This is the third article in our series on AI-driven dynamic pricing, discussing common challenges our clients have experienced and how to navigate them. In previous articles, we presented both the business benefits and investment point of view of AI-driven dynamic pricing.
Specifically, in the first article we discussed the business benefits and how to get started with AI-driven dynamic pricing, demonstrating that AI-driven dynamic pricing can significantly enhance revenue and operational efficiency across various industries. Notably, online reinforcement learning outperforms traditional methods like rule-based systems.
Then, in the second article, we argued that it’s beneficial to consider AI-driven dynamic pricing as an investment rather than an operational cost. A tailored system provides a competitive edge. Approaching as an investment through careful planning, solid change management and ongoing validation through small-scale experiments, enhances its long-term strategic value and return on investment (ROI).
In this article, we discuss the typical challenges our clients have encountered and share a path to success with AI-driven dynamic pricing practice, based on our experience with a wide range of clients. Our focus lies particularly on bespoke online reinforcement learning-based solutions, tailored to meet specific business needs.
To craft a path to success using an AI-driven dynamic pricing practice, we build upon the learnings from the two previous articles and discuss some additional challenges. When we inspect the challenges through Thoughtworks’ AI gap analysis framework, we see the typical challenges are more often organizational than technical. Some typical organizational challenges are related to strategic alignment, stakeholder buy-in, change management for successful adoption and a data-driven culture prioritizing data-driven insights and feedback in favor of intuition. Below we explore these challenges including some technical challenges and how they can be overcome.
Ensure strategic alignment
Strategic alignment, where all stakeholders pursue the same overarching business goals, is key for unlocking the potential of AI-driven dynamic pricing. However, organizations often struggle with this. For instance, when management fails to provide the right incentives and goals for tech teams to prioritize user-centric implementation, there is a risk that the solutions provided may not fully meet user needs or deliver optimal business value. Therefore, aligning business goals with stakeholder objectives is vital for success.
To attain strategic alignment, start with defining clear business objectives for AI-driven dynamic pricing, which are translated into measurable KPIs. The OKRs framework provides an effective method for this. Defining these goals involves addressing key questions, such as whether the dynamic pricing solution automates tactical pricing or informs strategic pricing decisions. These objectives are then translated into actionable KPIs, guiding the optimization of the dynamic pricing solution.
After defining business objectives and KPIs for AI-driven dynamic pricing, aligning stakeholder goals and incentives with these business goals becomes crucial. Picture this: sales representatives' bonuses are tied to regional sales figures. They may be tempted to override AI-defined prices during experimentation to safeguard bonuses. However, this hinders the system's understanding of market dynamics, including price levels and elasticity. Aligning incentives and overcoming cultural barriers unlocks the full potential of online reinforcement learning-based dynamic pricing — a vital step in change management.
Note also that the process of alignment is ongoing. Make sure to schedule a regular cadence for review and re-alignment as you progress so that the alignment remains solid throughout the journey.
Involve key stakeholders
Success in AI-driven dynamic pricing depends on considering technical, business and customer perspectives. Neglecting these key stakeholders during development and scaling can lead to suboptimal outcomes or failed adoption. Stakeholder mapping can help to identify the right stakeholders in the organization. Ensure involvement of the key stakeholders by encouraging participation and open communication, bringing in their perspectives, ideas and goals to fortify the programme and increase engagement.
The execution of AI-driven dynamic pricing depends on business stakeholders (leadership, revenue management, product, legal) to drive towards business goals and technical experts (data scientists, ML engineers, data engineers, front-end developers) to oversee that the solution meets technical requirements and is consistently maintained. These technical skills can be either in-house or external, but requires long-term commitment from the business for ongoing maintenance and improvements after initial adoption. Make sure that business and technical stakeholders collaborate to align the technical solution with the business objectives. Cross-functional teams, feedback loops and shared responsibility makes collaboration easier.
