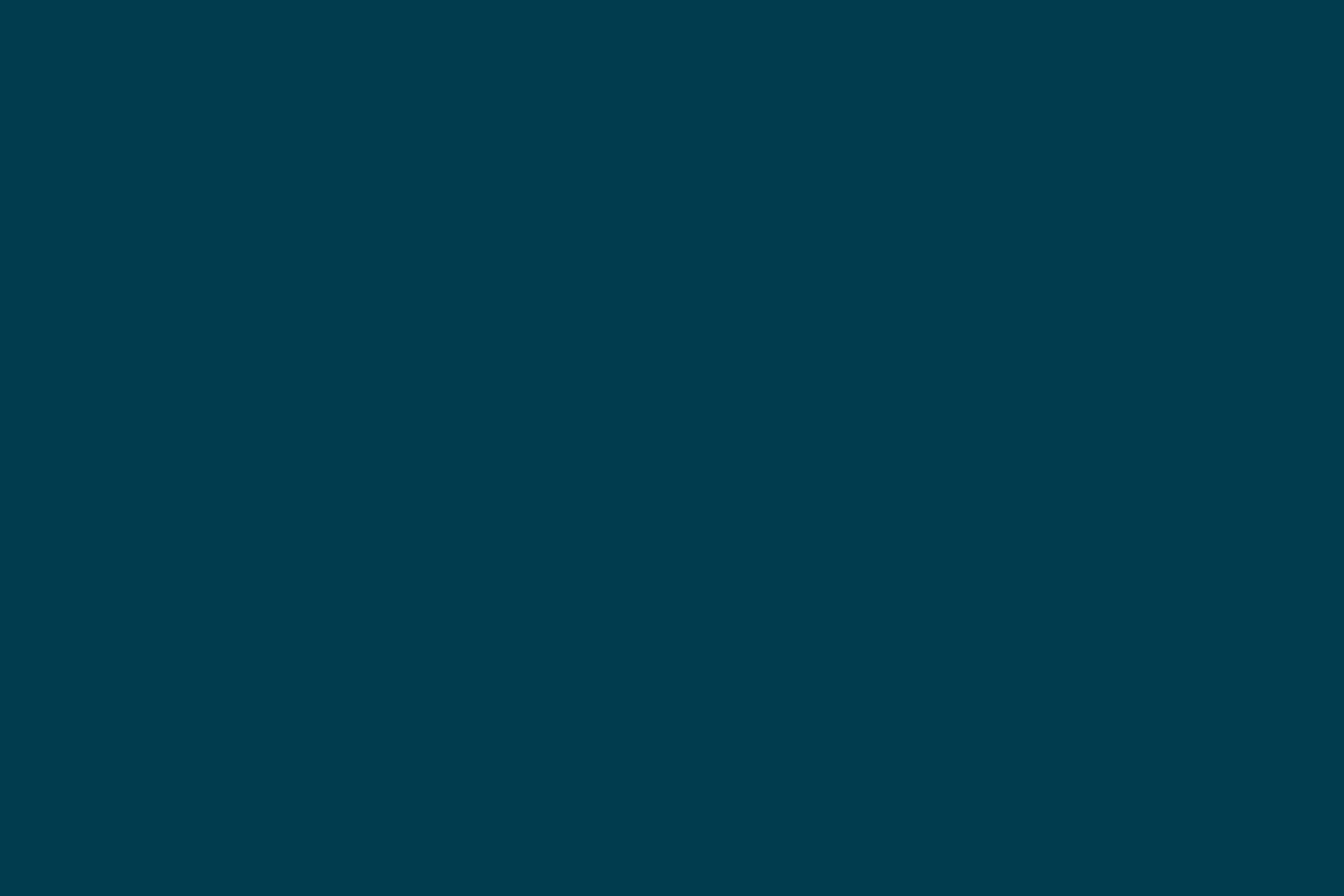
[Book Review] Actionable Agile Metrics for Predictability
Daniel Vacanti’s new book, Actionable Agile Metrics for Predictability, is a welcome addition to the growing canon of thoughtful, experience-based writing on how to improve service delivery. It joins David Anderson’s (Kanban: Successful Evolutionary Change for Your Technology Business) and Mike Burrows’s (Kanban from the Inside) books in my list of must-reads on the Kanban method as an alternative path to agility, complementing those works with deeper insight into how to use metrics to improve flow.
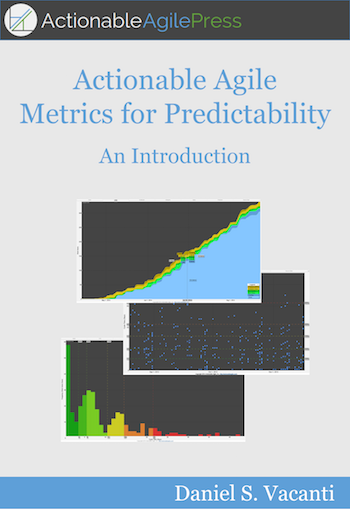
The book is a comprehensive action guide for knowledge-work organizations and teams looking to become more predictable. It focuses on what I often refer to as “second-generation” agile metrics -- cycle time, throughput and work-in-progress -- and shows how to use them effectively and make more informed decisions with them.
Daniel’s message about orienting metrics to promote predictable delivery and flow — which he defines as “the movement and delivery of customer value through a process” — is primarily grounded in his experience helping Siemens HS. He includes the case study (which has been published previously and is valuable reading in itself) at the end of the book and so keeps the rest of the book free from too many customer references, even while drawing on the pragmatic experience.
As someone who for several years has been helping teams and organizations improve using the metrics Daniel talks about, I learned a tremendous amount. One of the reasons is that Daniel is particularly keen to clarify language because it helps us carefully communicate these ideas to teams and management, some of whom may be using these metrics in suboptimal ways or, worse, perverting them so as to give them a bad name and undermine their value. Some examples: the nuanced difference between control charts and scatterplots and clear definitions on Little’s Law (and violations thereof), especially as related to projections and cumulative flow diagrams. I certainly gained a lot of new ideas, and Daniel’s explanations are so thorough that I suspect even novice coaches, managers, team leaders and team members won’t be overwhelmed. Daniel debunks several myths, which I appreciate, such as “work items need to be of the same size” and “kanban doesn’t have commitments.” He does so with clearly reasoned points that make them easy to convey to others. Finally, the book has a very helpful and clarifying discussion on classes of service, namely the difference between using CoS to commit to work (useful) and using it to prioritize committed work (hazardous for predictability).
As for weaknesses, I felt that the chapter on the Monte Carlo method lacked the same kind of depth as the other chapters. I came away wishing that Daniel had included some diagrams showing projections using percentiles from scatterplot data. But those are minor plaints for a book that constantly had me jotting notes in my “things to try” list.
Overall, I loved how Daniel pulled together (no pun intended), for the purpose of flow, several metrics and tools that have often been independently implemented and used and whose purpose— in my experience — was not completely understood. The book unifies these and helps the reader see the bigger picture of why to use them in a way I had not seen before. The book also provides a definitive and deep treatment of cumulative flow diagrams -- and how they provide a single view of the flow metrics of throughput, WIP and cycle time; the six properties of a CFD are both challenging and enlightening.
I’ve already used it many times in trainings and in coaching to give teams a firmer grasp of how to move from traditional agile metrics like velocity to richer, more actionable metrics that point organizations and teams toward improvements and data-driven decision making to reduce risk, improve predictability and deliver better.
Disclaimer: The statements and opinions expressed in this article are those of the author(s) and do not necessarily reflect the positions of Thoughtworks.