Our community of Data Experts think disruptively to provide pragmatic solutions for our clients' most complex challenges. We are curious minds who come together in collaborative and inclusive teams to push boundaries to make a positive impact in the world by harnessing the power of Data and Artificial Intelligence (AI).
We are looking for change makers, opportunity creators, status-quo shakers. If that’s you, what are you waiting for?
Making an impact across data archetypes

Data engineers
are responsible for bringing our clients scalable and robust solutions related to the processes of creating pipelines, platforms, organization, governance and data quality. They have experience in cloud, on-premises technologies and migrations.

Data architects
are responsible for designing reference architectures, covering key aspects of data management, governance, domains, modeling, integration, security, compliance and more. They are responsible for the discovery, roadmap, feasibility study and recommendation of frameworks, practices and tools in the data world to better meet business objectives.

Data scientists
are responsible for identifying business opportunities and how to respond to them through the applied use of data and thus maximizing client results. They play a strategic role both from a technical and business point of view, proposing the use of advanced machine learning techniques along with algorithms and success metrics that will serve in the future to evaluate the results of production models.

ML engineers
are responsible for providing the technical components capable of enabling CD4ML principles such as experiment versioning tools, data repositories, automation mats and integration layers with production environments. They work closely with data scientists, evaluating aspects of scalability and performance for proposed data models.

Data analysts
are responsible for conducting complex analysis, proposing business indicators and generating analytic solutions to support clients in generating business value. They have experience in transforming data into insights through understanding the business and creating automated dashboards for demonstrating results and making decisions.
Life at Thoughtworks as a data professional
Learn more about life at Thoughtworks as a data professional from Clara Brünn, Data Scientist, Ina Iovitoiu, Data Scientist, Inna Zykova, Data Engineer and Javier Molina Sanchez, Lead Data Engineer. From choosing Thoughtworks to what it’s like to work here as a data professional to details of their project work and advice to those thinking of bringing their data skills to Thoughtworks – this is great insight into being a data professional at Thoughtworks.
What kind of Data and AI projects are you working on at Thoughtworks?
What advice do you have for someone exploring Data and AI at Thoughtworks?
Why did you choose Thoughtworks to grow your Data and AI career?
What is it like to work in Data and AI at Thoughtworks?
People you might work with
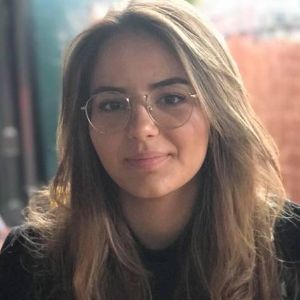
Meissane Chami
Lead machine learning engineer, UK
Meissane is a Lead Machine Learning (ML) Engineer for Thoughtworks UK. She started her career as a platform developer and then transitioned to her current role where she applies agile software delivery practices to AI and ML. Her primary expertise is in delivering effective AI (data science, MLOps, CD4ML) from proof of concept to production. Meissane's academic background is also in ML and statistics with a focus on unsupervised learning and generative models. She is currently interested in practical governance practices within ML teams. You can meet her in our Londo office.