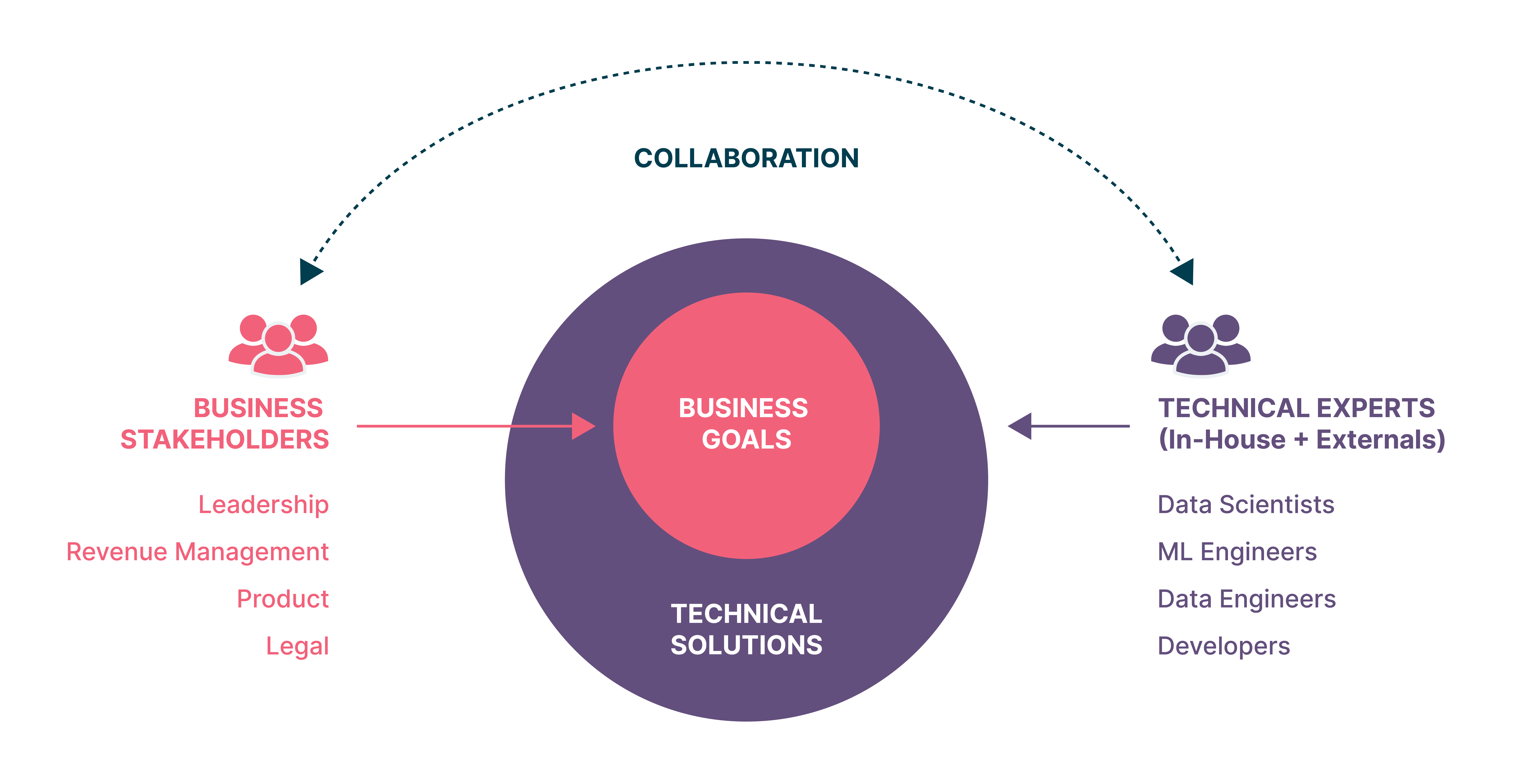
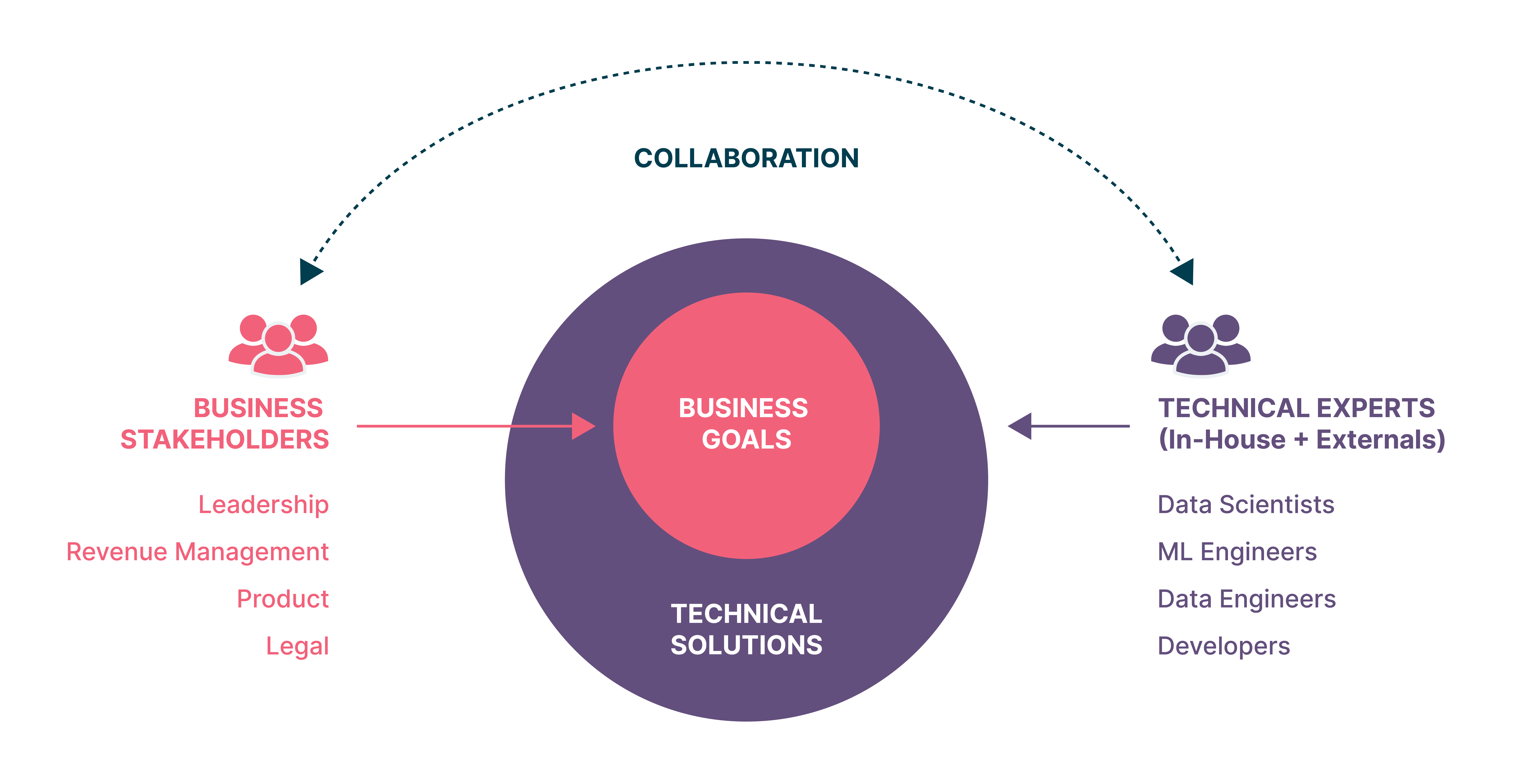
Including end-users such as revenue management and customers who are affected by the dynamic price in the testing phase of the development process is crucial for ensuring both customer satisfaction and the usability of AI-driven dynamic pricing. Understanding the user and customer profile, behavior and context is an invaluable input that helps to tailor the system to meet the specific needs of both customers and pricing decision-makers. By considering how dynamic pricing impacts customers positively, such as offering them more demand-driven and potentially cost-effective options, we can ensure that the system is not only fair but also aligned with their expectations and demands. Product thinking can also help shift the focus from just building features to understanding and solving user problems through continuous evaluation of feedback and market demands.
Facilitate adoption by effectively communicating change
Facilitating the adoption of AI-driven dynamic pricing requires robust change management. In addition to strategic alignment and involving the right stakeholders, consistent communication throughout the development and adoption stages is vital. It ensures stakeholders are aware of and educated about the implications of AI-driven dynamic pricing.
