This is the second article in a series exploring how artificial intelligence (AI) can deliver significant value to the financial services (FS) industry. You can read part one here, where we explored solutions within private banking and wealth management.
Capital markets are a critical part of a well-functioning economy, but firms face a range of challenges. The pressure is on firms to show that they are sustainable and have solid environmental, social and governance (ESG) credentials - this is no longer a ‘nice to have’, but a must-have to continue operating. The volume of regulation is only increasing following the financial crisis, with recent waves targeting the sustainability agenda. Margins are under pressure, yet the business and operating models are not set up to generate value from the exploding volume and variety of data that becomes available. All of this is compounded by rapidly changing customer expectations. For the multitude of players involved, on both the buy side and sell side, there are testing times ahead.
AI may not provide an instant solution for all the headwinds facing capital market players. However, if successfully deployed AI can help firms address many of the issues, even with the constraints posed by complex legacy tech and data stacks. The extent to which AI can aid firms has been masked by the narrow focus to date on automating repeatable processes. This has left untouched many opportunities to accelerate the development of innovative products and IP, which are critical in the digital age.
We have identified four promising areas where AI can be applied to address complex timely topics for many capital markets participants, and we offer advice based on our experience on how to drive AI value realization within large organizations.
Environmental, Social and Governance (ESG)
