In partnership with ‘The Modern Data Company’, we’ll share our practical experiences in delivering a rich Data Mesh experience on DataOS.
Data products are the fundamental elements of a data mesh. However, unless they’re discoverable and easy to use, their value is next to nothing. They need to be ‘sold’ in a way that meets the specific needs of their users.
But how can that be done? The answer is a self-service platform that facilitates everything a quality data marketplace needs. In this article we’ll explore what those things are, using The Modern Data Company’s DataOS as an example. Specifically, we’re going to focus on these key elements:
User experience
Product exploration
A frictionless purchase process
Support post-purchase
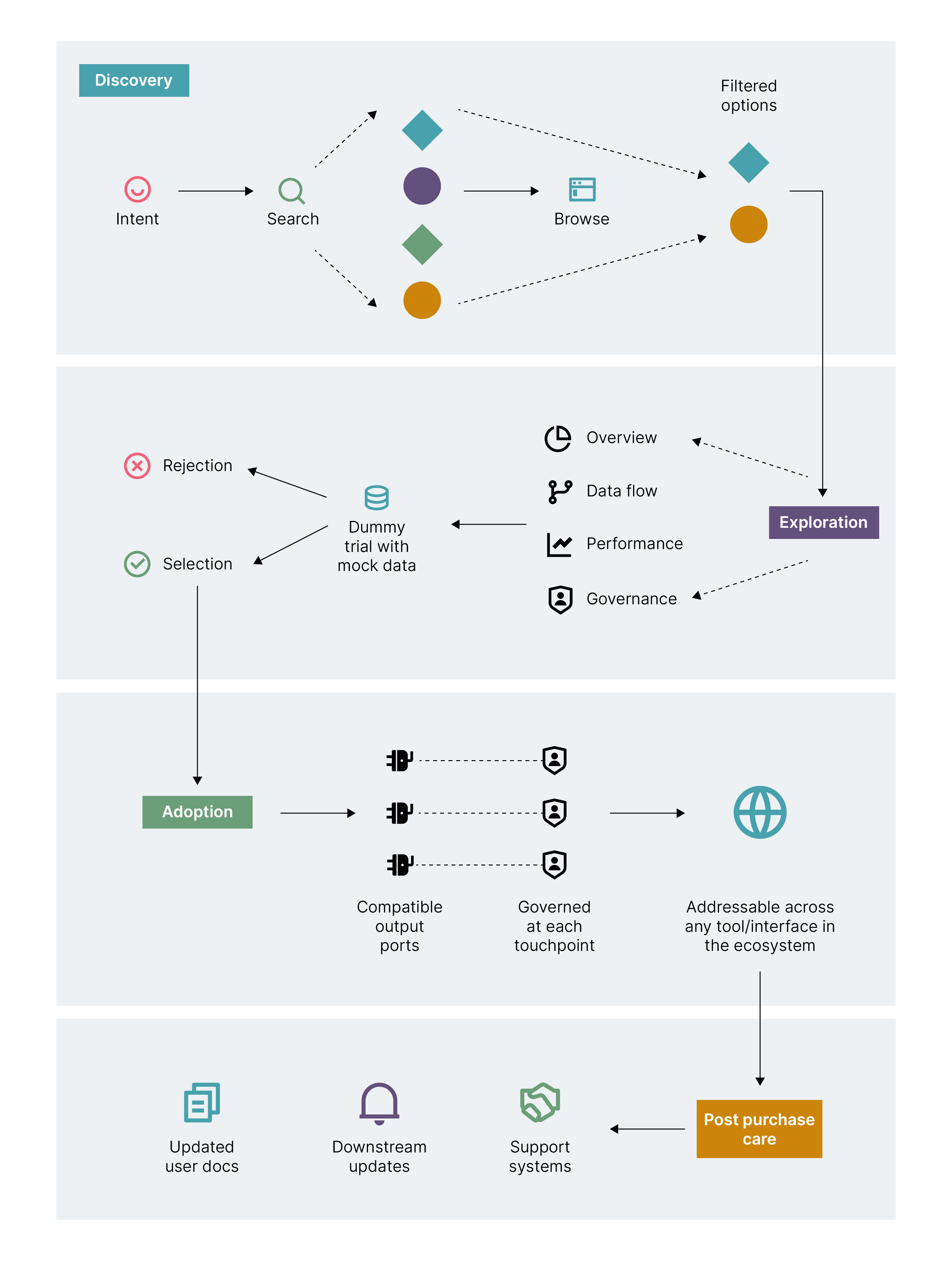
