Looking Glass 2024 coming in January
Partnering with AI
Making machine intelligence mainstream
The AI achievements appearing across the industry are nothing short of astonishing: just look at Google Deep Mind’s projects to predict 3D protein structures and control fusion reactors, or NVIDIA’s system to instantly create 3D photos based on 2D objects. But what’s really interesting is how AI and ML innovation is ‘trickling down’ and is now in the reach of the rest of us. Never before have these fields exhibited so much potential or mainstream applicability. Businesses are using AI solutions to scan physical receipts, detect fraud, recommend products to customers and even turn interactions over to synthetic spokespeople.
We see AI and ML becoming even more accessible, more embedded in the business — and more productive. Cloud vendors such as AWS are now offering data management tools and pre-trained ML models that can feasibly provide any company a head start in creating value through ML. Some vendors even offer AI services where businesses can simply feed data into a ready-to-use model to get an answer or outcome.
The growing emergence of ‘off the shelf’ solutions has the capacity to level the playing field, and make more and more solutions AI or ML-enabled. Nonetheless, the companies that do AI/ML best, and with the highest-quality data, will retain an advantage. That makes it important to continue to strive to develop AI/ML knowledge, capabilities and talent.
In the short time since publishing this edition of Looking Glass, tools like ChatGPT have taken the world by storm, providing further evidence that AI is becoming more mainstream. Its conversational style and back-and-forth between humans and the AI also showcase the ‘partnership’ approach for AI. ChatGPT and related technologies are deeply nuanced, and throw up many as-of-yet unresolved questions. See here, for an in-depth exploration of these issues.
Signals include:
A rapidly expanding enterprise AI market. Gartner predicts the market for AI software will approach US$135 billion by 2025, with growth more than doubling to 31% — a considerably faster pace than the software market as a whole.
Consumer products incorporating AI as a matter of course and without much fanfare. AI-powered features that might have generated significant excitement just a few years ago are now standard issue in devices like Apple’s iPhone, which uses AI to detect, sort and enhance images — often without users appreciating the clever underlying technology.
More organizations appointing chief data officers, and in some cases chief analytics officers (or chief data analytics officers). According to recruitment specialist Korn Ferry, only a decade ago barely 10% of big firms had such roles; the rate now among “data-intensive” companies is closer to three-quarters.
AI leaders like Meta disbanding dedicated AI groups in favor of embedding AI experts in product teams or other parts of the enterprise. This points to AI becoming an integrated part of everyday business operations, rather than a specialized, stand-alone practice.
Creative professionals are taking AI tools much more seriously and using them as time-saving helpers to create illustrations, art, articles, blogs and emails, and even program code.
The opportunities
Using AI to generate real business value. With AI and ML now in easier reach for many organizations, and more companies able to embed AI/ML capabilities in operations or products, there are a multitude of paths to explore. There are different ways to get value from AI and ML. For example, companies are applying AI to improve efficiency, ultimately reducing costs. In some cases AI is even being harnessed to create entirely new, award-winning products, by augmenting human creativity and decision-making. In other cases, ML is being used in much less dramatic ways to remove friction from user experiences.
Unlocking untapped innovation capacity by freeing up people to focus on the big picture. There’s an unfortunate but still common perception that adopting AI/ML inevitably means automating away jobs. But when applied in the right way, AI/ML reduces the amount of time people need to devote to mundane tasks, giving them room to focus on higher-value functions. By contributing to business and customer knowledge, AI can provide additional insights that pave the way for productive decisions and greater customer delight. The real goal of an AI/ML implementation should be to support and augment human intelligence, not render it obsolete.
Answering the big questions — everywhere. The sheer computational power and algorithmic understanding being offered to organizations today means they can solve problems that were previously insurmountable. Critically, these algorithms can be run in more and more places. Cellphones with embedded AI hardware can be as powerful as a desktop computer. Edge devices with constrained processors can use TinyML techniques to run AI almost anywhere, without even needing network connectivity. All this creates unprecedented potential to draw and analyze data from different sources to generate insights and inform decision-making, wherever that needs to happen.