Sustainability has dramatically grown in importance in recent years - becoming a differentiator for asset managers, a major consideration for capital raising, and a factor in corporate valuations. There is also an increasing regulatory focus with the introduction of mandatory disclosures across the world (e.g. SFDR in Europe) and the first cases of ESG misstatements under the spotlight with fines for BNYM and Goldman Sachs.
The problem with ESG is a lack of reliability, comparability, and transparency around relevant data. The Economist (2022) research found that of the six ESG rating agencies (fundamental sources of ESG data for FS firms), they used 709 different metrics across 64 categories, and only 10 categories were common to all.
A major challenge is around identifying, enriching, and interpreting vast troves of data a typical financial institution needs for its ESG objectives. Some of the data used consists of analysis provided by rating agencies, specialists (e.g. Factset TrueValue, Equileap), and NGOs active in sustainability (e.g. WEF), emissions data on supply chains and corporate tech stacks (The Conversation, 2022), as well as unstructured data such as satellite imagery (see figure below). Regardless of the source, data will inevitably vary due to the different assumptions providers make on the underlying issues. Moreover, while emissions data of varied quality is supported by multiple providers, social and governance metrics are harder to collect and standardize.
Without common standards in place, it's up to the individual financial institutions to develop solutions that differentiate them from competitors while creating trust in the ESG indicators they provide for firms they track or finance.