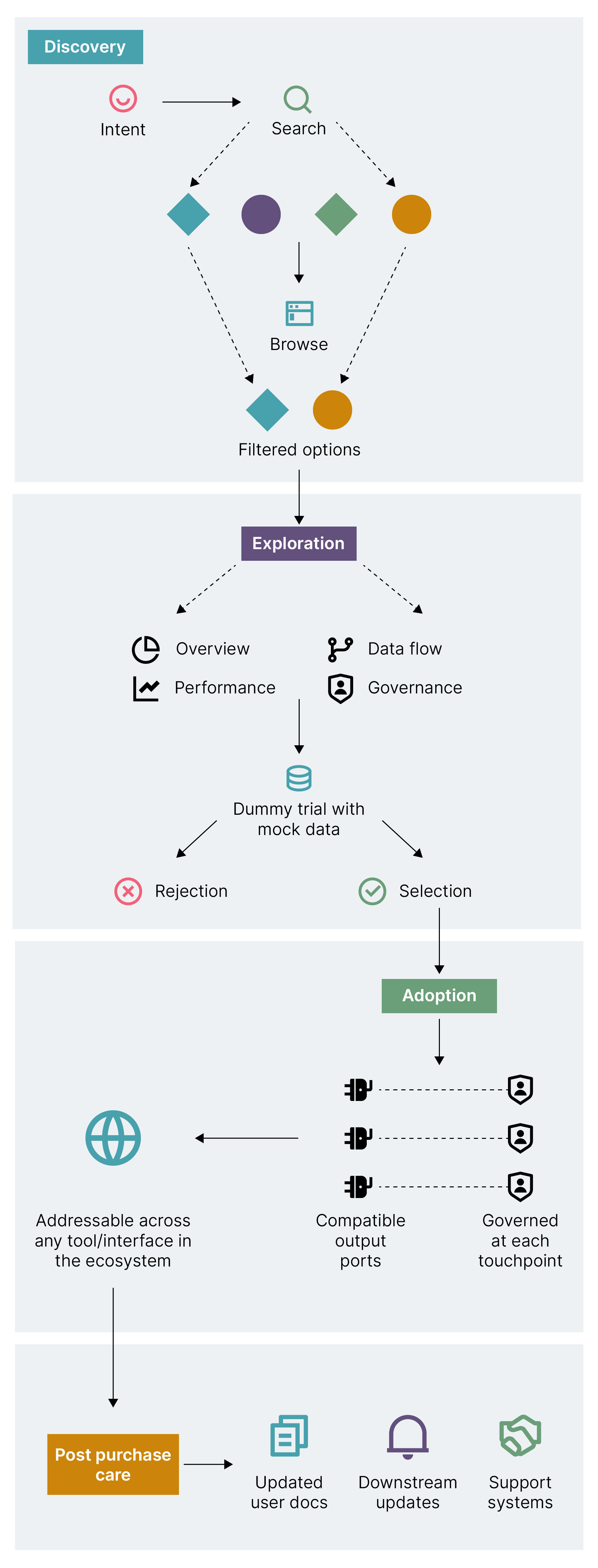
User experience
The user experience of a data marketplace is crucial. It relates to how information is presented and organized and, more broadly, how the user feels when using it — they should feel in control of what they’re doing and be able to accomplish their task quickly and easily.
This marketplace is designed to expose the right information at the right touchpoint in the user’s data product adoption journey. Of particular importance here is the discoverability of data products. In the data product marketplace, data products can be discovered either by searching (ie. entering a specific search term) or browsing (viewing what’s available and selecting from what’s displayed to you.
DataOS has an implementation of a marketplace called the Data Product Hub.
