Looking Glass 2025
Strengthening the data value chain
Leveraging data platforms and AI
As enterprise adoption of AI gains pace, there’s rising awareness of data’s role as a differentiator, and a source of competitive edge. Developing the capabilities to leverage data at speed and scale, and become truly data-driven, has become an emerging priority. Treating data as a product represents one of the most effective means to achieve this goal, and the best way to build and distribute data products is through data platforms.
The principles that underpin high-performance data platforms remain the same — decentralization and federated data ownership — but new trends and opportunities in the space are presenting challenges that organizations need to be prepared for. In particular, the rise of generative AI (GenAI), and the importance of unstructured data in it, requires teams to think differently about how data is managed and processed. It’s becoming critical to treat unstructured data as a first class citizen, not as structured data’s poorer cousin.
It’s also important to note the rising need for better — and ideally automated — governance of data products.
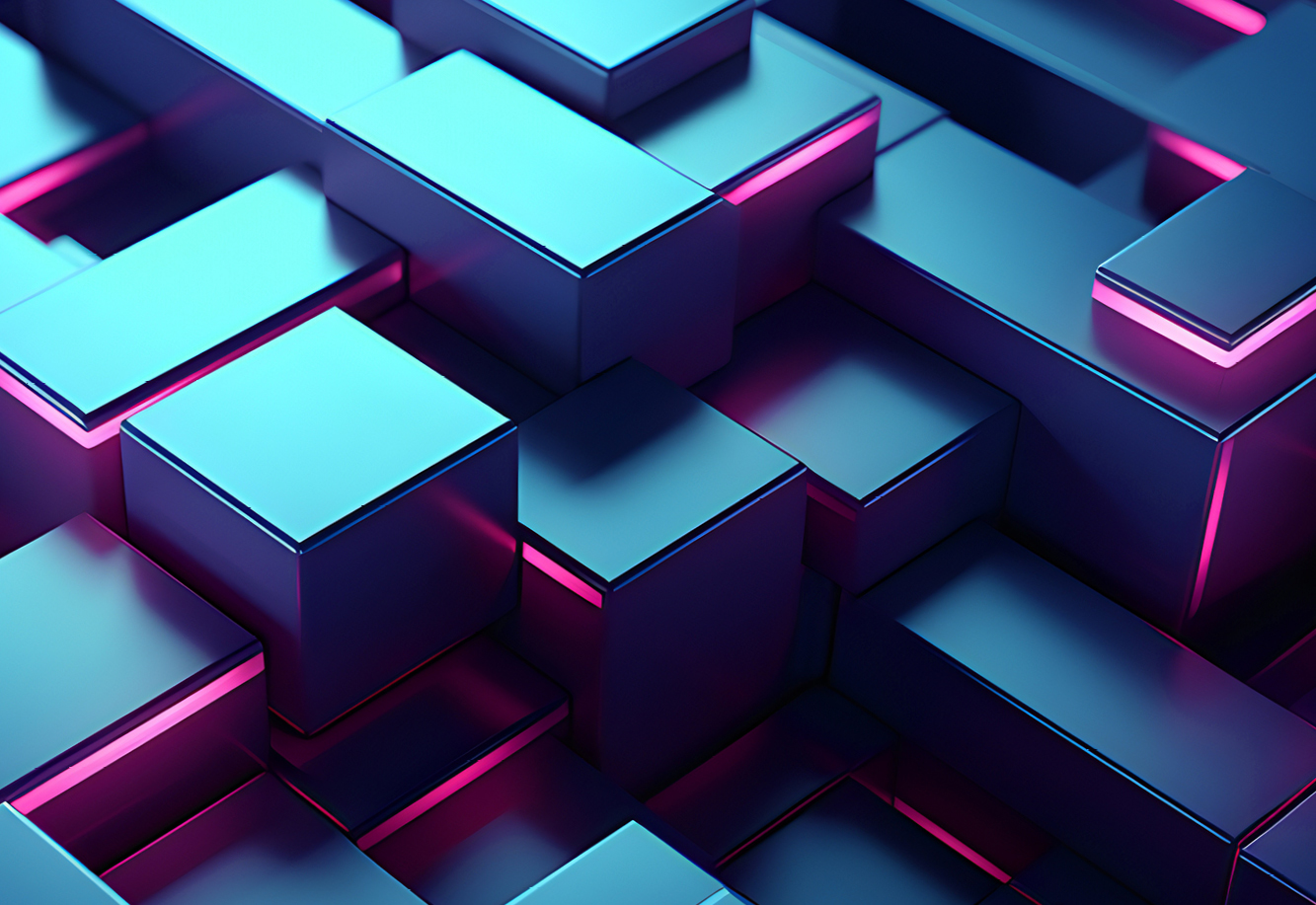
Data products — reusable data assets engineered to deliver trusted datasets for specific purposes — exist in dynamic environments where the needs of teams and the wider organization are constantly evolving, and it’s important that they also develop in a way that delivers value.