Answering the small questions — at scale. For some decisions, it’s possible to take humans out of the execution loop and provide fast responses at scale. For example, using dynamic pricing to boost hotel room occupancy. With this style of AI, humans are still involved in monitoring the results to ensure the system remains within intended parameters.
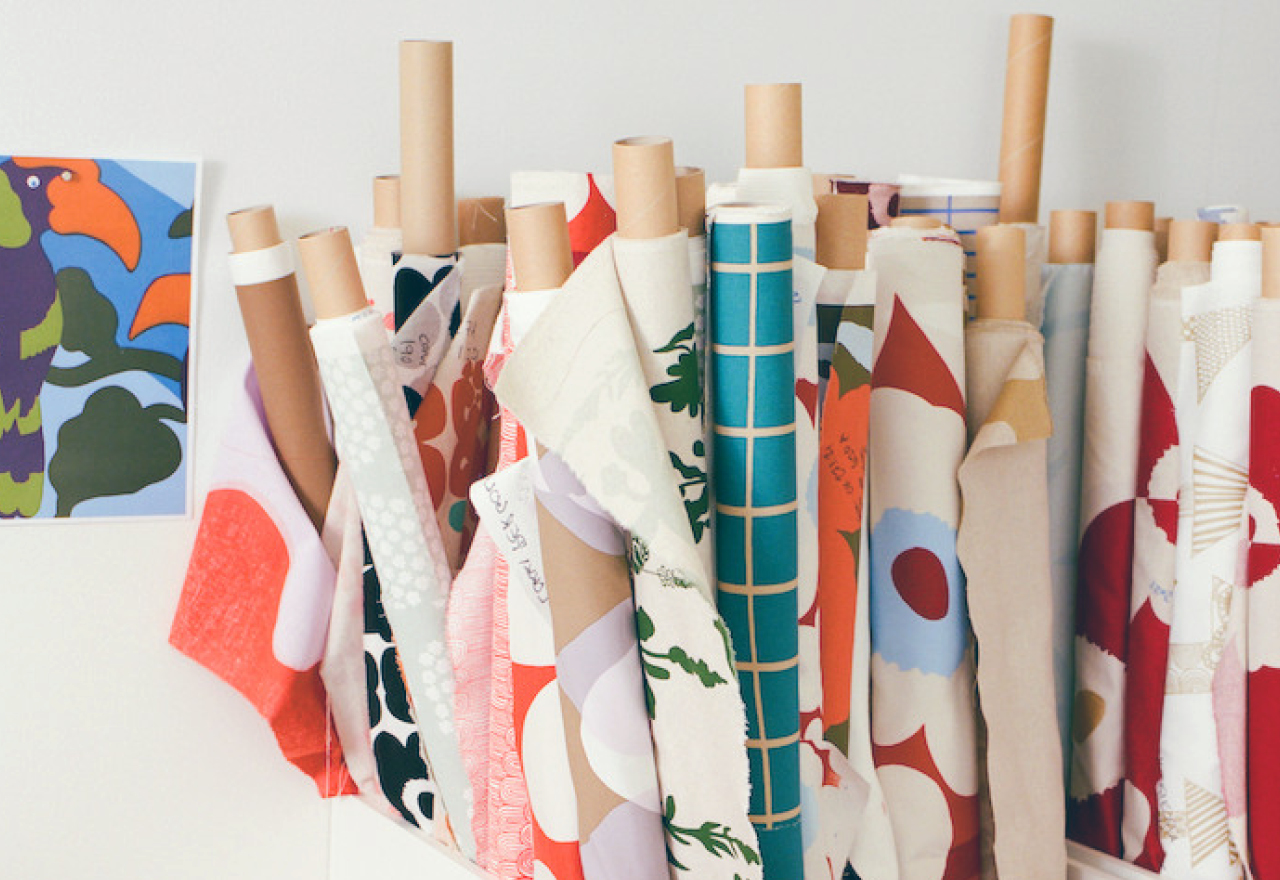
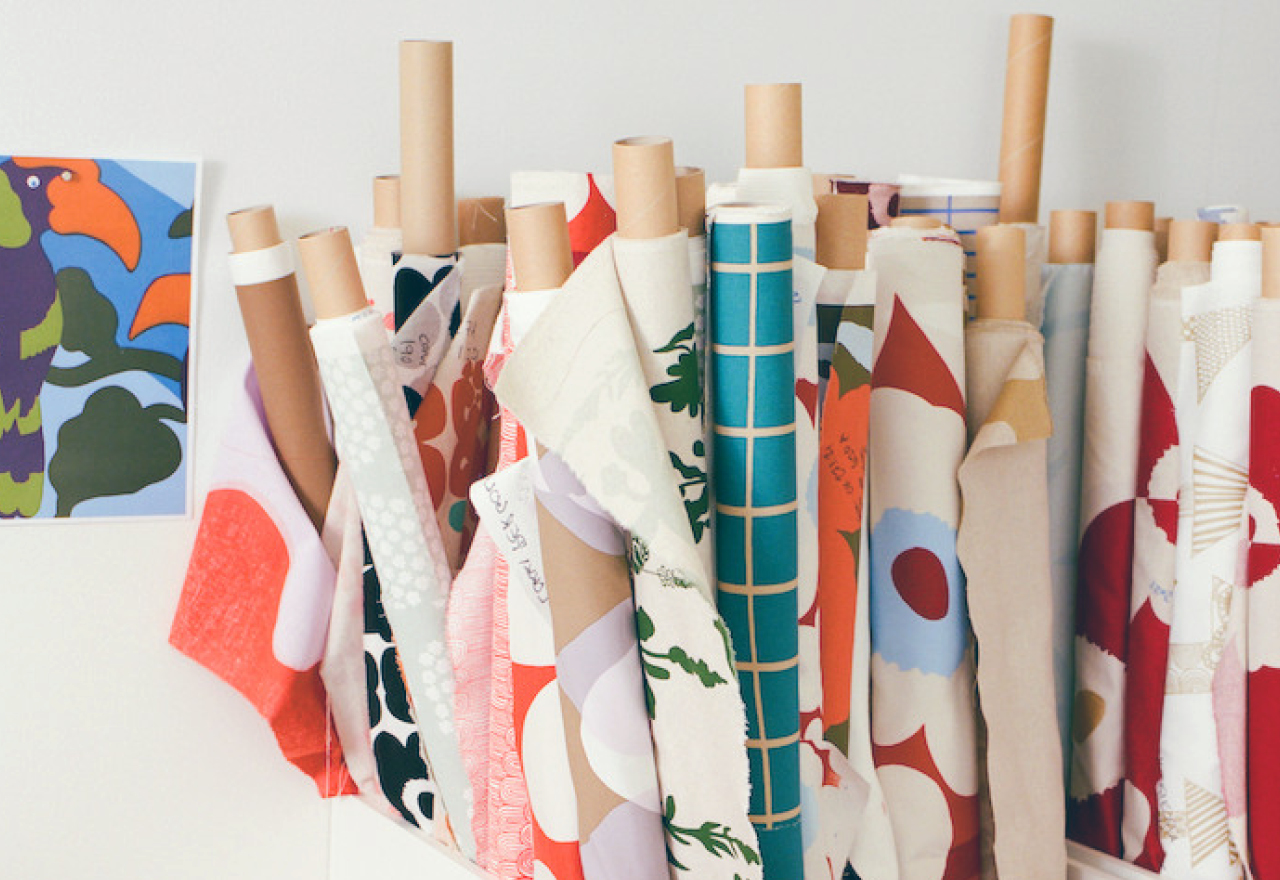
What we’ve seen
Maripedia enables users to upload photos of patterns with special meanings to them, such as prints cherished by a parent or grandparent. Then AI-powered image search analyzes the photo, compares it to digitized samples of thousands of Marimekko's patterns, and finds the closest match. Maripedia reveals the stories behind each historical design but also allows customers to find current products featuring similar fabrics. The experience provides a fascinating peek behind the scenes of Marimekko’s art of printmaking from the early 1950s to the 2020s, and gives the company new insight into what customers are interested in today. When customers are attracted to a particular historical print, Marimekko can act on this insight to create new products and stay ahead of trends and fashions.
