The best customer experiences feel invisible; they help users go about their day-to-day activities and achieve their goals with minimal effort or thought. And they are increasingly powered by artificial intelligence (AI) and machine learning (ML).
Whether it’s real-time decisioning guidance on Netflix or Amazon, or quick dispute resolution on Uber, customers now expect a certain level of automation. However, many organizations are yet to realize the full potential of AL/ML-enabled customer experiences, despite amassing a vast data pool.
At Thoughtworks’ recent Digital Leaders Forum, we explored opportunities for business leaders and their organizations to use AI to enhance their customer experience.
During the event, the audience was asked to describe in one or two words how exceptional customer experience should make their customers feel. The word cloud that formed showed the potential to differentiate at a deeply emotional level – creating satisfied, excited or delighted customers who feel understood, confident and secure.
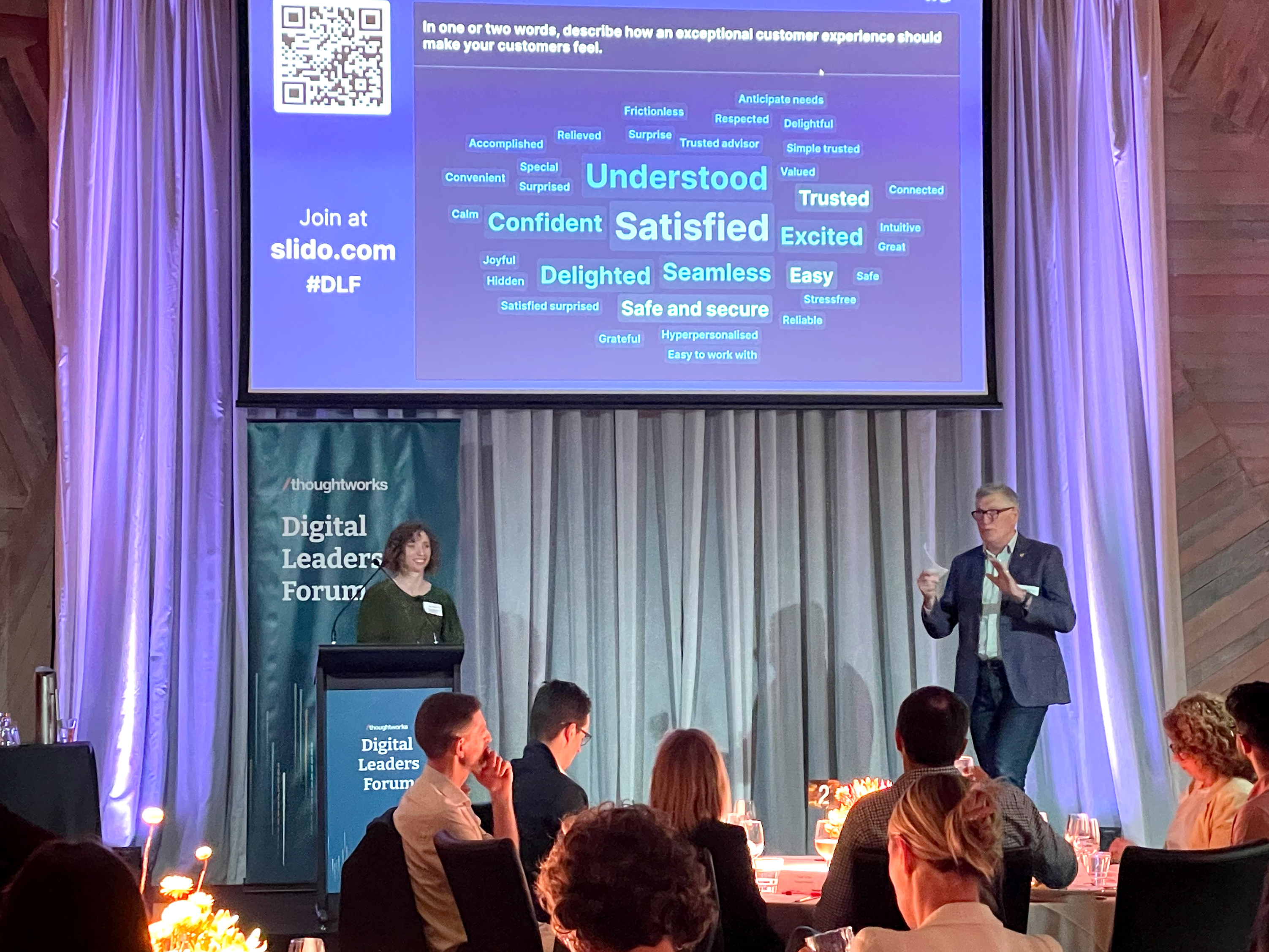
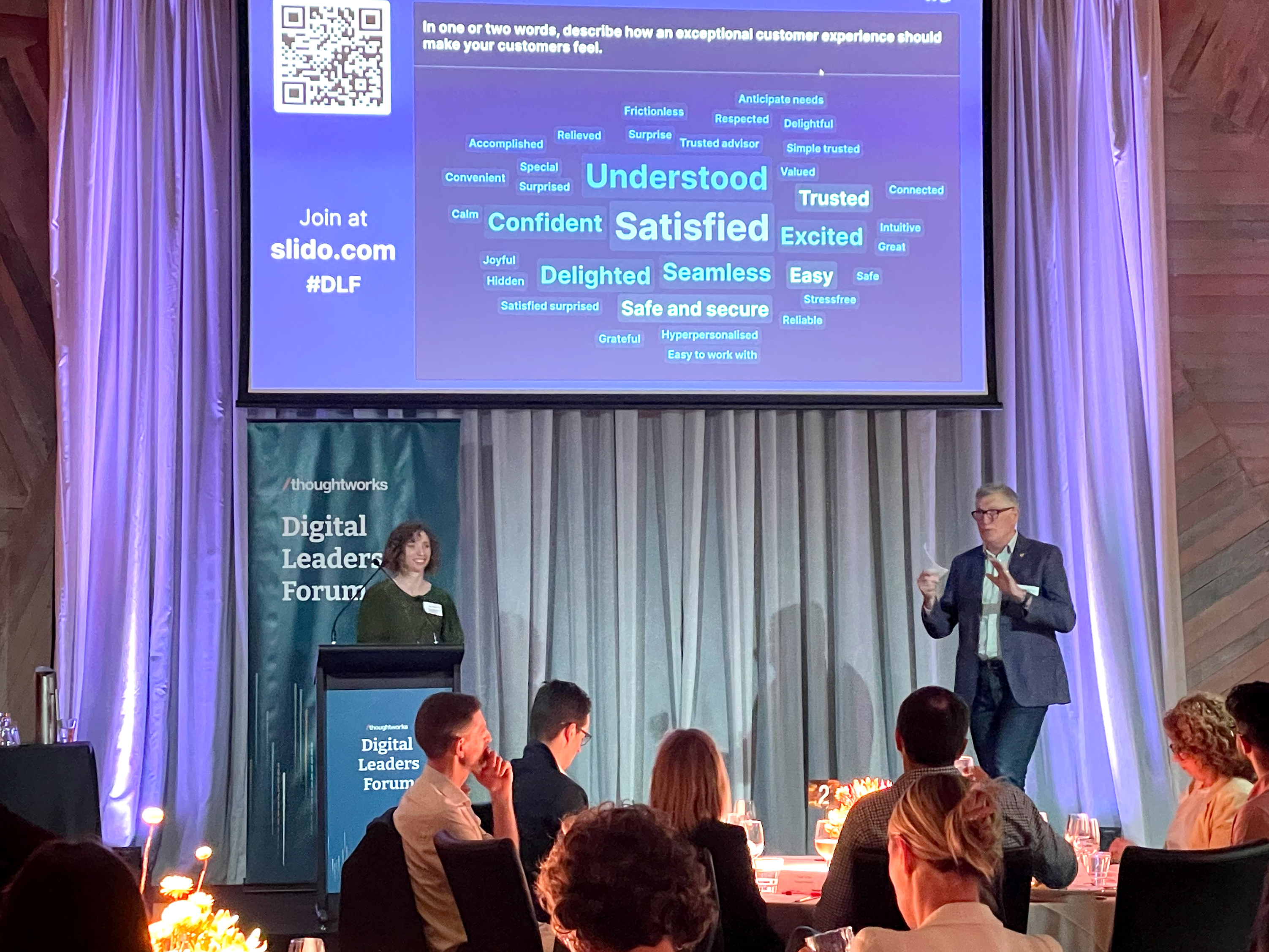
What’s holding AI-enabled customer experiences back?
Many organizations tell us they lack in-house AI and ML capabilities or expertise to identify, prioritize and execute AI-enabled customer experiences. Too often, technology-driven proof of concepts fail to align with real business use cases, while business stakeholders frequently fail to recognize the possibilities a technology partnership could deliver.
In addition, their systems aren’t set up to gather and organize the right data with real consent (or curate data of the right quality) to feel confident in the solution. They may even be unsure what data they are collecting – and why.