Maintaining the capacity for competitive and sustainable change requires intentional design of cohesive centralized and decentralized capabilities. Some organizations are navigating away from creating consensus-based ‘single sources of truth’ to forming integrated ‘contextual truths’.
Equally essential is ensuring data products are built with a clear line to business adoption. Platform and product thinking can help, but there’s a need to move beyond existing paradigms and tooling, and consider applying human-centered design for more effective ways for data to be consumed and leveraged by business users. GenAI and trends like ‘talk to data’ and graph-based discovery are creating promising opportunities in this space, transforming the way teams interact with and consume data.
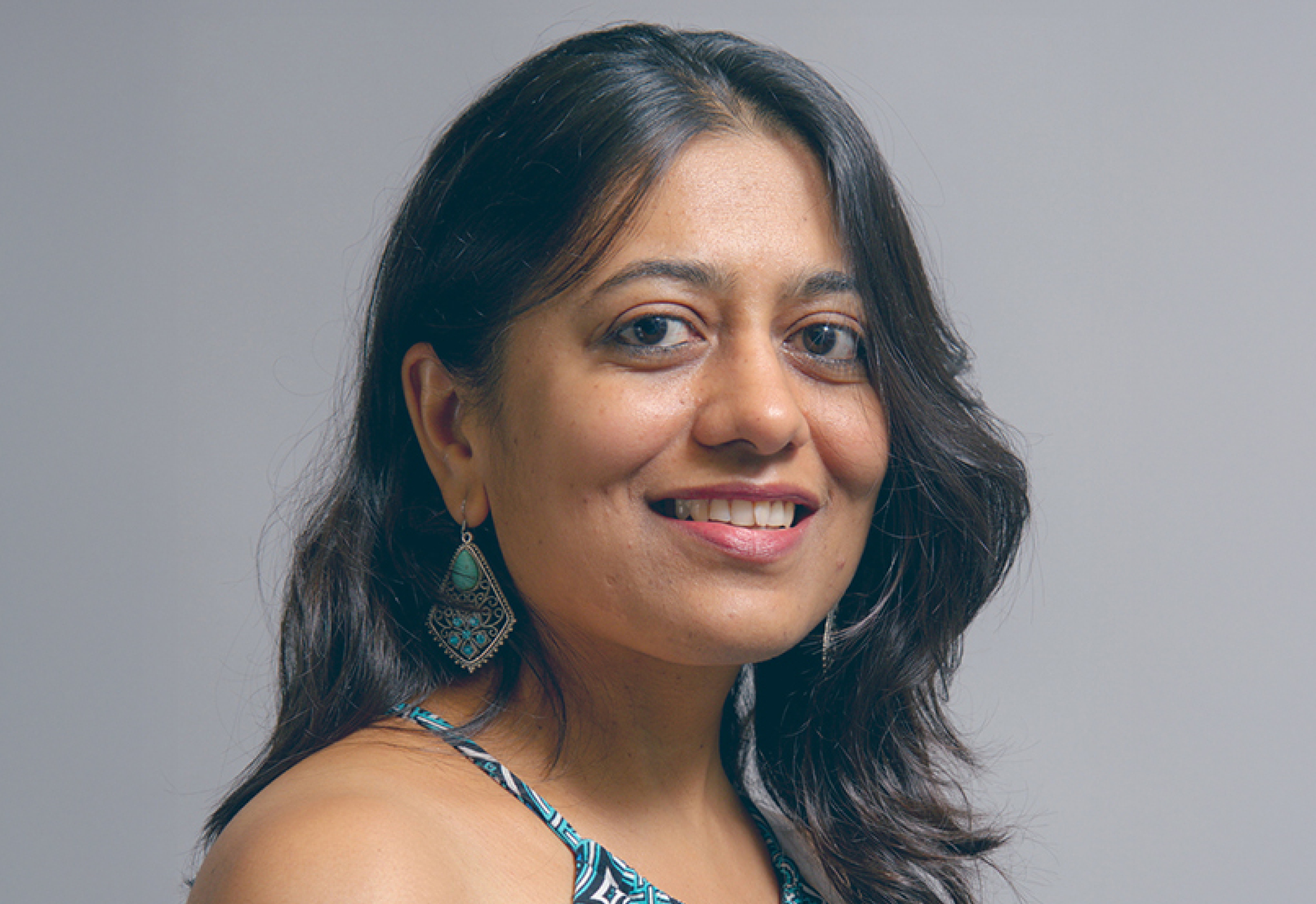
An open and evolving data and AI platform allows organizations to embrace uncertainty in rhythm with changing demands, fostering a culture of continuous learning.
An open and evolving data and AI platform allows organizations to embrace uncertainty in rhythm with changing demands, fostering a culture of continuous learning.
Signals
- Unstructured data moving from a supporting to a starring role. There’s growing focus on the use of unstructured data (such as text, video, images and audio) to build better AI training models, which requires integrating and working across different types of data in as frictionless a way as possible. Startups in this space are gaining significant investment and the likes of IBM are unveiling new products specifically designed to help enterprises unleash the potential of unstructured data in analytics and AI.
- Enterprises applying GenAI to better leverage unstructured data. GenAI’s ability to parse and summarize vast quantities of the information contained in everything from meeting recordings to PowerPoint presentations, and to support natural language interactions, is transforming the way teams access and use data and enhancing knowledge management. However, this trend is also raising questions as to whether AI and GenAI platforms should be integrated with other data platforms or kept distinct, which, in some cases, is leading to platform proliferation.