Trends to watch
Adopt
ML platforms. A solid ML platform provides a sound base for the entire ML lifecycle, from ingesting data to readying it for use in models and monitoring the results. This can substantially enhance the productivity (and satisfaction) of data analysts, who no longer have to be concerned about the more mundane aspects of making data fit for purpose. ML platforms also pave the way for more consistent, effective ML software, enabling the organization to put its best foot forward with automation.
Analyze
ChatGPT and large language models (LLMs). There’s intense interest in these technologies, which at first glance seem to have made remarkable advances in the capabilities and ‘intelligence’ of AI chat bots. But looking deeper, the technology has problems: an information cut-off date after which the bot knows nothing about current events or technologies, biased responses driven by skewed training data, and a propensity to confidently give wrong answers about just about every topic. While experimentation is appropriate, companies should fully investigate when and how ChatGPT and related technologies can be used within their organization and provide staff with clear guidance.
Anticipate
AI safety and regulation. As the ethical challenges created by AI become more apparent, governments and regulators are taking steps to develop rulebooks and codes of conduct that enterprises looking to apply AI/ML will have to take into account. Early examples include a roadmap for an AI assurance ecosystem that will form part of the UK’s National AI Strategy, the AI Bill of Rights unveiled by the White House’s Office of Science and Technology Policy, and the EU’s AI Act.