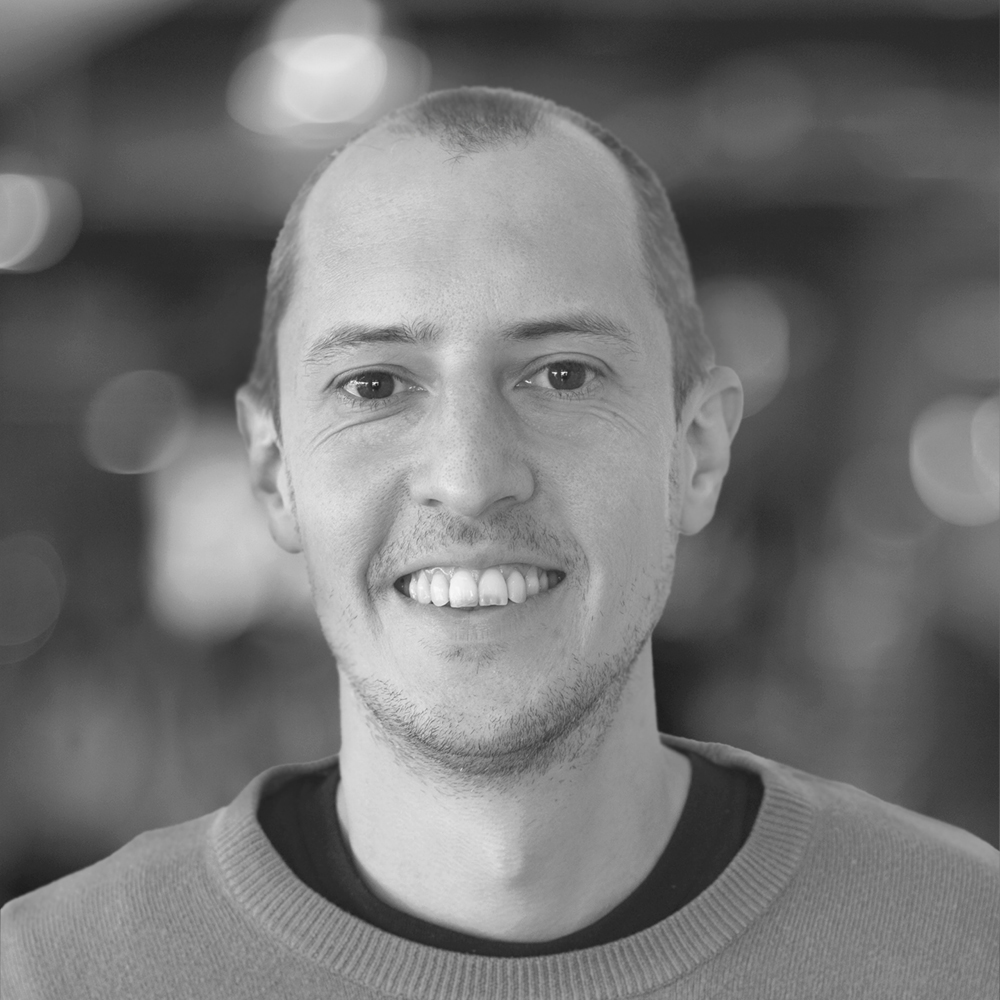
Ryan Dawson
Principal data consultant, UK
A technologist passionate about data, Ryan works with clients on large-scale data and AI initiatives. Ryan helps organizations get more value from data. His work includes strategies to productionize machine learning, organizing the way data is captured and shared, selecting the right data technologies and optimal team structures.
He has over 15 years of experience and is author of the Thoughtworks Guide to Evaluating MLOps Platforms, as well as many widely read articles about MLOps, software design and delivery.