- More organizations grappling with the challenges of treating data as a product, as it becomes a business imperative. Research shows the vast majority of businesses see clear benefits from such an approach, including improved data sharing and strengthening the connection between data and business goals. However, they are confronting multiple barriers along the way, from fragmented systems to uncertainty about data provenance.
- The rising importance of data discoverability. By empowering users to better discover, understand and use data assets, data catalogs can play an important role in data platforms and a data product approach. But they can also cause more issues than they solve if their user experiences or capabilities are limited, impeding the discovery process. The recent introduction of knowledge graphs to data platforms is addressing these risks, making it possible to draw out relationships and nuances in data that are typically lost in the process of abstraction.
- More pressure being put on data teams to demonstrate ROI and manage costs more effectively. The increasingly established link between data strategy and enterprise performance also means these teams can no longer work in isolation; instead strategies should be co-developed with, and create platforms that deliver results for, the business.
Trends to watch
Adopt
-
AI-ready data is data that has been structured and organized in a way that makes it easy for it to be integrated with AI systems. It has a number of specific qualities: high-quality (auditable and verifiable), consistent across different platforms and robust, comprehensive metadata.
-
A formal agreement between two parties – producer and consumer – to use a dataset or data product.
-
More granular access controls for data, such as policy-based (PBAC) or attribute-based (ABAC) that can apply more contextual elements when deciding who has access to data.
Analyze
-
An emerging set of techniques to certify the provenance of data and to govern its use across an organization.
-
Set of techniques and tools for processing and incorporating unstructured data, such as text, images, and videos, into workflows and decision-making. Approaches like natural language processing, computer vision, and data indexing systems make this data more accessible and actionable for businesses.
-
Technologies enabling the direct interaction of devices and information sharing between them, usually in an autonomous fashion. This enables to decision making and action with little or no human intervention.