Another challenge is customer hesitance – while they may expect frictionless experiences, they are not always willing to exchange their data to receive it – for example, one source suggests 43% of eCommerce shoppers prefer to checkout as a guest. That means you’ll increasingly need to rely on anonymized channel, location and behavioural data to guide their experience.
So how can you overcome these hurdles – to deliver impactful and personalized experiences – at scale? Here are six guiding principles to help you deliver AI-enabled CX.
1. Stop fixating on the “single customer view”
Collecting every possible data point about a customer – their personal information, purchase history, interactions, behaviour, intent – used to be the holy grail for CX. But now we realize a single customer view is not necessary to deliver a genuine, useful customer experience. Ultimately, the need for data may depend on the stage of your transaction or relationship.
The more identifiable data you collect, the more informed consent you will need to obtain – creating additional friction. Instead, focus on understanding the customer in the context of the specific task they’re trying to achieve. For example, anonymous episodic customer data could be enough to power an eCommerce product recommendation tool. But if a customer is seeking after-sales support, they will appreciate not having to re-enter information they expect you would hold from their order.
2. Think ‘less is more’ with data austerity
At Thoughtworks, we advocate for data austerity. That means only collecting data when there is an obvious case for its use (not ‘just in case’). Handling data with the utmost care, and only retaining absolutely necessary personally sensitive data for as long as it is needed and no longer – rather than seeing it as a source of potential revenue. Hoarding data can turn it into inventory, an overhead to manage, rather than an asset.
It’s also worth remembering that historic individual preference data can quickly become irrelevant. Your organization may have specific data retention requirements, but don't confuse regulatory or audit mandates with keeping customer data for other objectives. There’s also the risk of inadvertent data hoarding if you lose visibility over what is retained.
Data austerity, or data minimisation, also means only propagating real data where it’s absolutely required. For example, in most cases synthetic data would be adequate during product testing. It’s also important to avoid using real customer data to train synthetic models. Sensitive information could also potentially leak that way – and it’s unlikely you have explicitly gathered consent for that purpose.
The risks are all too apparent. In the aftermath of recent high-profile and widespread data breaches in Australia, we can expect to see still greater regulations on data privacy and consent, given higher penalties for breaches have already been introduced. So it’s important to be transparent with customers about the way you use their data, and build in privacy by design – using principles that consider privacy at every level of solution design.
3. Decentralize your data models with Data Mesh
Data architecture is the foundation of every organization’s data strategy, but it's not just the domain of CIOs and data architects.
Turning data into actionable insights and better business outcomes has been the goal of organizations for well over a decade. Recently, organizations have turned to Data Mesh, a new approach to data management, based on a distributed architecture for data management. The idea is to make data more accessible and available to business users by directly connecting data owners, data producers, and data consumers, while also allowing owners more control over privacy outcomes.
A recent white paper by Harvard Business Review Analytic Services discusses the benefits of using a decentralized approach to data – and the disadvantages of a centralized data management system. It explores how companies are implementing Data Mesh, the challenges they are facing, and how they are overcoming them. The report also focuses on how to form Data Mesh teams and how to create and track metrics.
Download the white paper to learn more about creating business value with data mesh
4. Build a better data quality system
Data quality issues could be wasting up to 40% of your team’s time – and can undermine your customer experience and introduce risk, despite best intentions.
Often quality issues result from poor collection processes and legacy systems. Improving customer experience may also require training and a culture change – for example, do your salespeople understand the importance of putting the right prospective customer data into your CRM?
Shifting to a Data Mesh approach can also support the modernization of legacy systems. Dash Mesh models treat data as a product, thinking about the specific ‘job to be done’ with that data - such as detecting fraud.
Instead of a massive data swamp, collaborative teams can curate the contextual customer data they need to provide useful insights that power better customer experiences. Data Mesh can also build in clear governance structures to ensure data ownership is with the right people, and aligned with decision-making.
5. Set up an AI/ML capability – without creating more siloes
You may have great ideas on how artificial intelligence or machine learning could enhance customer experience, but lack the capabilities in-house to evaluate, prioritize, and test those hypotheses.
One solution is to create focus areas of specialist expertise, which can be deployed to support teams to run small experiments to test and learn. These specialists need to be embedded in the value stream approach to work – not separated in a center of excellence. Otherwise, every data handover has the potential to add friction and reduce data quality.
When this approach is executed successfully, you can quickly iterate, learn, and then apply the same approach to the next product team platform.