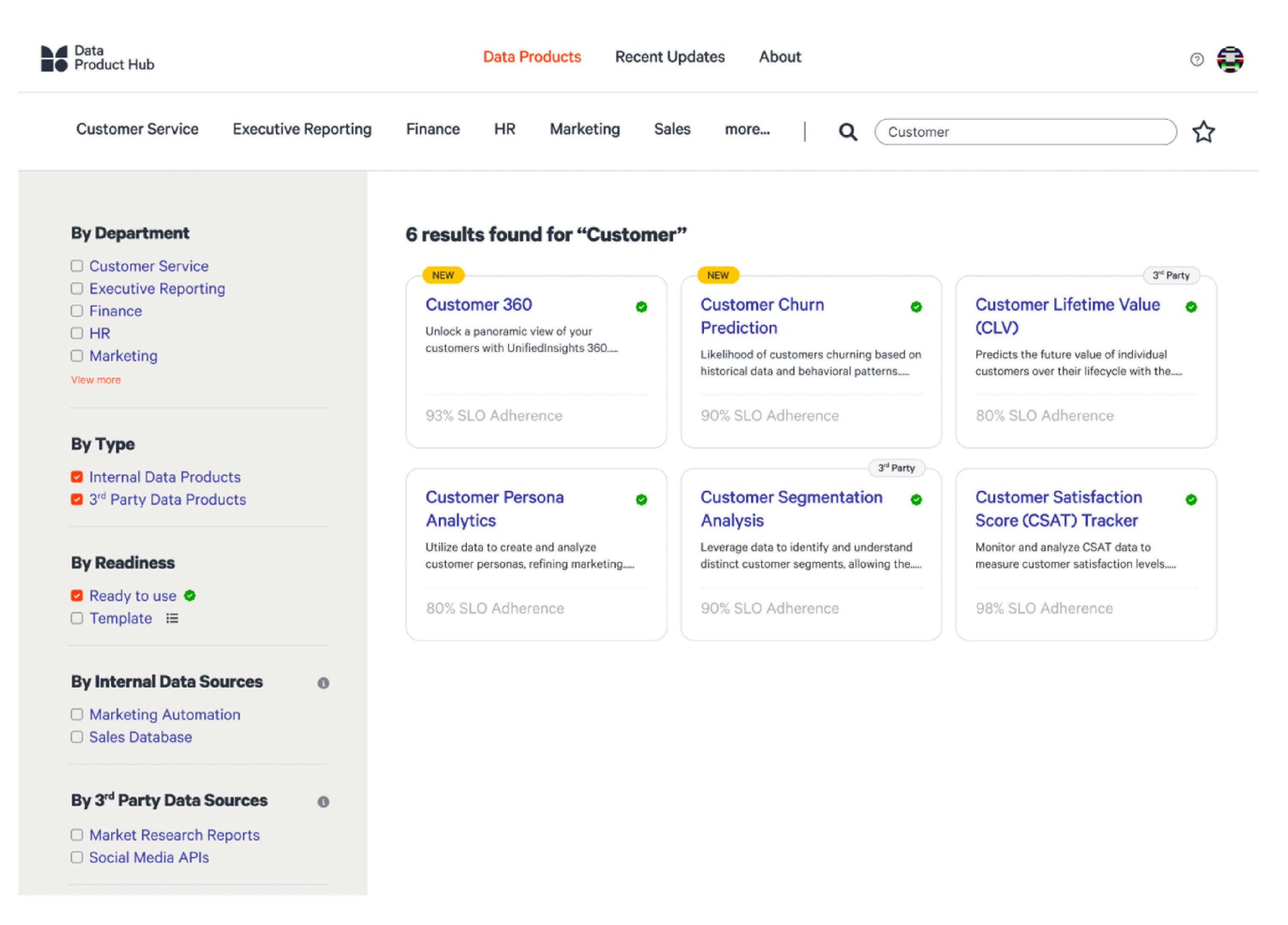
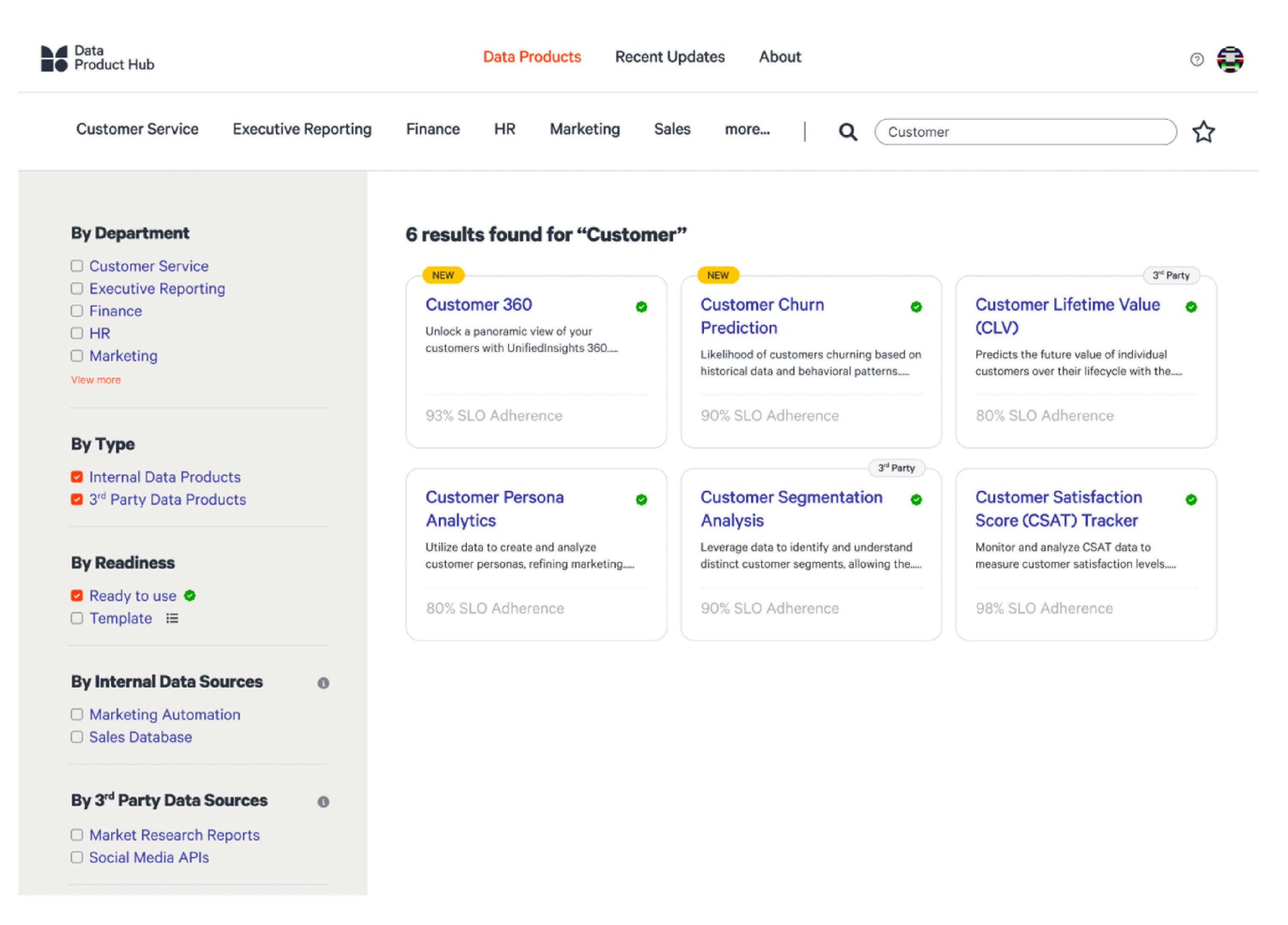
Search is used when a user has a specific query or item in mind and wants to find it quickly. For search to be effective, it needs to have a comprehensive metadata model. This should consist of precise descriptions, synonyms and tags; your data platform can manage and deliver this for you.
For instance, every time a sales representative engages in a conversation with marketing for more context and introduces a synonym as a tag for features/columns in a data asset, like "lead" for "prospect," this tag becomes part of the broader metadata model. Consequently, it becomes visible across all interfaces, products, or dashboards used by any sales personnel to access the data in that table. Similarly, adding other tags for policies, quality, and context, keeps adding to the rich metadata of the data asset, making search experiences for both sales and marketing more intuitive over time with higher usage.
Browsing, on the other hand, is used when users want to explore or discover information in a more open-ended or exploratory manner. It involves navigating through categories, menus or hierarchies to discover content or options of interest. Ideally, search is followed by browsing to narrow down the search results further using features that are layered on top of semantic information such as Filter and Explore. These filters help users narrow down their search for targeted results.
What these filters are will depend on what’s most relevant for your data marketplace; however, we think these are a good starting point:
Quality: This allows users to filter or sort based on use case requirements, such as freshness, completeness, or correctness
Performance: This allows users to sort products as per their historical performance data in past and existing use cases to understand its adoption
Downstream impact: Allows users to explore and analyze the downstream impact before taking decisions around the product
Accessibility: Filter for the access mechanism to serve the different consumer personas
Ownership: Search by product owners to make additional queries or requests for product enhancements