-
Talk to data (T2D) is a technology that allows users to interact with and analyze data using natural language queries as opposed to, say, the kinds of analytics and business intelligence dashboards that have become commonplace over the last two decades. It makes it easier to uncover insights and has a lower barrier to entry, giving more employees the ability to explore and ask questions about data.
Anticipate
-
A data architecture style where individuals control their own data in a decentralized manner, allowing access on a per-usage bases (for example, Solid PODs).
Adopt
Analyze
Anticipate
The opportunities for strengthening the data value chain
By getting ahead of the curve on this lens, organizations can:
- Consolidate data and AI platform capabilities, enabling AI as a service to embed this new technology and empower users to leverage it successfully throughout the organization. Surveys have shown that despite concerns about the wider impacts of AI, adoption has positive implications for teams’ collaboration, efficiency and performance.
- Use AI (and GenAI) to build and maintain data products more effectively. Emerging AI tools have the potential to contribute to data products in a number of ways, from synthesizing and analyzing information garnered in end-user research or testing, to accelerating coding and creating documentation that can smooth the path to effective adoption.
- Enhance control over costs. With data management often dominating enterprise technology spending, introducing new tooling to track data lineage and analyze the impact of complex data initiatives can help teams determine and demonstrate ROI with greater precision. FinOps thinking can contribute significantly to this process by strengthening the links between tech and business teams and ensuring investments come with financial accountability.
- Strengthen data governance by introducing emerging best practices and structures. These include data clean rooms, secure, self-contained environments where enterprises can blend proprietary and third-party data to improve analytics and personalization while protecting customer privacy; and data contracts, which by setting ground rules for data users and consumers, can improve transparency and trust when sharing data across an organization.