Deep learning techniques can be used to triage complex ESG data sources, extracting the key indicators from various sources. Once obtained, AI can be applied to consolidate similar signals, verifying and enriching the information while reducing noise. The final step of converting into meaningful ESG scores will require human judgment, supported by AI employed to track and identify the warning signs of deteriorating ESG metrics, in real time.
Problems with ESG data: Numerous data sources of varied quality, heavy reliance on publicly available data and lack of transparency on methodologies and metrics used
ESG rating agencies - widely different and uncorrelated scores across rating providers, little clarity on definitions, ambiguous methodologies, metrics & weightings
Publicly available data - not always independently verified information. Quality & data availability across geographies vary
Specialist data providers - primarily rely on publicly available information. Assumptions made in models that aggregate the data are not disclosed and vary significantly
Alternative data sources - used to a limited extent if at all at present
Company data (not public) - a most reliable source of data, e.g. for Scope 3 emissions, labor conditions in 3rd countries. Not available to the data providers and rating agencies
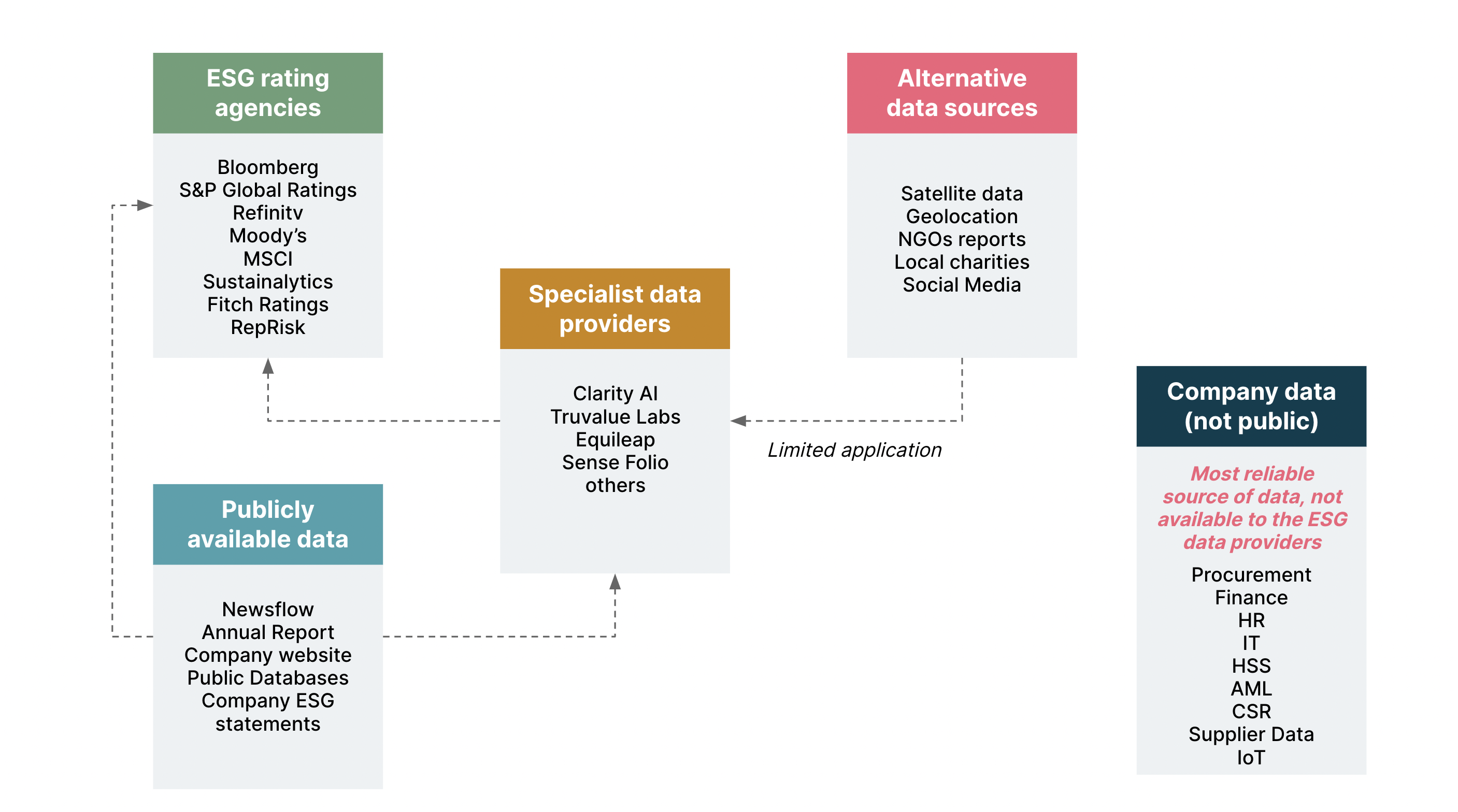
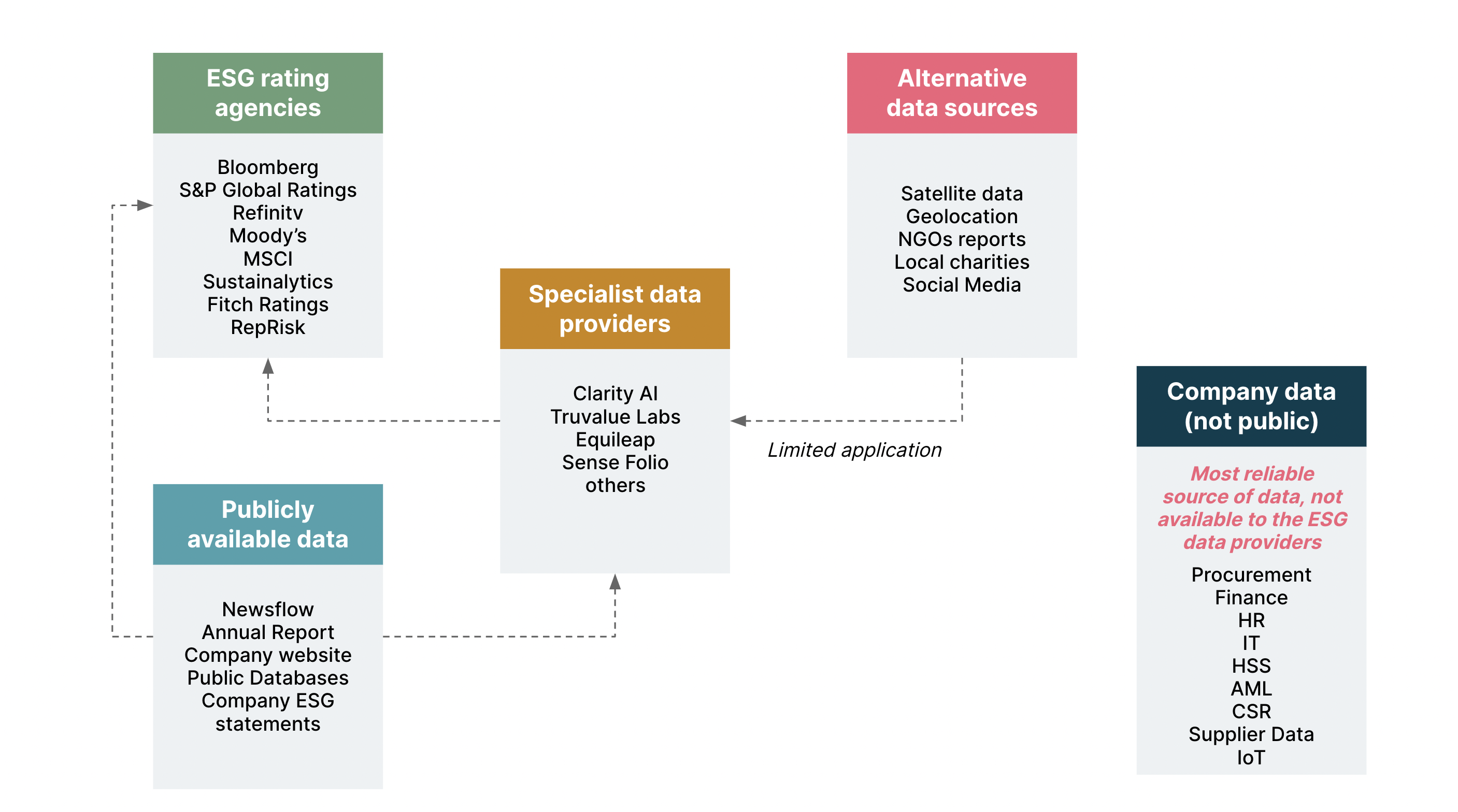
Regulatory compliance for new products
The volume and pace of regulation increased dramatically following the 2008 financial crisis, raising the stakes for non-compliance. Interpreting and implementing regulatory requirements is a costly undertaking for FS firms. It is not unusual for regulatory compliance efforts to take a few years and absorb a significant percentage of ‘run the bank’ budgets.