Trends to watch: the ones we're seeing today
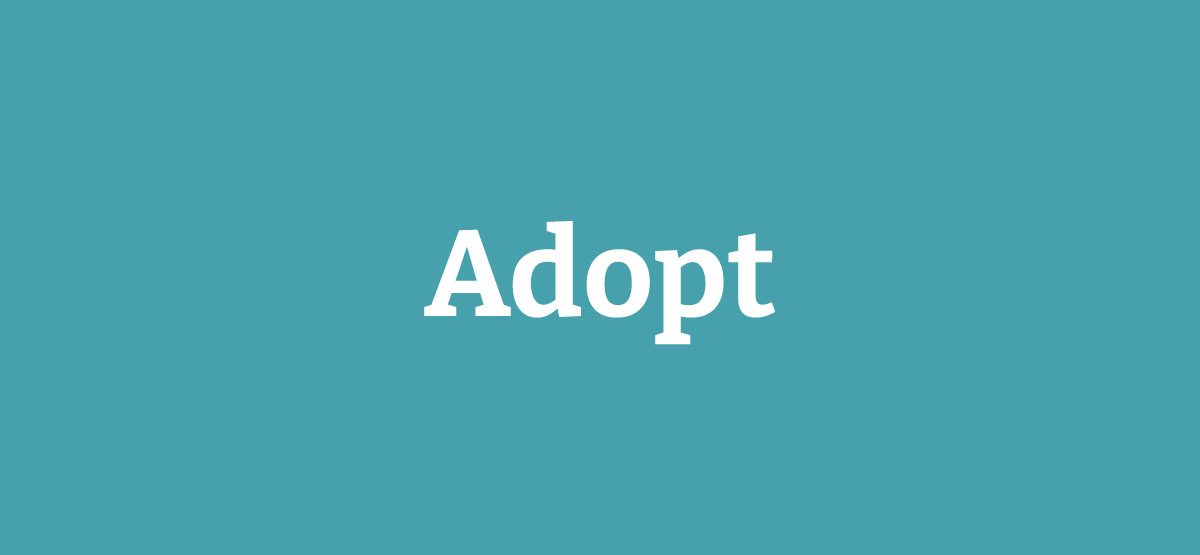
- Agent-based simulation
- AI as a service
- Digital twin
- Explainable AI
- Green software engineering
- MLOps
- ML platforms
- Natural language processing
- Online machine learning
- Operationalize AI
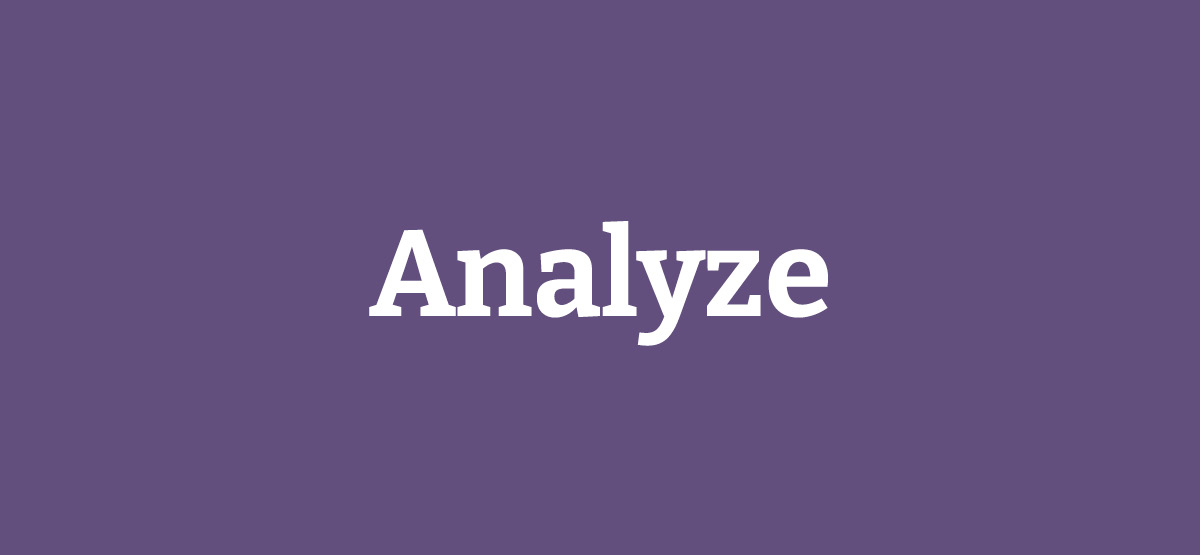
- AI-assisted software development
- AI-generated media
- AI marketplace
- AI/ML on edge
- AutoML
- Autonomous robots
- Autonomous vehicles
- ChatGPT and large language models (LLMs)
- Code of ethics for software
- Ethical frameworks
- Personalized healthcare
- Privacy-enhancing technologies
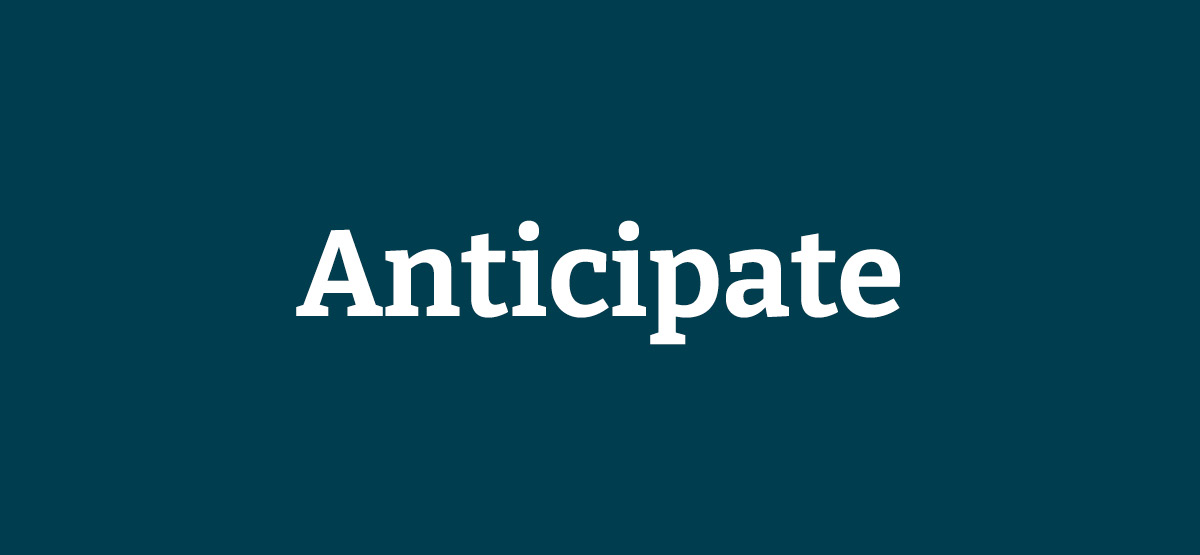
- Encrypted computation
- Smart cities
Advice for adopters
Ensure AI and ML are part of your enterprise toolbox. DeepMind has people looking at the world’s most complex algorithmic problems — protein folding, controlling fusion reactor plasma with magnetic fields — and asking “could we solve this with AI?” The business world needs to adopt a similar approach when confronting more mundane challenges. Take steps to raise awareness of the range of AI/ML tools and services becoming available, so when your people face a problem they consider bringing these solutions to bear. In general ‘off the shelf’ AI services can address undifferentiated problems, such as image recognition or speech-to-text, whereas customized models are more appropriate in cases where your data will be very different from that of competitors, such as customer churn and sales forecasting.