- Combine knowledge graphs and GenAI, which can enhance understanding of large, complex data sets by mapping the relationships among entities within them. This opens the possibility of more semantic approaches to integration, which in turn can help create a better user experience for data consumers. In addition, combining knowledge graphs and GenAI can also deliver better LLM responses because we’re taking explicit knowledge from knowledge graphs and combining it with implicit statistical knowledge from LLMs.
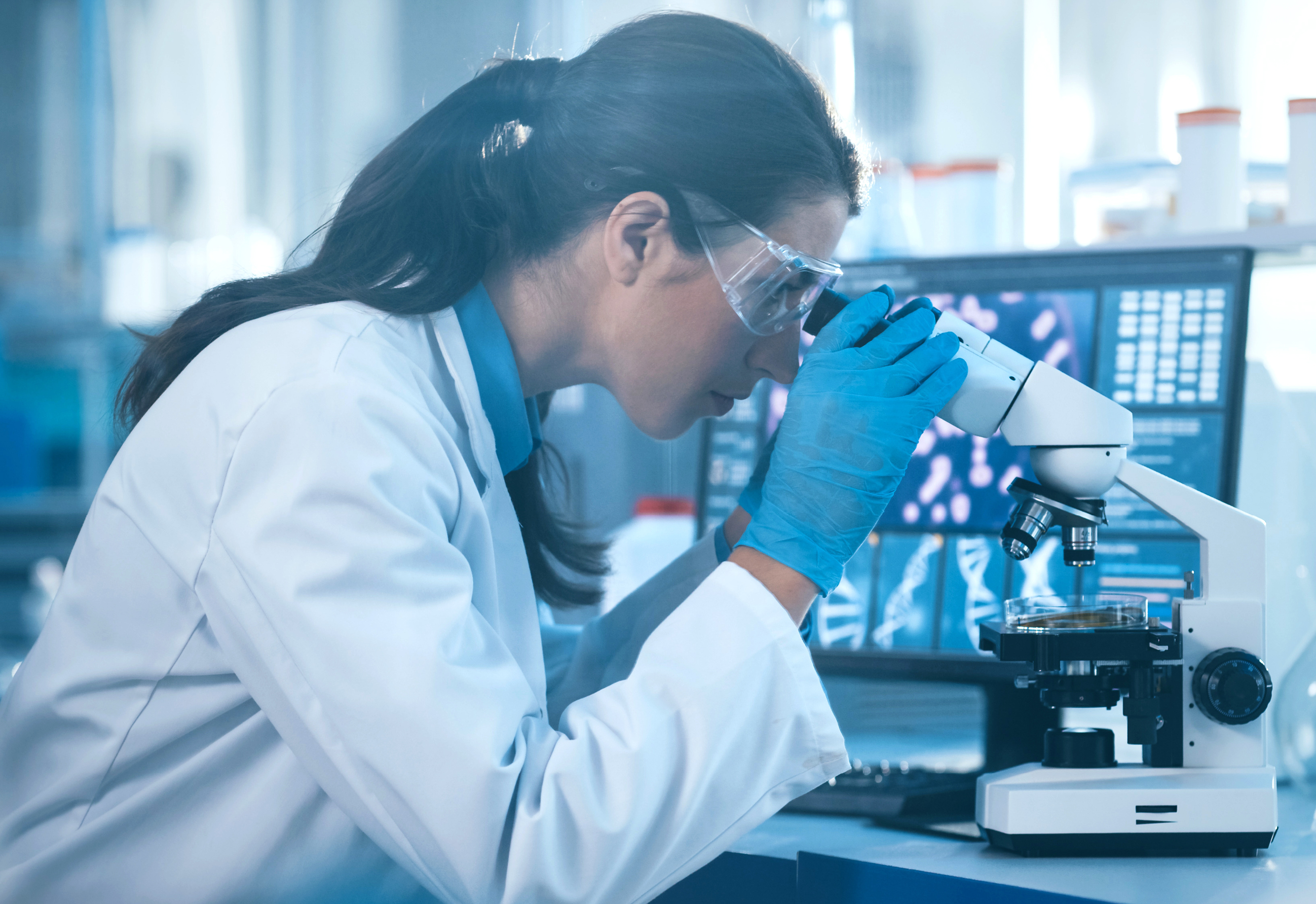
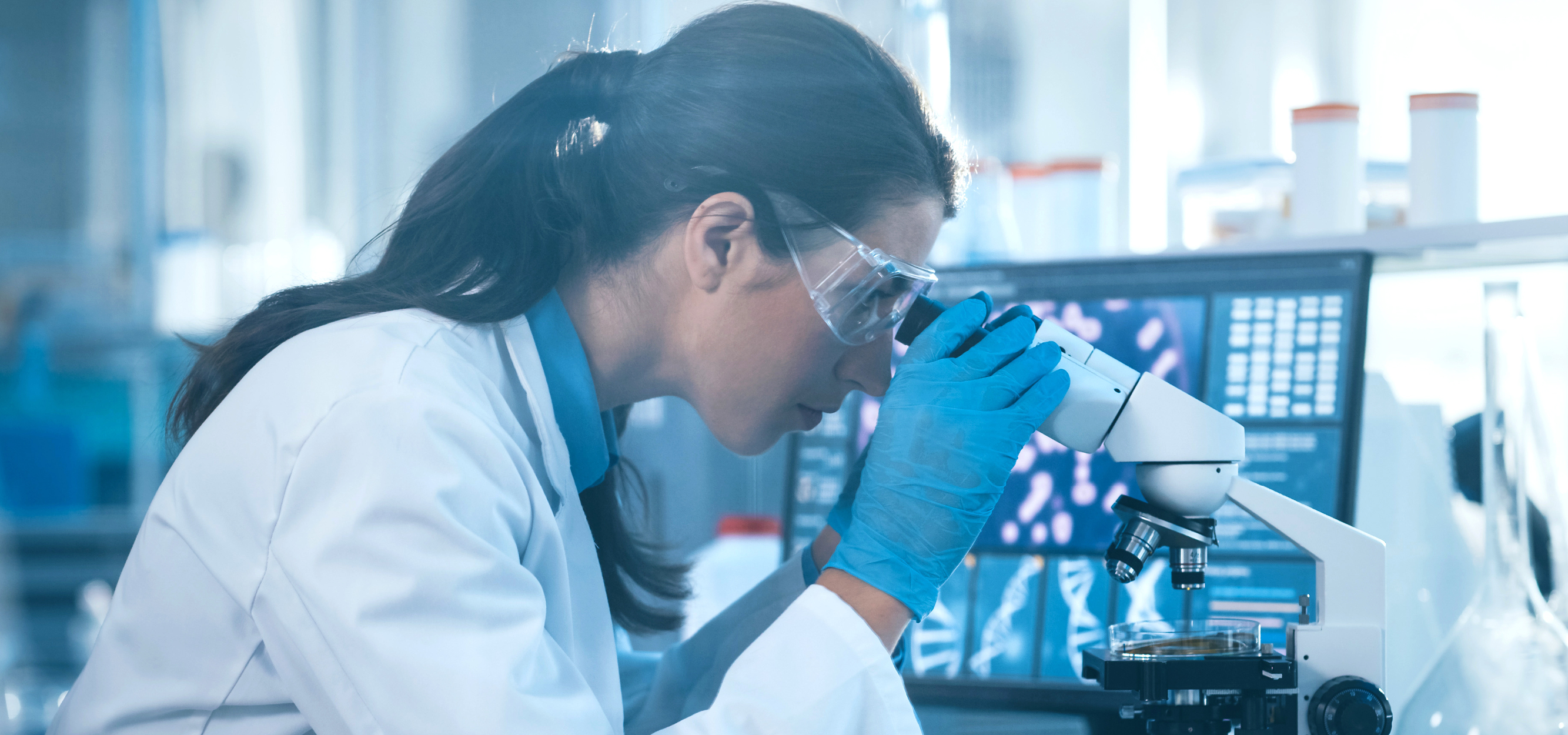
What we've done
Pfizer
Thoughtworks is working actively with these leading pharmaceutical companies to create data mesh platforms that enhance their ability to create and deliver transformative data products. With Pfizer, we helped develop cutting-edge layered platforms serving AI-powered data products, graph-based semantic interoperability, and LLM-based agents that drive the firm’s oncology research, supporting early drug discovery.
Gilead
For Gilead, we supported the design and implementation of Gilead DnA, a scalable enterprise-wide data platform that provides data engineers and researchers with a secure self-service environment for data processing, complete with ‘talk to data’ functionality
Actionable advice
Things to do (Adopt)
- Lay the right foundations for creating effective data products by implementing a data mesh, which places data within the reach of teams that need it most and reduces friction between data producers and consumers.
- Automate data governance as much as possible to ensure policies are implemented consistently and with minimal impact on data usage and consumer experience. Fitness functions and more rigorous monitoring of service level indicators (SLIs) can be good places to start.
- Start treating unstructured data as a first class citizen that is given the same attention and prominence as structured data in your data platform, and draw on its potential to improve analytics and AI models.