The challenge is around translating 100s of pages of regulatory reporting standards (RTS) into a reports generation engine. In essence, numerous fields from operational systems and employee-maintained files need to be enriched and mapped to regulatory definitions and reported daily in some cases. All of this is in accordance with differences in jurisdiction, product types, counterparty, etc.
In practice, this means any new products firms develop must comply with all the applicable regulations. The scale of this challenge could be grasped if one considers that in the UK alone financial regulators published on average over 900 regulatory updates per month in 2021 (UK Finance, 2021).
Here, NLP (natural language processing) models can be developed to extract regulatory requirements from RTS (regulatory technical standards), enabling them to be converted into machine-executable rules – the concept of ‘policy as a code’. The model ‘learns’ from historical data and previous regulatory interpretations to dramatically speed up the translation and implementation of new standards. The process can be taken one step further. By adding the rules to a knowledge graph, FS firms can immediately assess the scale and impact of regulatory updates and infer insights into which business areas, processes, and underlying systems will be affected by the new regulations. This brings end-to-end visibility, significantly reduces the risk of misinterpretation, and accelerates the development of new products.
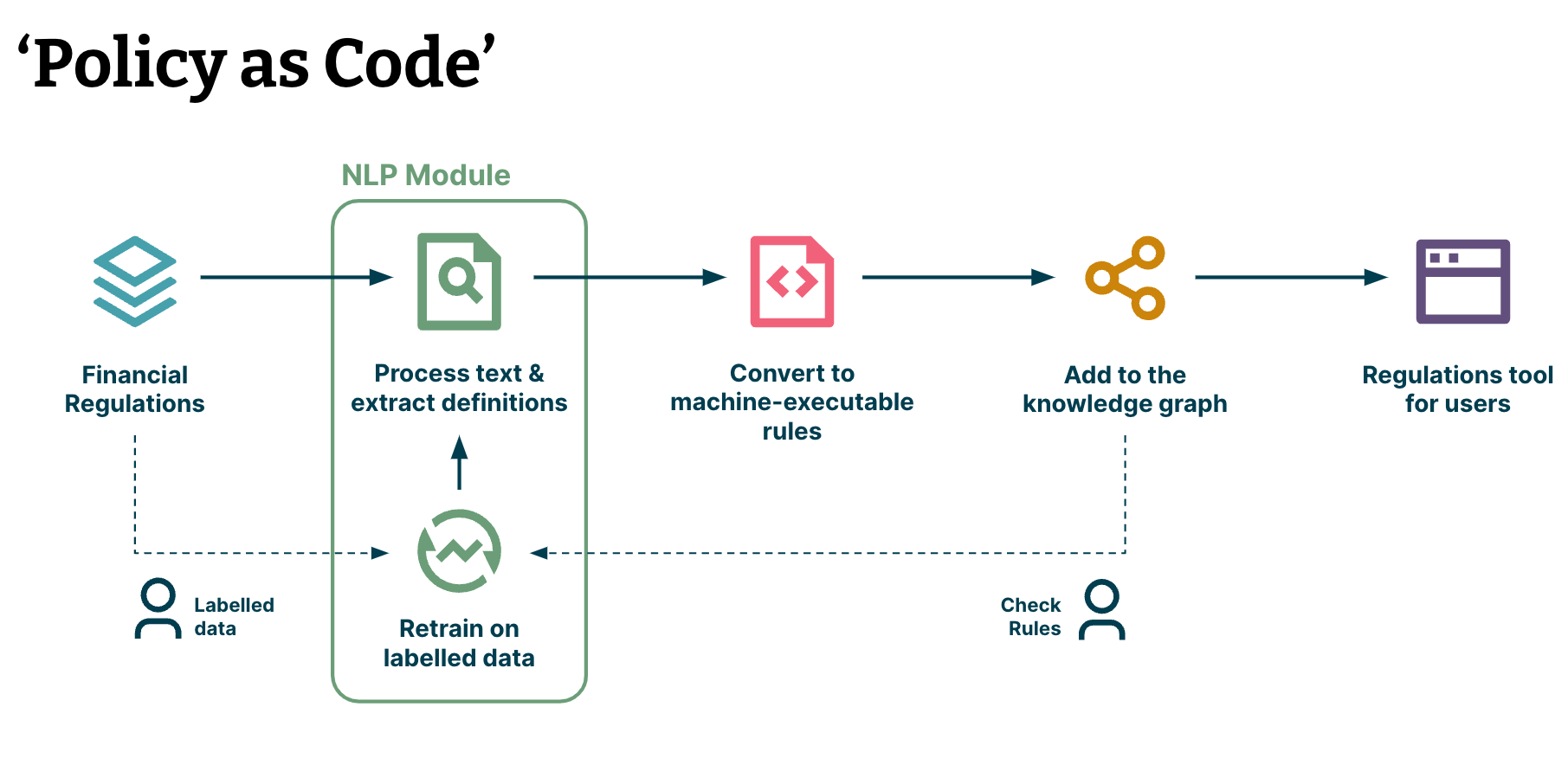
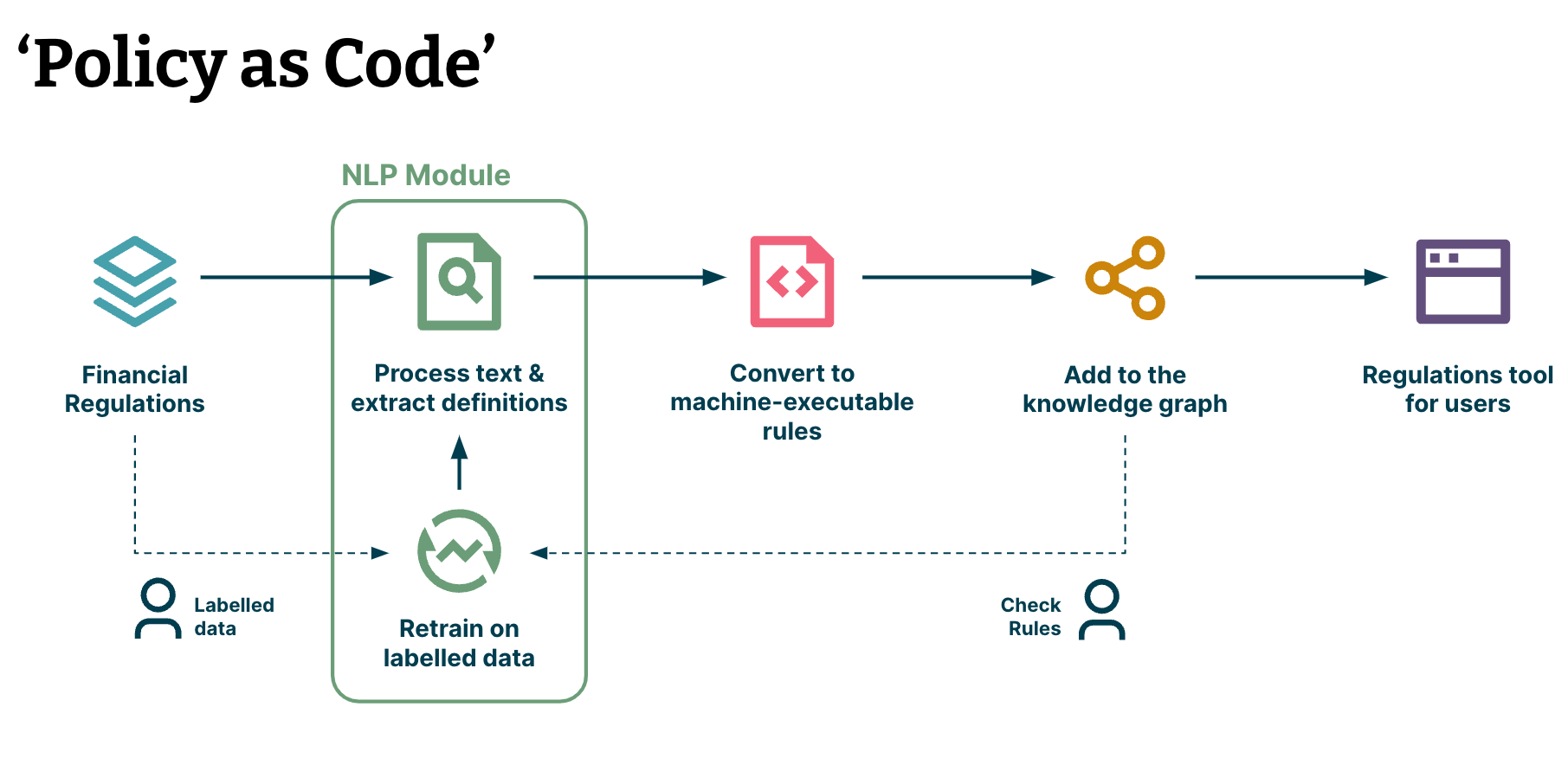
Customized research recommendations
With restrictions imposed by MIFID 2 preventing financial institutions from bundling research as an add-on to core services, it is more critical than ever to provide the right research at the right time to the right recipient. Institutions and investors get a barrage of investment recommendations from various financial institutions - making it even more difficult to stand out.