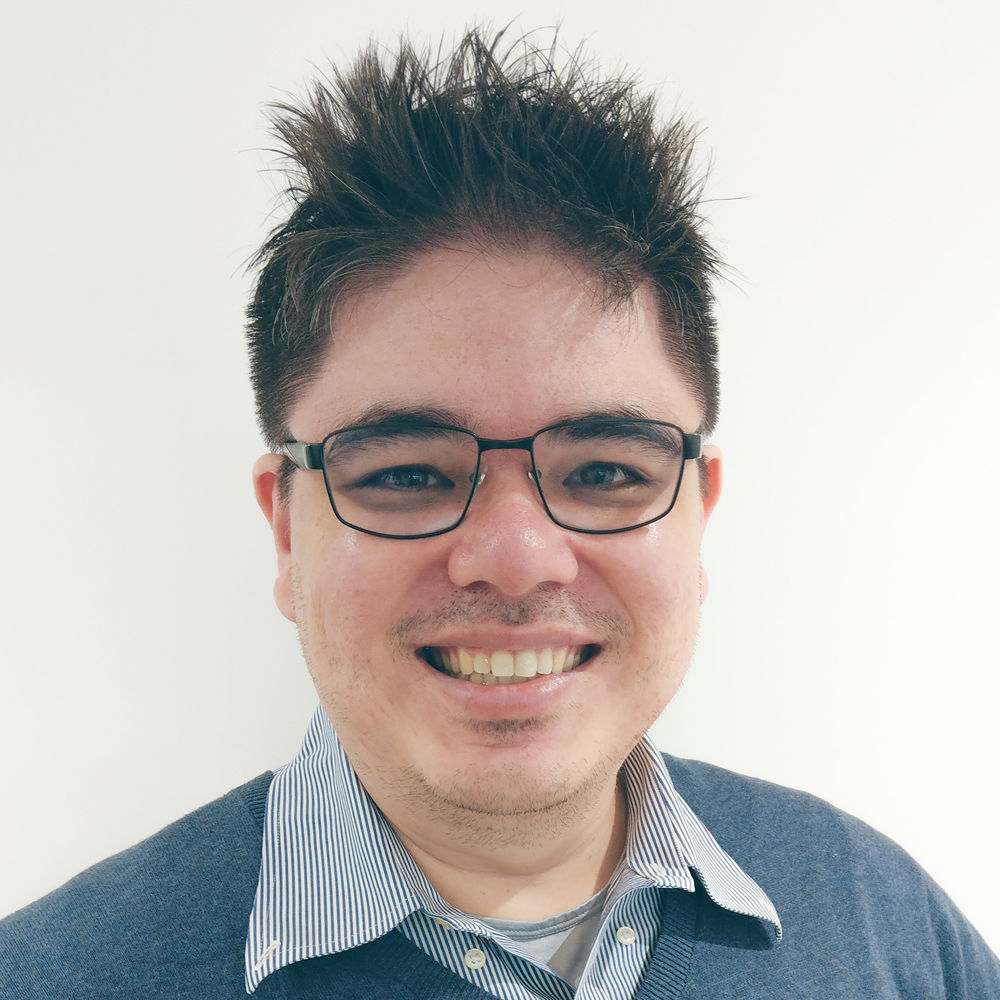
Danilo Sato
Head of Data & AI Services, UK
As the Data service line lead for Thoughtworks UK and Europe, Danilo is responsible for building high-performing teams and unique propositions that help our clients win with Data & AI. That covers the full spectrum of our services, from data strategy and governance to building the Data & AI products and platforms that bring the strategy to life. Danilo is also responsible for driving Thoughtworks' unique approaches, such as Data Mesh and Continuous Delivery for Machine Learning (CD4ML).
How we help our clients
Data strategy & governance
We help our clients to get greater value from data by creating a clear roadmap that ensures trustworthiness, security and compliance, while making it effortlessly accessible and user-friendly. This way they can take control of their data landscape and empower data consumers through clear governance policies and alignment with business objectives.

Data platform modernization & Data Mesh
We support our clients to put their data into action by enabling business teams to create and consume reliable self-service data products that scale easily and support diverse analytics.
With our help they apply world-class data architecture models such as Data Mesh to bring a product mindset, modern software engineering methods, and people-centric changes to accelerate data delivery.
AI & analytics
Our data experts consult our clients in elevating their potential for extraordinary results by automating routine work and augmenting your team’s unique capabilities with people-centric, ethical artificial intelligence (AI) and analytics.