- Invest in a superior data product development experience to accelerate adoption. Mapping decision journeys can help the organization better understand and trace how to move from use cases to data, and particularly AI data, products.
Things to consider (Analyze)
- Extend user experience and human-centered design to data and AI. This includes thinking carefully about how to build the best possible interface and experience for discovering and accessing data, out of an expanding range of GenAI-enabled options.
- Examine ways to track and document data lineage and improve metadata for data products for data consumers. Doing so can also enhance data governance and data engineering by highlighting opportunities to smooth the flow of data throughout the organization. AI tools can play a valuable role in this process by providing a quick and precise snapshot of data’s history and transformations.
- Adopt mechanisms to minimize the risk of creeping centralization. Encourage teams to think less about creating a single source of truth and more about adopting federated data management that efficiently delivers what the use case or context demands.
- Track ROI for data and AI transformations. It’s important to be able to demonstrate the value and impact being driven by data and AI initiatives. There’s no single way of doing this, but it’s a valuable step in ensuring teams remain value-focused and that projects in this area have organizational buy-in.
Things to watch for (Anticipate)
- Next-gen user experiences like voice and VR impacting data discovery. By allowing users to query data naturally and moving data visualization into a three-dimensional space, new tools promise to transform the way teams perceive, interact with and understand information, paving the way for deeper analysis and collaboration.
- Propagating more granular access controls as data platforms and products scale to more users and data product development accelerates. Studies show data professionals are already walking a fine line between prioritizing security and not impeding the efficiency and flexibility data platforms are designed to provide.
- Adopting GenAI and knowledge graphs to improve data discovery and better describe and document entities in large data sets.