Product exploration
Discoverability — whether through search or the browsing functionality — is an important first part of finding the right data product. However, users may need to take a more in-depth look at a given data product to determine whether it meets their needs for a specific use case.
Product exploration provides a cross-sectional view of the infrastructure, code and data layers of every product that the organization owns. Indeed, the UI design — discussed above — is important here. In the context of product exploration, below are different interactive views that are offered to a consumer
Product overview. This summarizes a host of information in a compact way so users can consume it at a glance. It includes high-level elements around governance, ownership and data flow. It also provides information on service level objectives and potential use cases.
Data flow/lineage. A quick 360-degree view of the end-to-end journey of data through the product.
Data product SLO adherence. This gives an insight into how the data product is delivering according to business goals and objectives.
Data product policy view. This includes information about what can be accessed and any associated policies users need to be aware of in relation to the given data product.
Documentation and provenance. Allows the user to explore the data product in greater depth.
If you’re a marketing analyst, for instance, you may want to identify which customer segments will respond better to certain campaigns — this will help you devise new and effective ones. You might begin by exploring data products related to campaigns to assess their suitability; by examining descriptions, tags, lineage and past usage, you can narrow down your options. To enhance their confidence in the choices, you might even review the data's service level objectives (SLOs), to increase your confidence in a given data product. Lastly, if your report or use case involves sensitive information, easy data product exploration makes it possible to review the relevant governance protocols attached to a data product.