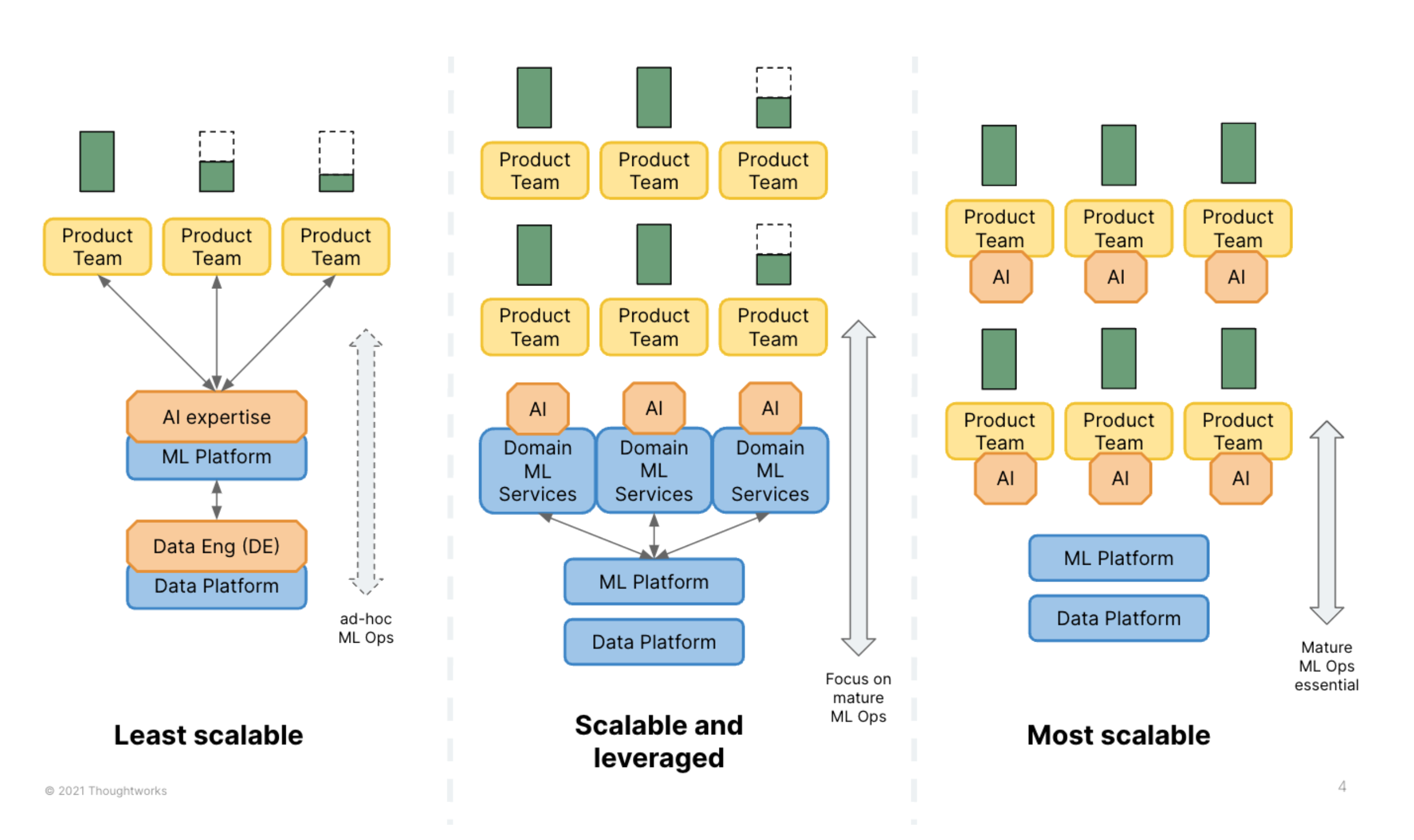
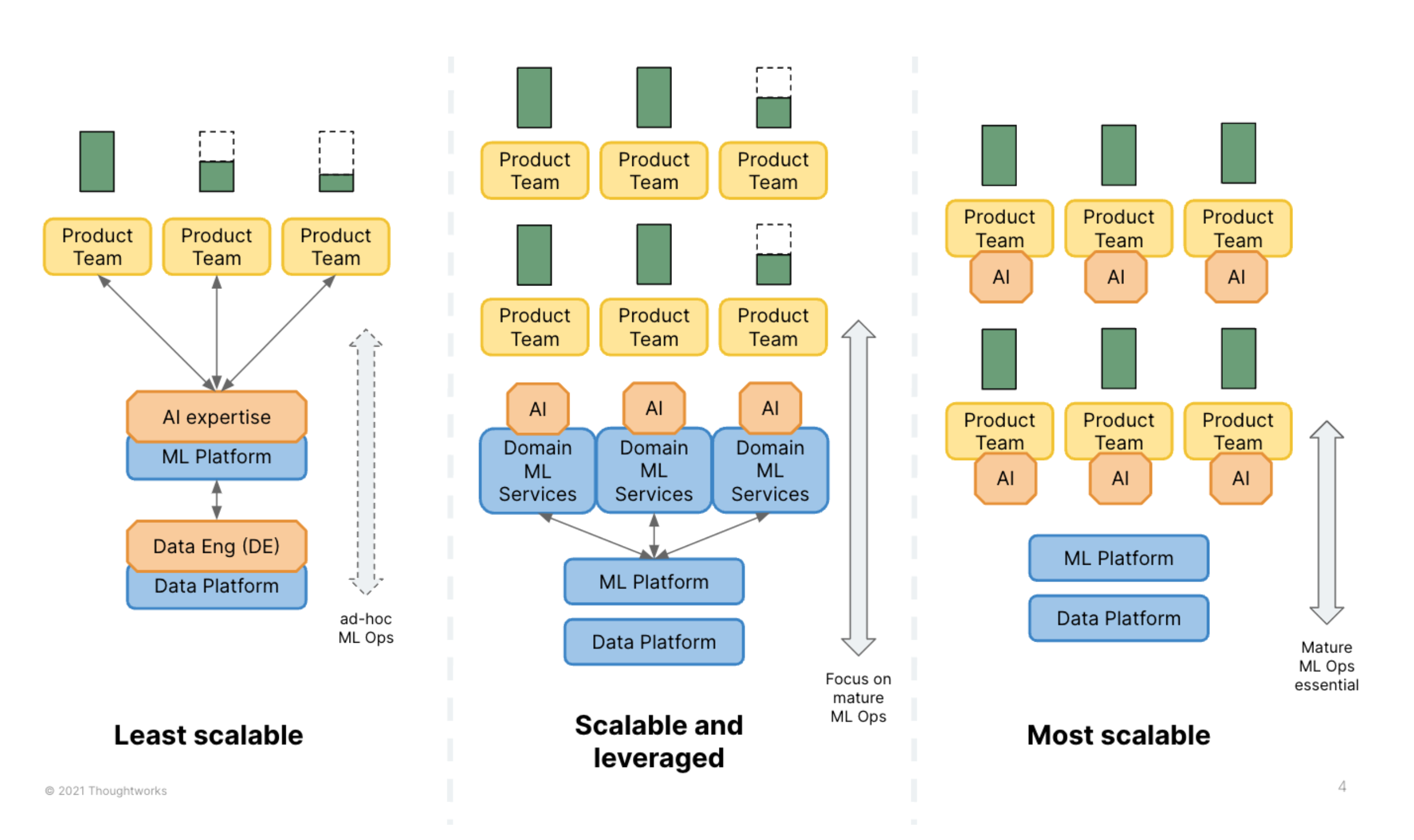
6. Use AI to augment – not just automate
Artificial intelligence is now seen as a valuable tool to augment human interactions, rather than replace them. And it’s worth bearing this in mind with high stakes or high stress customer experiences – such as travel disruption or a home loan approval. If the only option is delivered by an algorithm, there is a risk of frustration, or even reputational damage.
AI can reduce the time and effort it takes to provide the ideal customer experience, but some level of human intervention will continue to be required to assess and mitigate the risks of unintended AI biases. For example, There have been examples of algorithms in financial services, healthcare, policing and recruitment discriminating against people on the basis of race or gender. Dr. Wallace acknowledged there is still much to be done to ensure AI is deployed within an ethical framework. Her book, Checkmate Humanity, outlines the world’s first taxonomy of AI harms and offers recommendations to guide organizational decision-making.
Ultimately, machine learning is just that – it is constantly learning, and there is a potential cost when it gets things wrong. Even the best system will occasionally fail, which is where human insight and allowance for intervention will continue to be essential.
That’s why it’s so important for Australian organizations to start investing in developing the AI and ML skills they will need for tomorrow. Armed with the right capabilities and data platforms that build in privacy by design, we can find new ways to safely deliver the personalised, yet anonymised, customer experiences customers expect. Experiences that will make them feel delighted, satisfied and understood. And then we can be ready for the next CX frontier – in the virtual world of the metaverse.
To discuss opportunities to enhance your customer experiences with responsible AI, please get in touch with our team.
Disclaimer: The statements and opinions expressed in this article are those of the author(s) and do not necessarily reflect the positions of Thoughtworks.