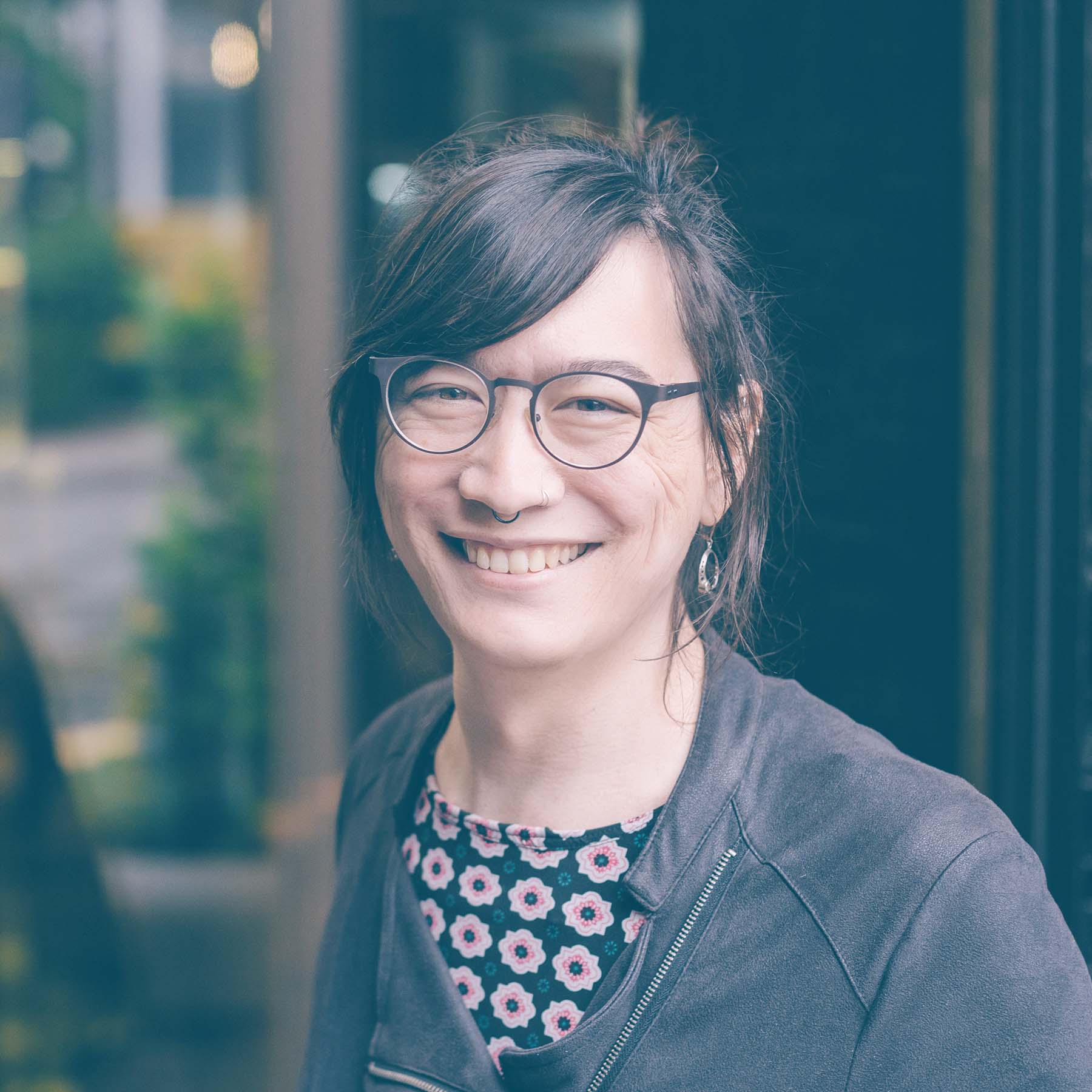
Data should challenge our assumptions and instincts from time to time. And if it doesn't there is something wrong.
Data should challenge our assumptions and instincts from time to time. And if it doesn't there is something wrong.
Building Data Mesh on Databricks and Azure for a large manufacturing company
Rieke Heinze and Dominika Makuch
It is hard to find detailed technical recommendations for implementing Data Mesh. Thoughtworks is currently supporting a large European manufacturing company on their Data Mesh journey using a concrete technology stack: Databricks on Azure. In this talk you will learn about concrete examples of libraries and services we used to implement Data Mesh principles. We will also share lessons and obstacles we faced, as well as things we found missing.
Unlocking value from Data and AI at scale
Danilo Sato and Kiran Prakash
In this webinar, Thoughtworks' experts discuss the benefits of using the Data Mesh approach and the disadvantages of a centralized data management system, drawing on real life data projects.