Support your ML efforts with an underlying data platform or data mesh. Even if you have the same model or AI product as your competitor, good data, and the ability to harness it effectively, can be a massive differentiator. For example, if you use an AI service to predict customer demand for your products, providing higher-quality historical data over a wider range of data points will give you more precise answers, even if a competitor is using the same cloud AI service. Better data can be the difference between effective or ineffective use even of packaged models, and good data platforms will enhance the productivity of your people.
Avoid establishing AI ‘centers of excellence’ or simply telling your team to ‘go do AI.’ All this does is give the impression of AI as something that stands apart, when what’s really needed is guidance on the right model for infusing AI across the organization. Much like security or DevOps, AI works best when practitioners are working directly with people across the enterprise, helping others learn.
Recognize that AI/ML systems will face an adoption curve and possible lack of trust, beyond the challenges of operationalizing AI from a technical level. Slicing implementation into small experiments on impactful projects can minimize the chances of massive disruption while helping build comfort and buy-in. Advances in explainable AI and privacy enhancing technologies can help increase trust over time.
Strive to measure the impact of AI/ML-infused products and services. Measuring value is always difficult, but it’s important to at least try to demonstrate there’s a clear business case for change. Estimate the extra value created by using AI and ML over simpler software with if/then/else logic, or the value created by freeing up people from manual tasks and allowing them to think about more complex problems.
Remember AI also has negative manifestations, and consequences. Along with the justifiable concerns about the rise of AI-powered surveillance and inherent bias in algorithms, there are growing concerns about AI solutions facilitating digital addiction. Training large models can also consume large amounts of energy, running counter to sustainability efforts. It’s advisable to remain sensitive to these concerns, and think through the possible unintended consequences of applying AI to a process or business goal, not just for reputational and regulatory reasons, but because it’s the right thing to do.
Be open-minded. Be prepared for AI to lead you to learn things you weren’t expecting to learn, or towards an approach that you didn’t necessarily consider originally. At the same time, because people sometimes call things AI that aren’t in fact AI, and claims are made about AI that are hard to verify, maintain a degree of skepticism and recognize AI/ML is not the answer to every business problem.
Understand that most of the AI technologies you hear about in the press — especially ChatGPT — are tools for experts. ChatGPT has a tendency to fabricate plausible sounding answers that are factually incorrect, so is best used by someone who already knows what a ‘good’ output looks like. We expect this to improve as the technology is developed and better training data is fed to the bot, but the point remains: organizations should carefully monitor and verify the outputs before relying on them. We’ve written about this in more detail in our article ChatGPT: A useful tool buried beneath the hype.
The rise of machine learning and AI continues to impress me. AI can now solve hard problems like protein folding, controlling plasma within fusion reactors, and creating art from text. But don’t let these fancy use cases distract you – AI and ML usage is becoming more mainstream, providing value across all sectors and industries.