To further deep dive into data, it should also be possible to spin up dummy use cases. These can show users how a product works and what it can do, discover helpful patterns for use to make it easy to apply to real-world scenarios. Success stories from other users can also prove very helpful in a data marketplace: combined, these features help users decide what data product is right for them and to begin using it quickly.
In DataOS, there are different interactive views for product exploration. Take a look at the screenshot below:
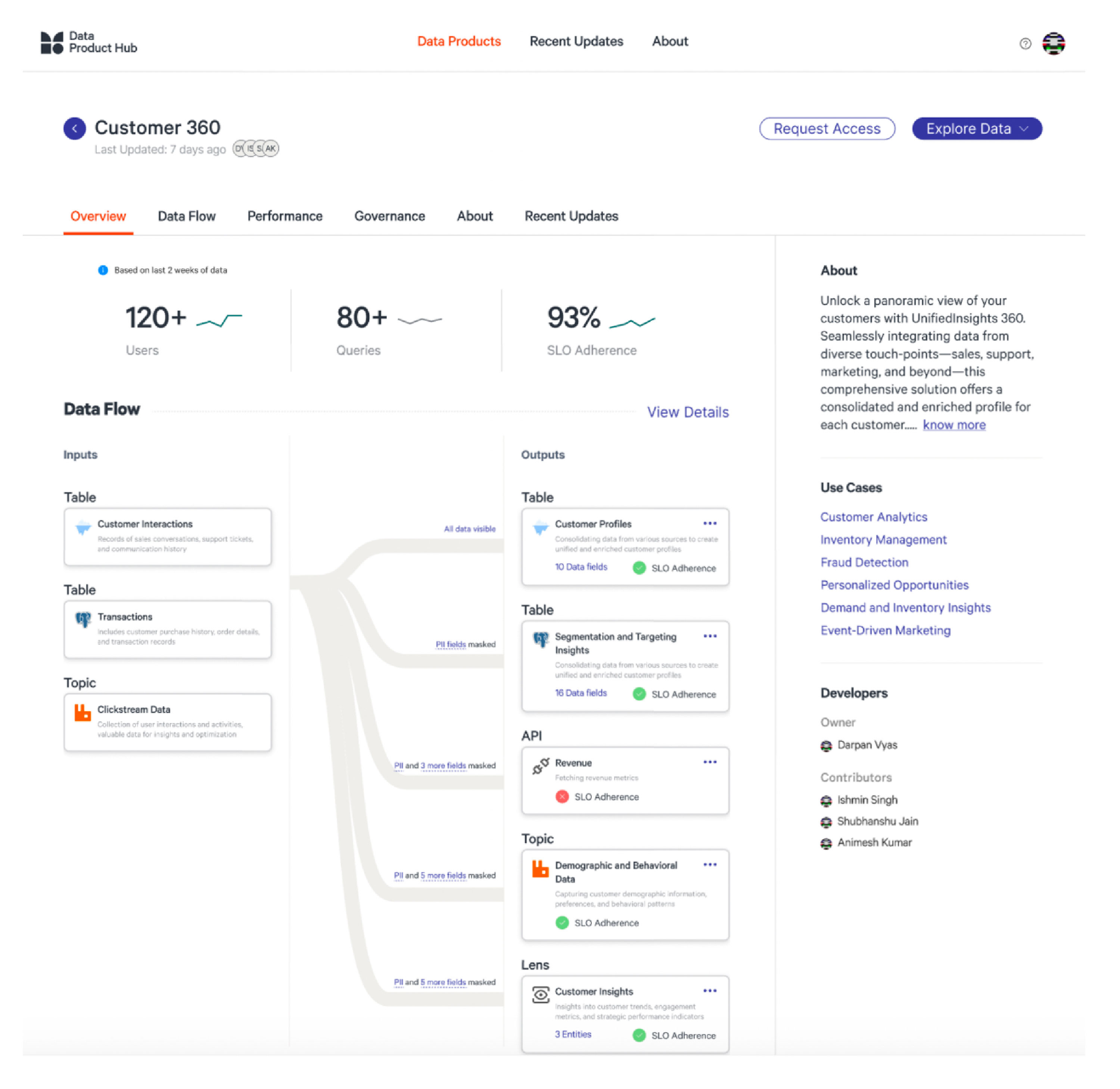
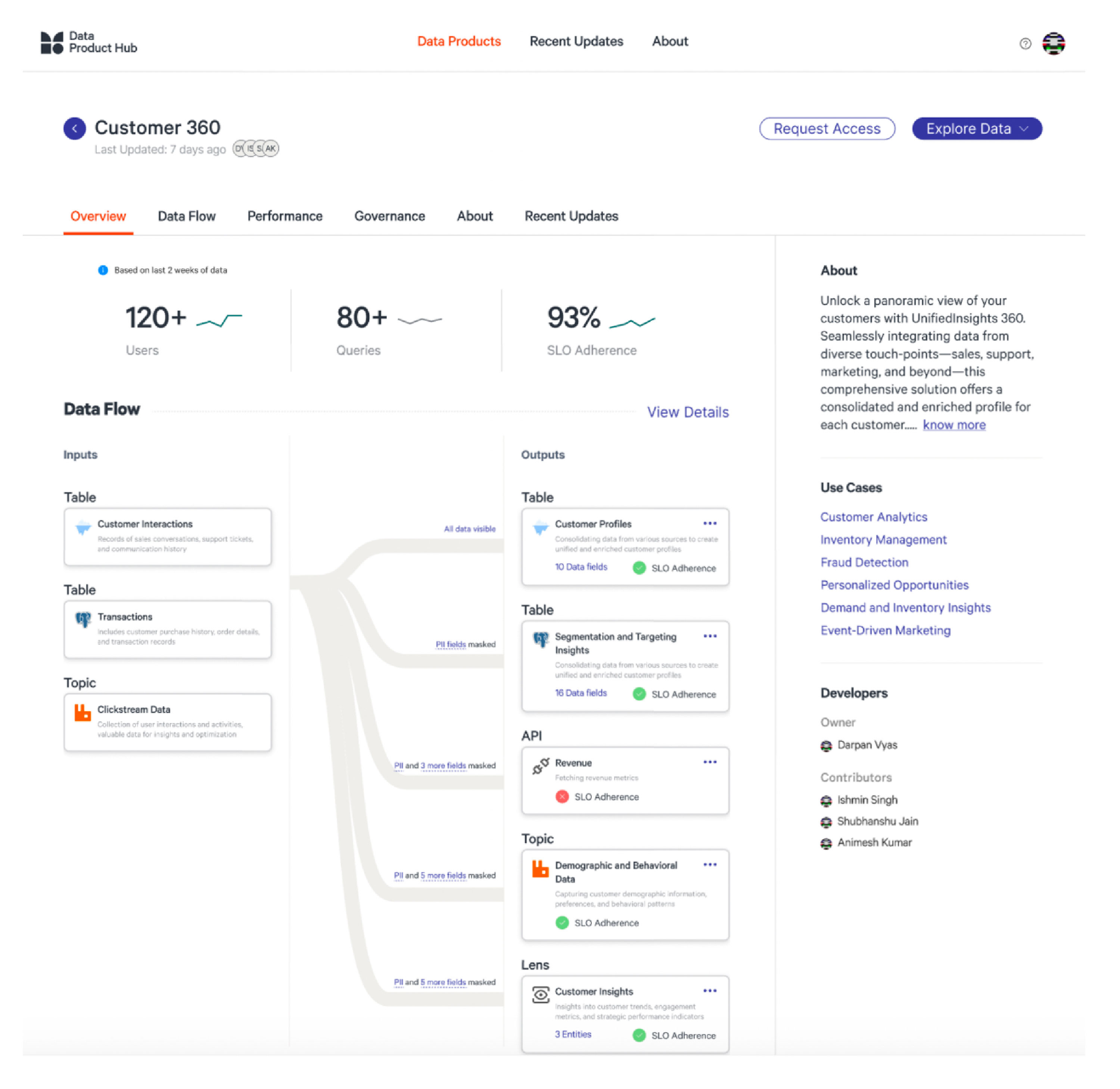
A frictionless data product purchase process
Once a data product has been chosen, that certainly isn’t the end of the story. A complex and messy adoption process can be frustrating and undermine the potential value of the data product. Some of the common points of friction in the data product ‘purchase’ process include long queues of requests, incompatible data formats or outputs and incomprehensible data.
A self-service platform can do a number of things to help users during this phase.
Compatible output ports. All output ports should be available for the user to pick from along with natively accessible output ports such as SQL. The platform provides a standard interface to develop a wide range of output ports, as required by consumers of data products across domains.
Access: Imagine a marketer needs access to data provided by an upstream source, like customer profiles, to better understand their target audience. The process of gaining access can be time-consuming due to verification and implementation protocols. The self-serve platform should enable quick and easy access through declarative policy-driven methods. Access requests can be self-initiated, or automated workflows can be triggered with human involvement. The data product marketplace, by virtue of the data product anatomy, can implement policy models across data and infrastructure layers through simple semantic calls such as user and masking tags.