A research personalization engine could be developed - similar to Netflix’s recommendation algorithm. The engine would learn what research is most important to each individual (e.g. using page views to understand what was viewed by whom for how long), as well as suggesting closely related research (based on portfolio composition and trading behavior for example) to help them explore new sources of insight. Trade and quote history, industry, company events, newsflow timing, and many other characteristics can all be considered as inputs.
Alternative data for investment signals
Financial services firms are always looking for an edge over competitors. In the investing space, there is a big advantage in incorporating alternative, unstructured data that is now widely available (e.g. sentiment from social media, newsflows, consumer spending, lifestyle data, data extracted through web-crawling, and data from alternative dataset providers). To make sense of this continuous flood of data, AI could be applied. Advanced techniques, such as causal inference, could assist investment professionals in identifying how signals from alternative data sources indicate future stock performance, while optimization methods could support them in creating more robust portfolios. Our AI: Augmented approaches utilize such techniques and allow users to handle uncertainty better through portfolio simulation and stress testing based on historical data.
A holistic approach to data in the FS firms
The alternative datasets powering the use cases above will be valuable across many areas in the typical universal bank. There is a paradigm shift taking place right now in enterprise data management and Data Mesh is at its heart. A powerful socio-technical approach, Data Mesh enables firms to leverage their data assets by treating them as products and continuously evolving based on business needs. For instance, alternative data for investment signals could be packaged into a product that can also serve the ESG solution outlined earlier and power CRM initiatives that rely on comprehensive customer profiles. Insights from models in production could be fed back into the mesh to be used as inputs for other models and help evolve the data products.
Financial institutions are starting to realize the value of data mesh. Saxo Bank recently engaged Thoughtworks, recognizing our position as a pioneer of modern data practices. We helped the bank implement a data governance platform that now allows teams to discover and use diverse data assets from across the bank, democratizing insight and supercharging innovation.
Pursuing AI opportunities in the enterprise
We recommend the following practical steps to drive AI value realization:
- Discover opportunities: Characterize and quantify the business opportunities, but don’t solve them right away. This phase should be guided by workshops and interviews with business stakeholders to identify their needs and how AI can support them. An initial risk assessment should be conducted for each opportunity, along with data and tech maturity assessments to understand feasibility.
- Define priorities: Define a set of clear priorities that are shared across all stakeholders, and aligned to desired ML capabilities. We recommend a Lean Value Tree (LVT) to ensure the prioritization of AI initiatives is clearly aligned with strategic goals (per the figure below). LVT forms part of EDGE, an operating model for the digital age.