Clear communication about pricing logic enhances stakeholder understanding for the smoother adoption of AI-driven dynamic pricing. A solid understanding of pricing logic empowers informed decisions, avoiding unnecessary business rules that can override pricing. While it may seem tempting, balancing overrides is crucial for effective pricing, because excessive use may damage profitability and create maintenance bottlenecks. To enhance stakeholder understanding, use visualizations, real-world examples and transparent data based on consistent testing to communicate results and how the pricing model works. Both understanding and confidence promote the adoption of AI-driven dynamic pricing.
Clear communication about the benefits and changing roles in adopting AI-driven dynamic pricing optimizes its potential. This creates opportunities for stakeholders, such as revenue management or pricing managers, to engage in more strategic pricing activities and learn how to maximize the benefits of the new pricing system, facilitating smooth adoption.
Embrace an empirical, data-driven culture with continuous feedback
In order to unlock the full potential of AI-driven dynamic pricing, businesses must cultivate an empirical, data-driven culture rooted in continuous feedback loops. This practice is pivotal for making informed decisions that align with both business goals and customer expectations. By prioritizing data-driven insights over intuition, organizations can optimize their pricing strategies and drive sustainable growth.
To achieve this, businesses should begin with a small-scale, iterative thin-slice implementation of AI-driven dynamic pricing. This allows for controlled experimentation and gradual refinement based on data insights and user feedback.
For example, a retail company might start by applying AI-driven dynamic pricing to a small selection of high-demand products. They would closely monitor customer responses, collect feedback and analyze sales data to fine-tune the pricing strategy before implementing it across their entire product range. This iterative process allows them to optimize pricing strategies based on real-world data and user inputs. It therefore helps mitigate any risks and allocates resources effectively, ensuring that each stage of development maximizes value generation.
To refine an AI-driven dynamic pricing practice, organizations should establish continuous feedback loops, gathering insights from both internal pricing managers and external customers through methods like surveys and interviews to optimize pricing strategies that are aligned with customer expectations and market dynamics. Implementing a small-scale, iterative process, allowing for controlled experimentation and gradual refinement based on data insights and user feedback leads to a more customer- and user-centric solution that delivers maximum value.
Streamline data access, sales channel integration and infrastructure
Efficient data access is fundamental for successful AI-driven dynamic pricing. By supplying contextual data, including customer profiles, product information, cost and competitor pricing as a snapshot export to the development team, companies empower them to establish a robust dynamic pricing model from the outset. This proactive strategy expedites development, guaranteeing that initial decisions are well-informed, thereby reducing the likelihood of costly adjustments in the future.
Integrating dynamic pricing across various sales channels, particularly third-party platforms, can pose technical challenges. Given that many organizations depend on these channels in addition to their own online platform, it's important to develop a strategy for regular price updates. These platforms often require pre-calculated prices due to limited support for real-time pricing adjustments. However, updating these pre-calculated prices frequently should ensure consistency for consumers, even on platforms that may not readily support real-time pricing customization. By adopting this strategy, businesses can overcome technical limitations and still yield the benefits of the first article offered by AI-driven dynamic pricing such as boost in revenue, streamlining operations, and allowing for more strategic decision-making.
