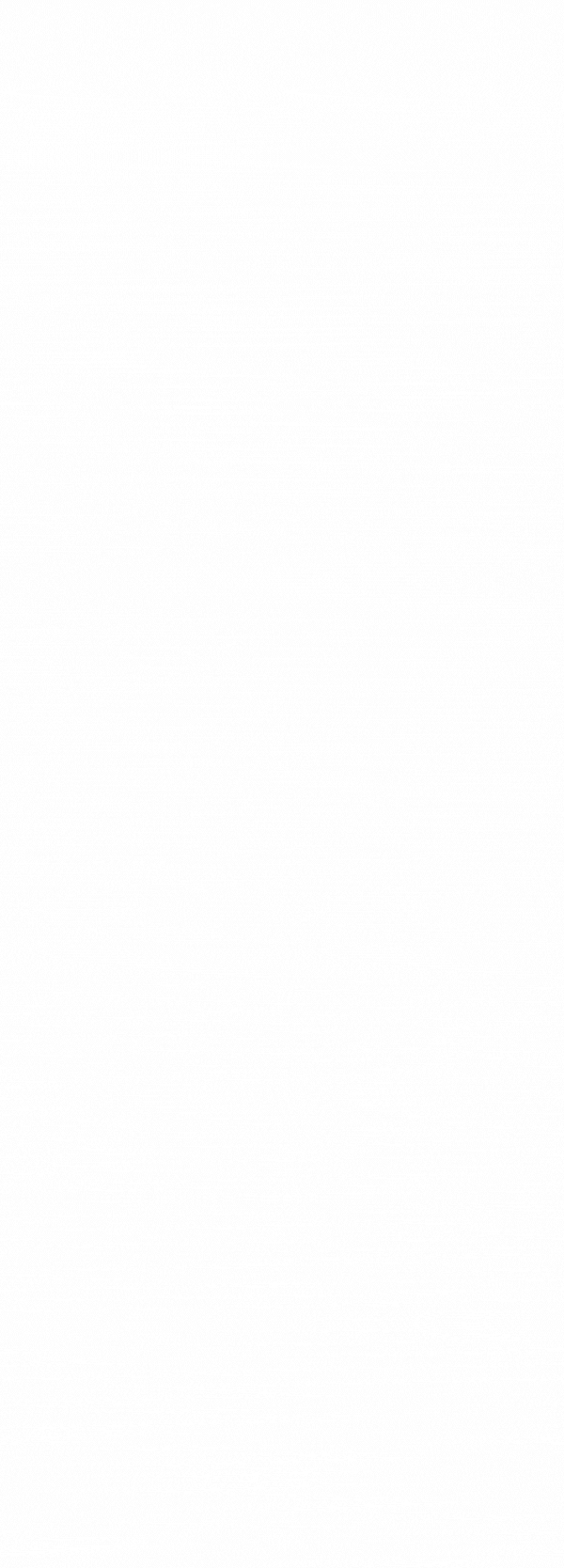
Ethics: Allowing systems to make decisions like who gets access to a product or service, or when an image or interaction presents a possible problem, means these systems may have direct ethical implications. That puts the onus on the business to ensure AI-driven decisions are transparent, traceable, and as free of bias as possible.
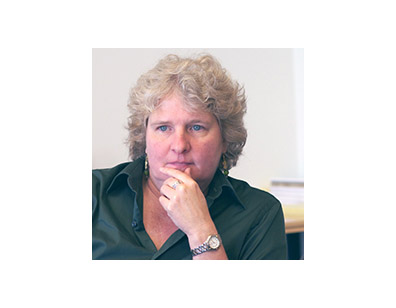
“To the fullest extent possible, you want people involved who have different views of the system, and different intersection points with the system or with the data. You want some people who are very familiar with how it’s supposed to work, and you also want people who are familiar with the consequences.”
Rebecca Parsons
Chief Technology Officer, Thoughtworks
From old limitations to new possibilities
AI is a fast-developing field, with unprecedented computing power, tailored algorithms and natural language processing all set to extend the frontiers of what’s possible for businesses. Enterprises can look forward to AI playing a more strategic and creative role as the age of augmentation arrives in earnest. At the same time, there are tasks that no system can take over.
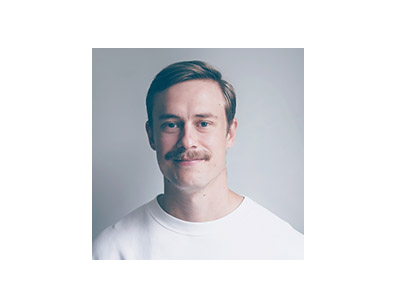
“Reinforcement learning is really underused, but it’s going to be the biggest thing since the AI hype started in two to five years. The idea is that you don’t take all your past data and depend on that, but create an agent that takes actions and learns from the feedback in real time, completely autonomously.”
Jarno Kartela
Principal Consultant at Fourkind, part of Thoughtworks
Perspectives delivered to your inbox
Timely business and industry insights for digital leaders.
The Perspectives subscription brings you our experts’ best podcasts, articles, videos and events to expand upon our popular Perspectives publication.