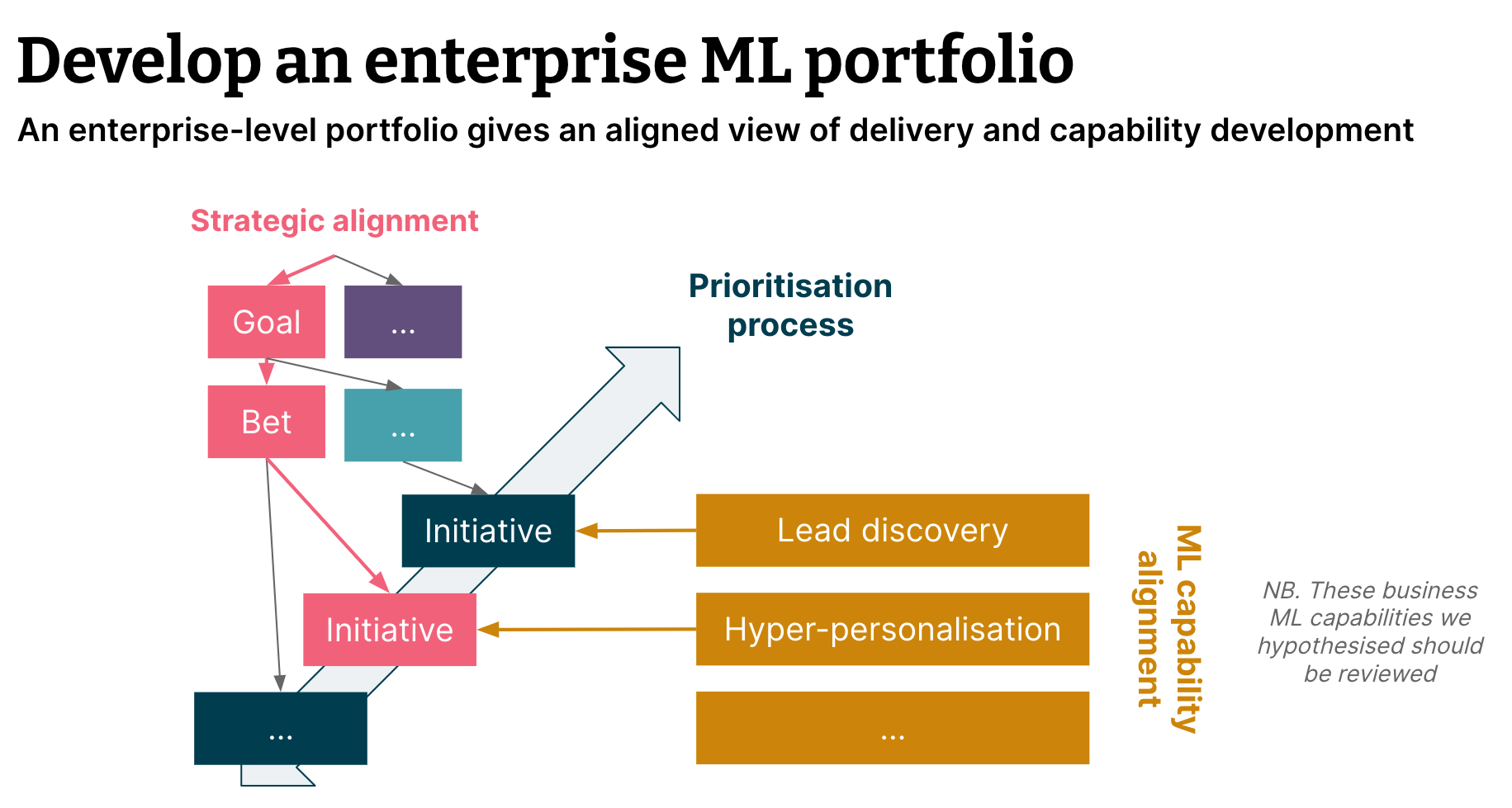
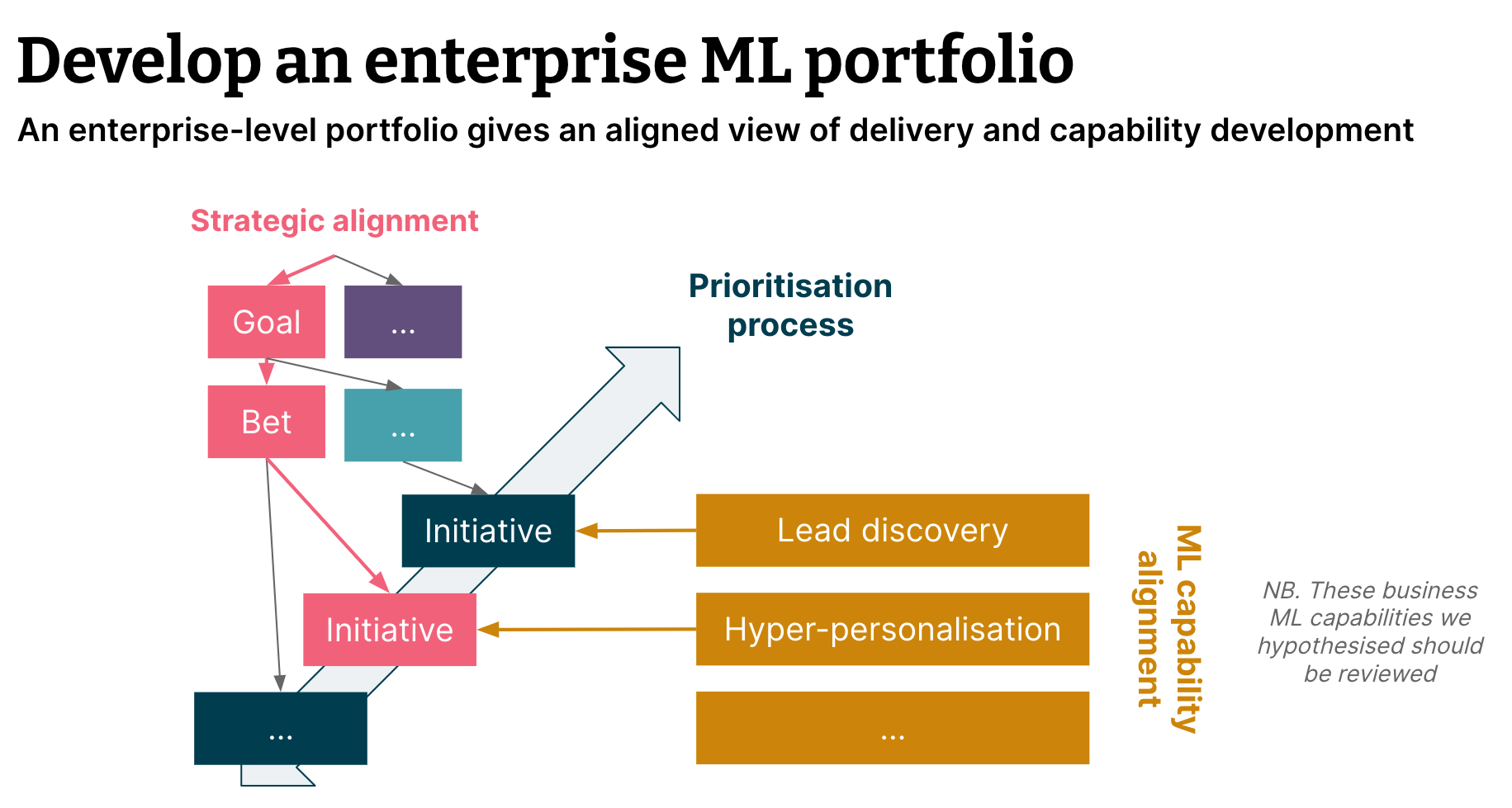
3. Deliver value: Adopt an end-to-end approach by delivering value to the stakeholders iteratively, and measuring outcomes early and often. Use two delivery tracks to manage uncertainty and realize value rapidly (per the figure below):