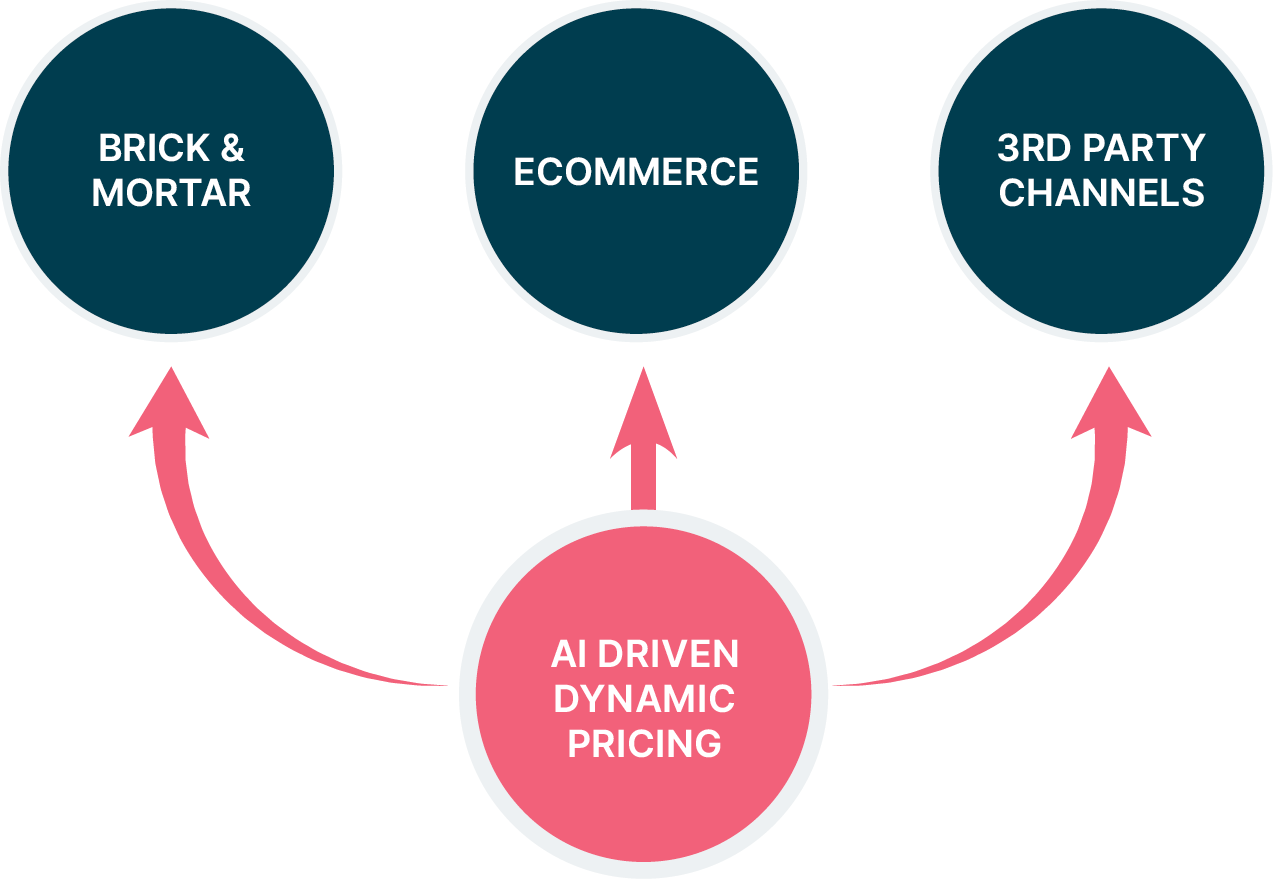
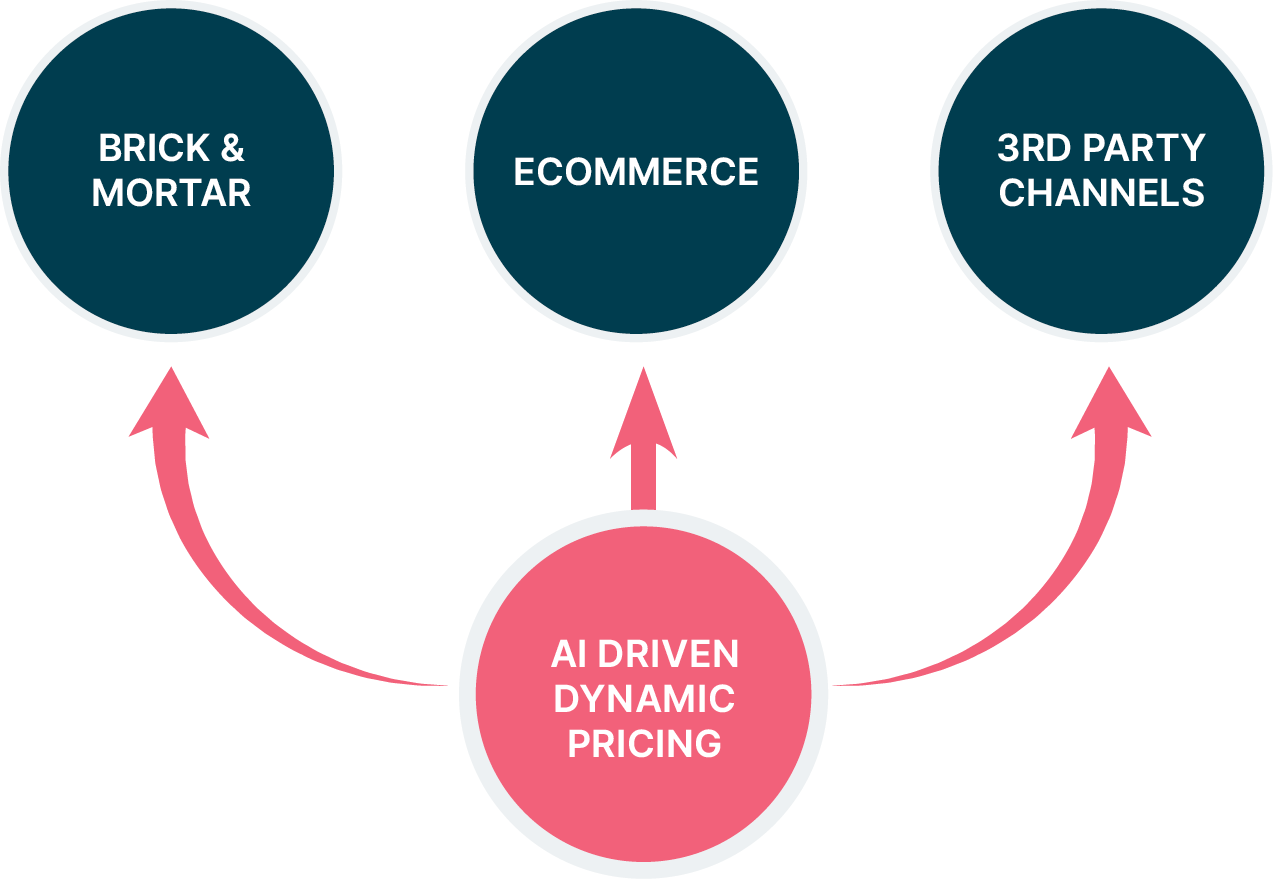
To implement AI-driven dynamic pricing, it’s crucial for organizations to invest in robust infrastructure. This involves integrating online reinforcement learning with vital data sources and sales channels. A plug-and-play approach can offer seamless integration between a dynamic pricing system and what is already in place, like your ERP and revenue management system. This eliminates the need for extensive system restructuring. Leading cloud vendors typically provide components needed to set up an online reinforcement learning system with minimal latency, which guarantees optimal performance and enhances the overall effectiveness of your dynamic pricing strategy.
Keys to success with AI-driven dynamic pricing
In summary, here are the key activities that pave the way for success and overcoming the challenges discussed in this article:
Create strategic alignment: Align the goals of AI-driven dynamic pricing with the overall business strategy, while addressing potential challenges arising from incentives and cultural barriers.
Involve appropriate stakeholders: Engage business stakeholders, end-users, customers and technical experts for effective development and implementation, establishing customer satisfaction and technical excellence.
Prioritize change management: Focus on clear communication, stakeholder understanding and an inclusive design process for successful adoption.
Embrace a data-driven culture with continuous feedback: Prioritize data-driven insights over intuition, beginning with controlled, small-scale implementation, integrating continuous feedback from customers and end-users for iterative development.
Ensure data access, integrations and infrastructure: Facilitate timely data access, and implement robust infrastructure and integration strategies, enabling well-informed decisions and maximizing benefits.
Although every organization will go about these activities in different ways, when done well they will collectively help you to drive value, align business goals and customer expectations and better leverage your infrastructure for seamless integration and optimal performance when it comes to dynamic pricing. This is no easy feat as dynamic pricing can be challenging to get right — organizations that are able to leverage AI in this space are well-positioned to seize the initiative in this field.
Disclaimer: The statements and opinions expressed in this article are those of the author(s) and do not necessarily reflect the positions of Thoughtworks.