Surface relevant metadata that catalyzes the “buying” decision. There should always be enough information for a user — this keeps the data product exploration stage short.
Quick integration with the required application(s). Every asset and resource in any data product on the data products marketplace is universally addressable. In other words, any user can refer to the required asset, resource or the entire data product to start consuming quality approved and governed data. Once the user has been granted the required access to the data product, they can refer to the said asset from any approved tool or interface to start integrating the product with their apps/projects.
Of course, every use case will be different — users will want, need and expect different things from a data product. However, a considered and effective data product platform ensures that the process is smooth, with minimal friction.
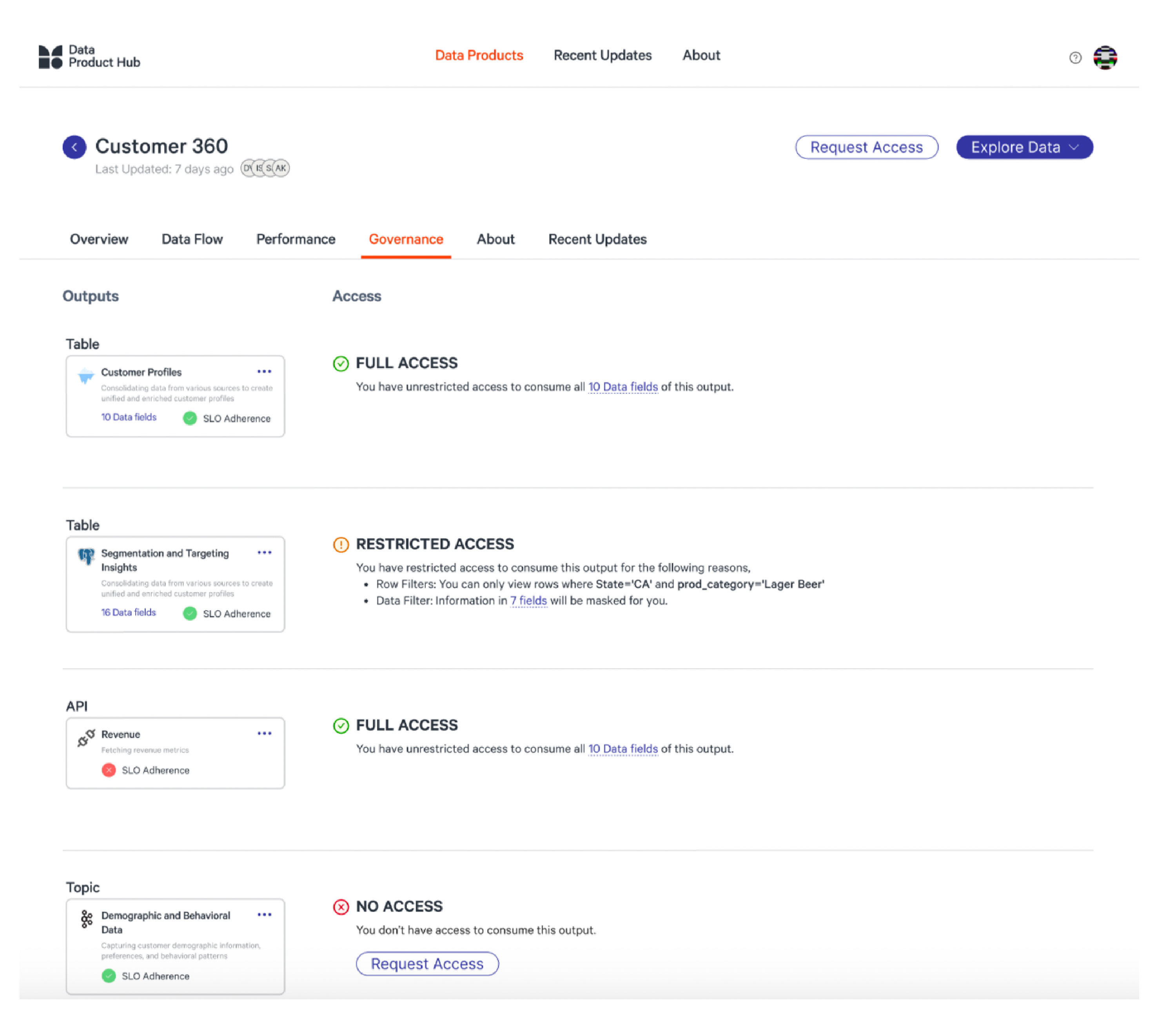
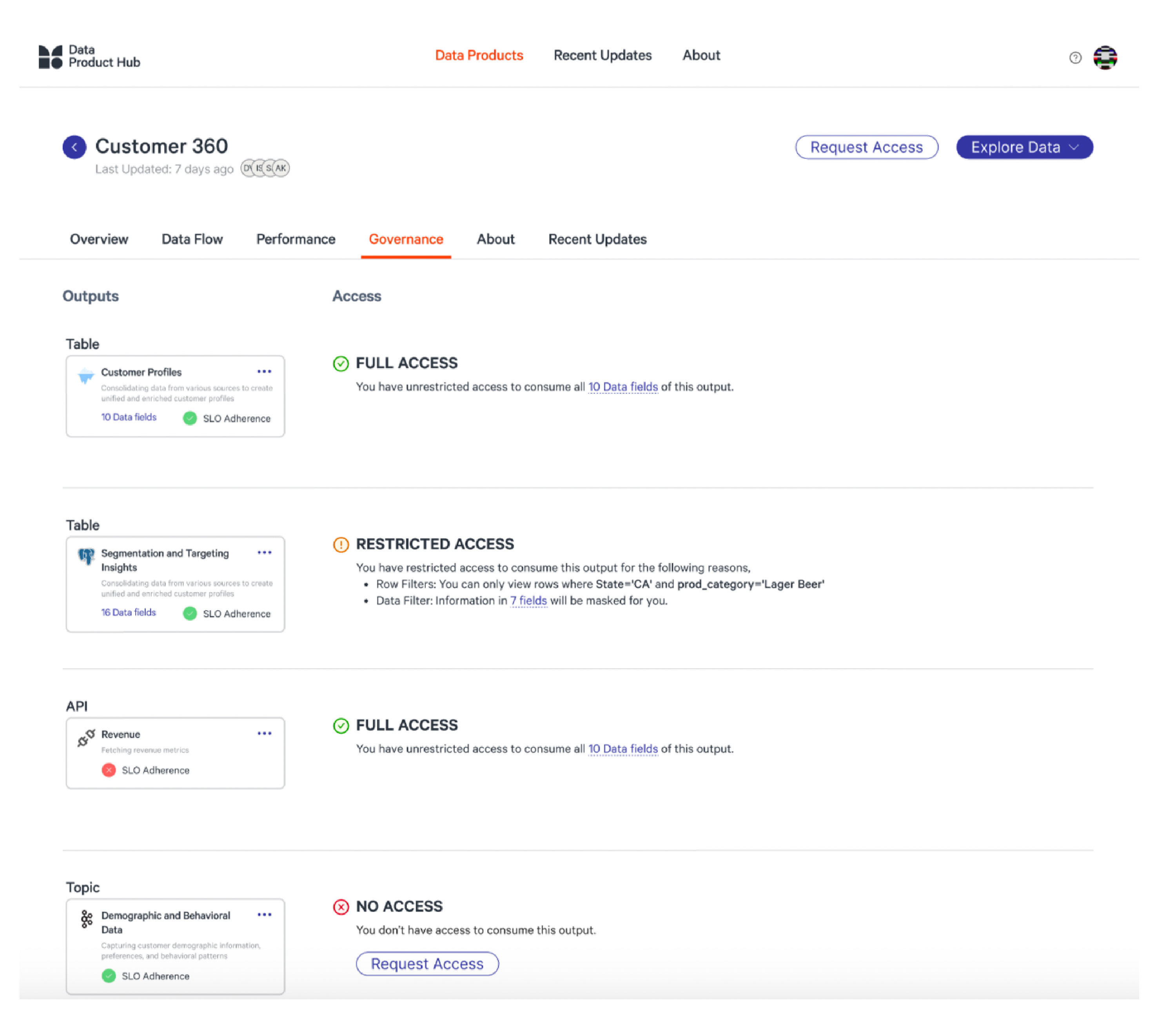
Post-purchase care
We often talk about post-purchase care in relation to certain consumer goods — particularly complex technical products like laptops. However, the concept is one that’s useful when thinking about data products in a data mesh. Users shouldn’t simply be expected to get on with it and left to their own devices. Support systems, downstream updates, up to date documentation are all essential in the post-purchase phase. These all help ensure the data product can deliver value over time, and that any issues a user might face can be dealt with quickly.
Trust and transparency empower users
Data products aren’t, of course, consumer goods. But if we are to really embrace data product thinking, users need to be treated like any other customer: their journey from discovery to adoption needs to be well supported. Indeed, the benefit isn’t just to the individual user — it helps to embed a culture of trust and transparency which is essential for data mesh to be effective. A Data Product Marketplace can facilitate organizational change by empowering individuals to make informed decisions about data products quickly and easily.
Disclaimer: The statements and opinions expressed in this article are those of the author(s) and do not necessarily reflect the positions of Thoughtworks.