a) Experiment - run experiments to determine the feasibility and performance of solutions
b) Develop - continuously build and release successful experiments as features, incorporating iterative feedback
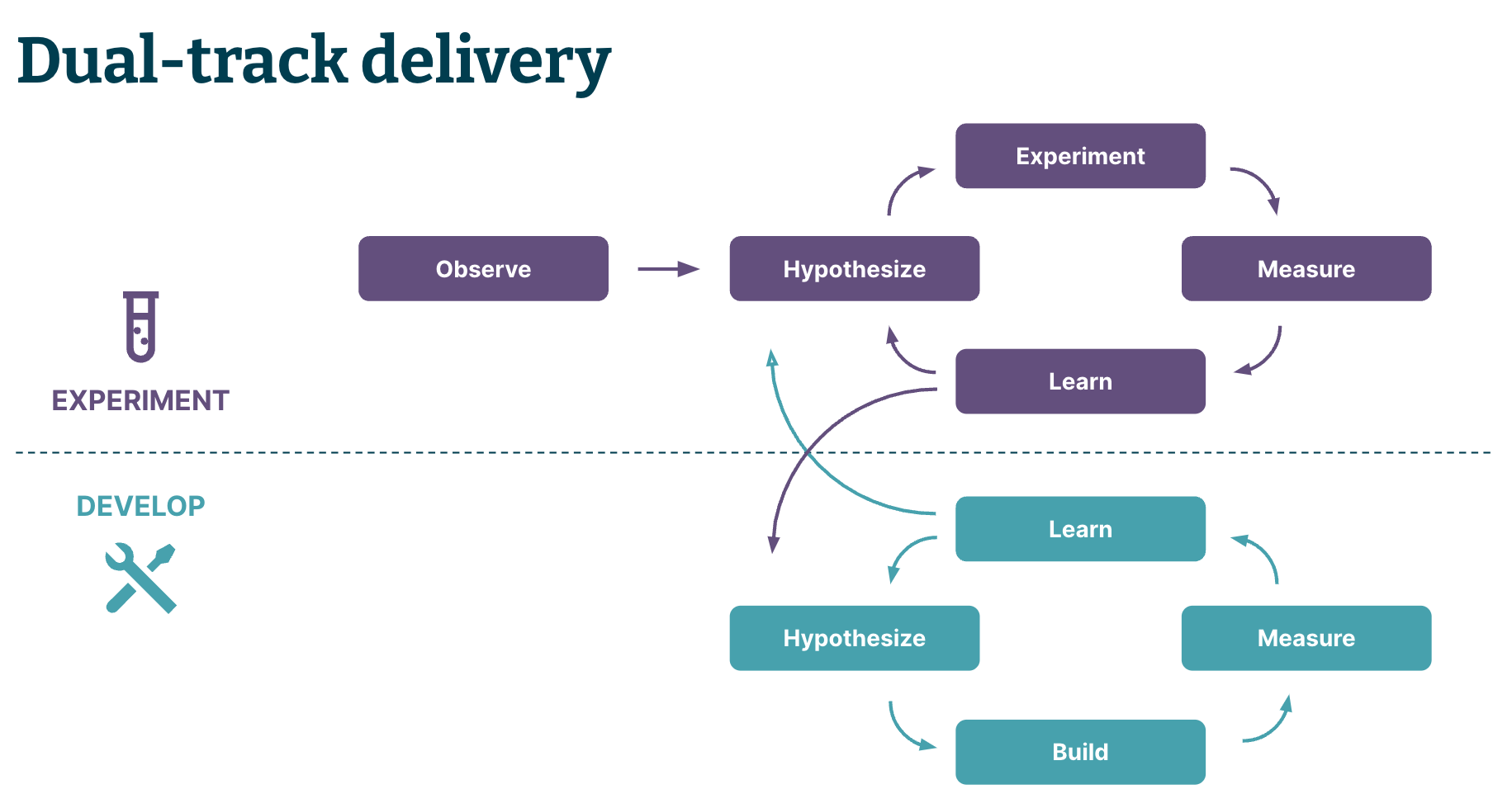
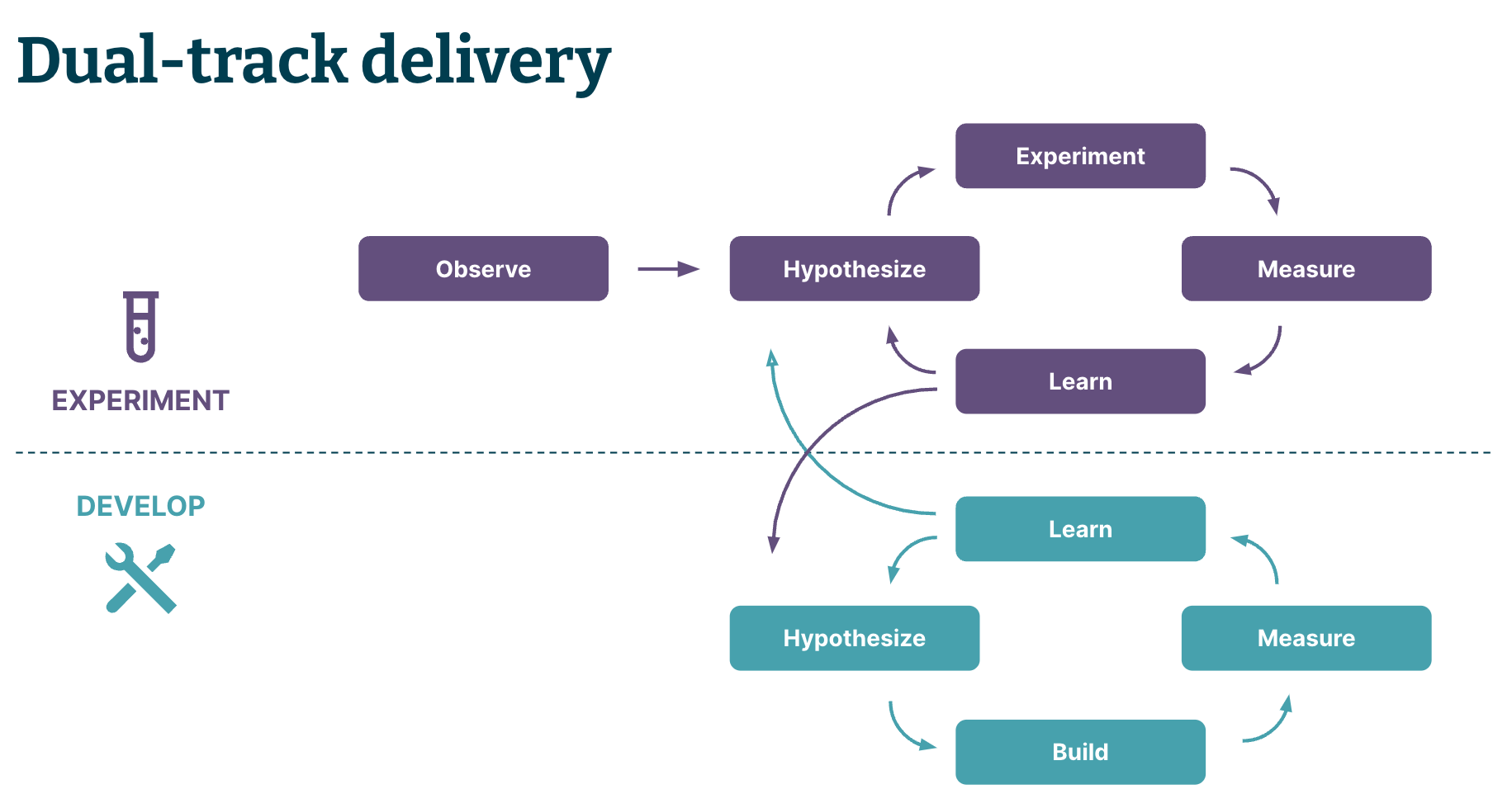
Following these steps will help FS firms capitalize on the opportunities we’ve explored in this article. However, choosing a trusted digital partner can unlock AI-driven value realization at scale. At Thoughtworks, we pride ourselves on being that partner; seamlessly blending our international FS expertise with our experience in delivering value through AI at an enterprise scale.